ACF-TransUNet: Attention-based Coarse-Fine Transformer U-Net for Automatic Liver Tumor Segmentation in CT Images.
2023 4th International Conference on Big Data & Artificial Intelligence & Software Engineering (ICBASE)(2023)
摘要
Diagnosis of liver tumor via Computed Tomography (CT) imaging is crucial. However, manual delineation is time-consuming and presents inherent subjectivity. While deep learning models like U-Net have significantly enhanced image segmentation, they sometimes falter in capturing the nuanced variations in liver tumor morphology. Similarly, models combining Convolutional Neural Networks (CNNs) and the Transformer architecture have shown promise but still face challenges in balancing global and local feature extraction effectively. To address this problem, we propose a novel Attention-based Coarse-Fine TransUNet (ACF-TransUNet) architecture explicitly designed for liver tumor segmentation. ACF-TransUNet synergizes the strengths of CNNs and Transformers, emphasizing globally relevant spatial features while retaining detailed local information. Our architecture incorporates a channel attention module to weigh the importance of each feature channel, ensuring adaptive feature recalibration. Integrating a novel Coarse-Fine (CF) module enables the model to simultaneously produce coarse-grained and fine-grained feature maps, whose weighted fusion ensures a comprehensive and precise representation. Employing a two-stage strategy, we initially localize the liver region using the U-Net, and then segment the tumors using ACF-TransUNet. Our method achieves competitive performance on the LiTS dataset with a Dice score of 0.7752, demonstrating its potential to be a valuable tool in clinical liver tumor diagnosis.
更多查看译文
关键词
Liver tumor,CT,U-Net,TransUNet,channel attention,Coarse-Fine
AI 理解论文
溯源树
样例
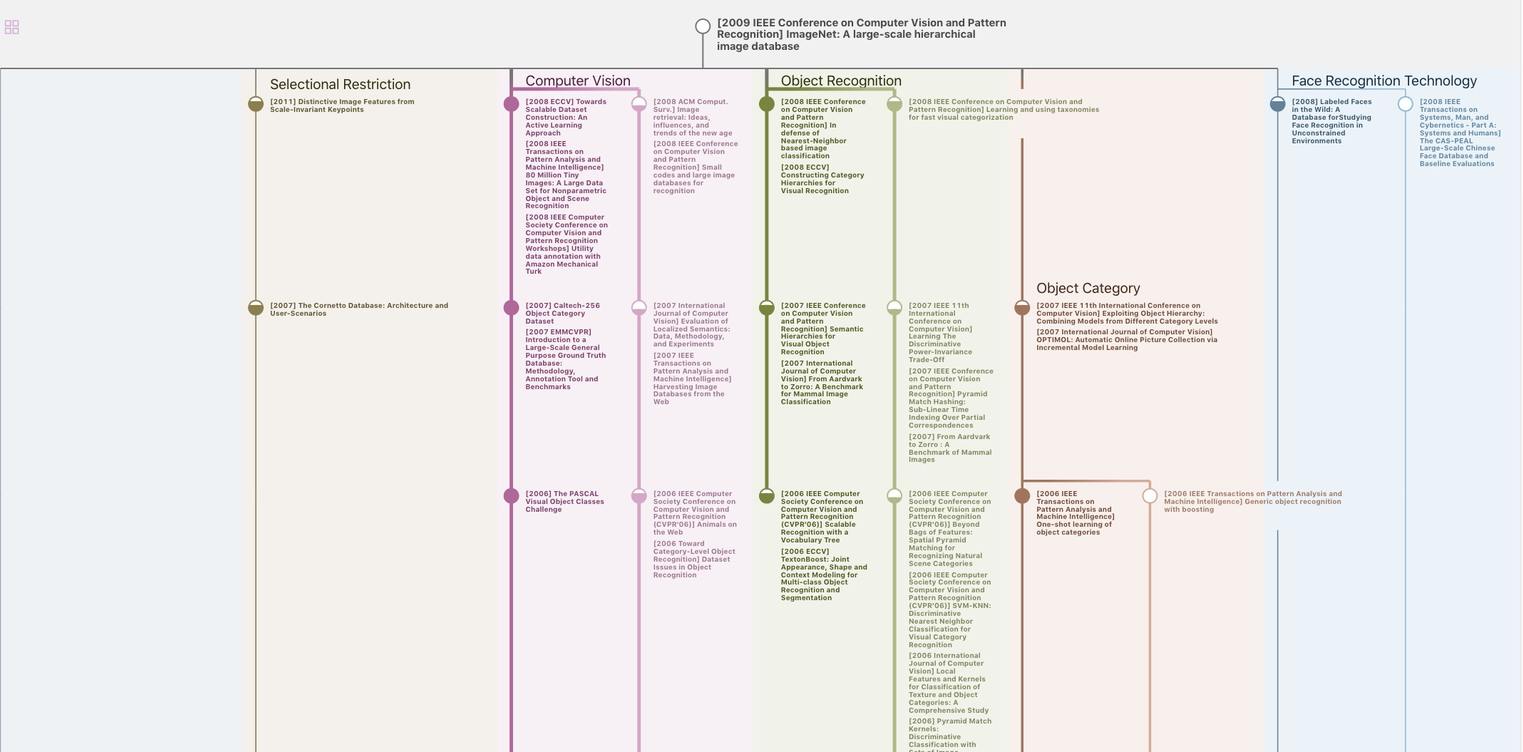
生成溯源树,研究论文发展脉络
Chat Paper
正在生成论文摘要