Deep CNN- RNN with Self- Attention Model for Electric IoT Traffic Classification
ICBASE(2023)
摘要
Network traffic classification is crucial for network monitoring and analysis, including legitimate interception, quality of service assurance, application bottleneck prevention, and malicious behavior identification. Currently, the three main approaches for traffic classification are based on ports, payloads, and statistical features, i.e. machine learning. In the Electric Power Internet of Things domain, Modbus and MQTT are essential communication protocols. Modbus is widely used in industrial automation, while MQTT is designed for IoT ap- plications and offers faster response time, greater throughput, lower battery and bandwidth usage, and the ability to operate over low-speed network connections. This study focuses on network traffic classification in the context of power IoT. By simulating the IoT environment and capturing packets using Modbus and MQTT protocol communication, traffic data is processed into a pseudo-image format. A network architecture based on CNN-RNN with a self-attention mechanism is proposed to classify network traffic data and achieve higher classification accuracy. The Self-Attention module enables adaptive focus on different regions and feature capturing. Extensive experiments with multiple models and datasets demonstrate that the CNN- RNN model can achieve an accuracy of approximately 95%. Furthermore, the Self-Attention mechanism improves the CNN- RNN model's convergence speed, enhancing its training speed.
更多查看译文
关键词
component,electric power internet of things,neural networks,network traffic classification
AI 理解论文
溯源树
样例
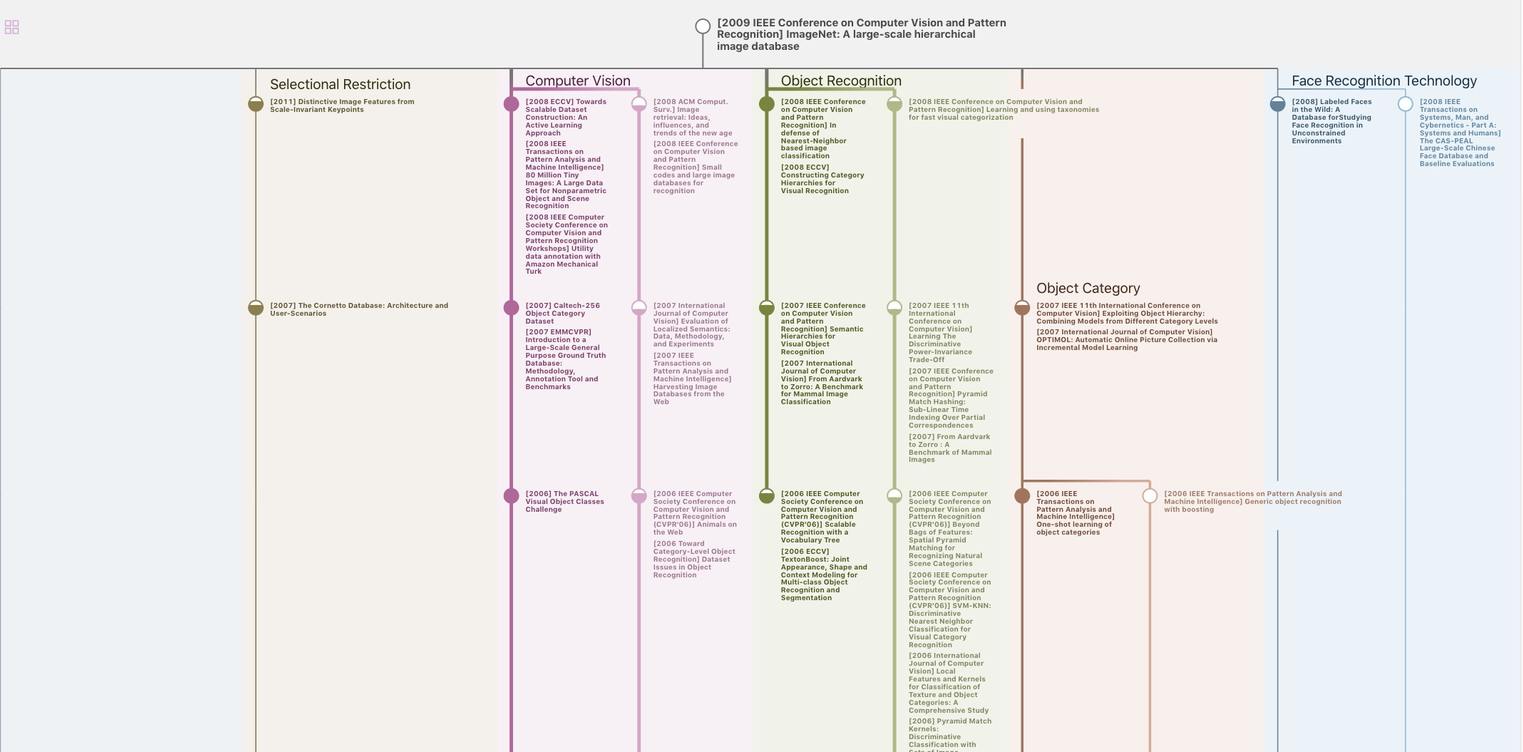
生成溯源树,研究论文发展脉络
Chat Paper
正在生成论文摘要