Investigating the impact of borehole field data's input parameters on the forecasting accuracy of multivariate hybrid deep learning models for heating and cooling
ENERGY AND BUILDINGS(2023)
摘要
Heat pump systems coupled with Borehole heat exchanger (BHEx) are crucial for the efficient and cost-effective heating and cooling of buildings. Accurate performance forecasting of BHEx can help to reduce energy consumption, optimize system design, improve system reliability and ultimately contribute to the sustainability of the built environment. The performance of deep neural networks, including hybrid deep learning algorithms, is dependent on the number and true identification of input parameters used to train the model. While research on the accuracy and selection of individual deep neural networks is well-investigated, the impact of selected input parameters on hybrid algorithm selection and its subsequent influence on multi-step forecasting performance has not been quantified specifically for geothermal heating/cooling systems. The focus of the current work is to investigate and quantify this impact on multivariate hybrid deep learning algorithms for forecasting borehole heat exchanger outlet temperature. In the current work the developed hybrid AI models (LSTM-CNN, GRU-CNN, CNN-LSTM, CNN-GRU) have been trained, validated, and tested using the measured borehole-field data with variable number of input parameters (3,4,5 and 6). The validated AI models are used to forecast 24 h ahead performance of borehole heat exchanger to meet the heating and cooling loads. The most essential input parameters for the data-driven AI models are determined via an importance-level analysis by using Explainable AI techniques of Shapley Additive Explanations (SHAP). The findings show that increasing the number of input parameters has the potential to enhance the multi-step forecasting accuracy of the examined deep learning models. However, it is crucial to note that there is a point where including additional input parameters may not lead to further improvements. Therefore, it is essential to identify the optimal number of input parameters that can maximize the accuracy of the hybrid deep learning models. Moreover, the analysis highlights that depending on the architecture of specific model layers, it is possible to eliminate redundant weather sensors associated with time variables without compromising the accuracy of the hybrid deep learning models.
更多查看译文
关键词
Borehole coupled heat pump,Heating and cooling,Ground source heat pump,Prediction model,Hybrid forecasting models,Data driven modeling
AI 理解论文
溯源树
样例
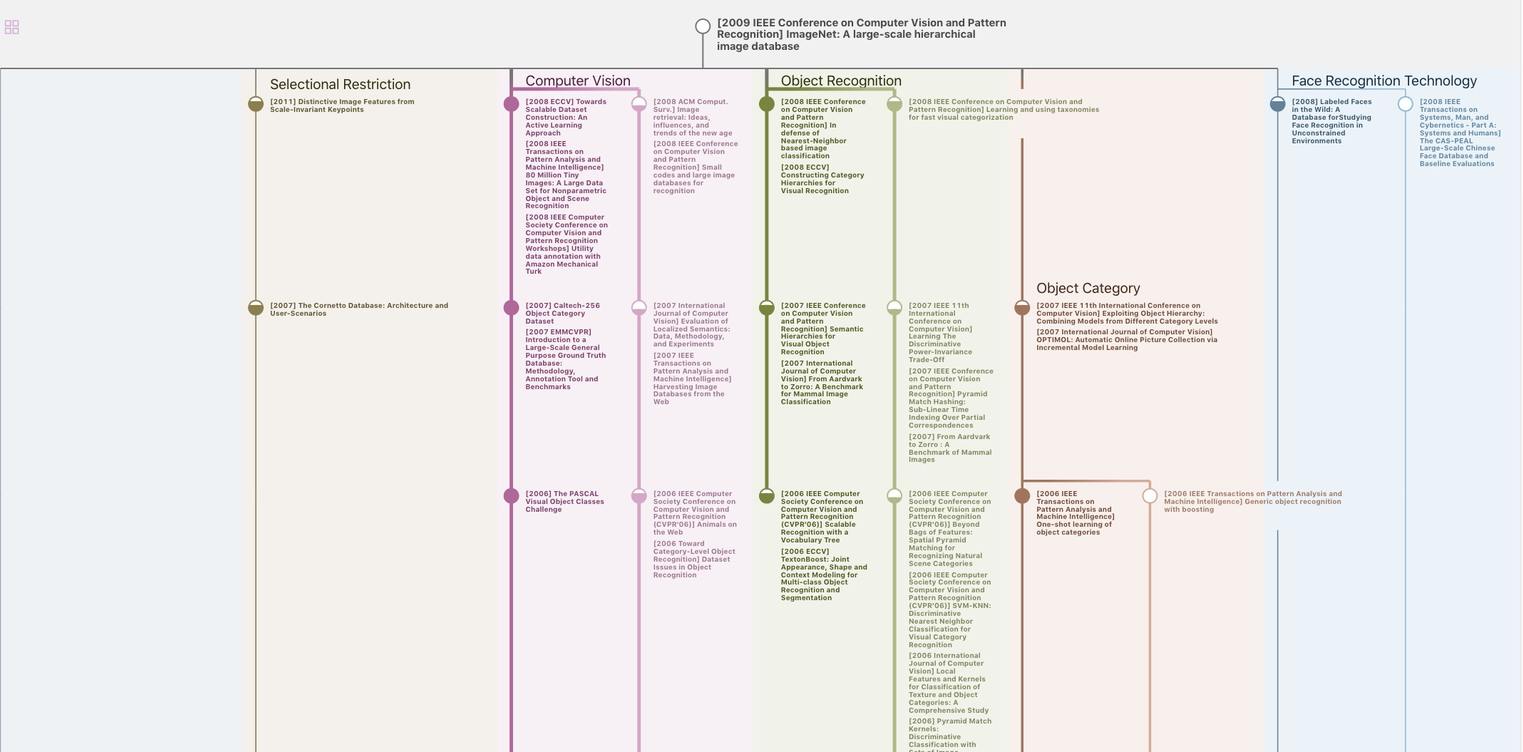
生成溯源树,研究论文发展脉络
Chat Paper
正在生成论文摘要