Adaptive batch mode active learning with deep similarity
EGYPTIAN INFORMATICS JOURNAL(2023)
摘要
Active learning is usually used in scenarios where few labels are available and manual labeling is expensive. To improve model performance, it is necessary to find the most valuable instance among all instances and label it to maximize the benefits of labeling. In practical scenarios, it is often more efficient to query a group of instances instead of a individual instance during each iteration. To achieve this goal, we need to explore the similarities between instances to ensure the informativeness and diversity. Many ad-hoc algorithms are proposed for batch mode active learning, and there are generally two major issues. One is that similarity measurement among in-stances often only relies on the expression of features but it is not well integrated with the classification algo-rithm model. This will cut down the precise measurement of diversity. The other is that in order to explore the decision boundary, these algorithms often choose the instance near the boundary. It is difficult to get the true boundary when there are few labeled instances. As a large number of instances continue to be labeled, infor-mation between instances is less used, and the performance will be greatly improved if it is properly used. In our work, we propose an adaptive algorithm based on deep neural networks to solve the two problems mentioned above. During the training phase, we established a paired network to improve the accuracy of the classification model, and the network can project the instance to a new feature space for more accurate similarity measure-ment. When batch labeling instances, we use the adaptive algorithm to select the instance by balancing the maximum uncertainty (exploration) and diversity (exploitation). Our algorithm has been validated for heart failure prediction tasks in real-world EHR datasets. Due to the no public of EHR data, we also conducted vali-dation on two other classic classification tasks. Our algorithm is superior to the baseline method in both accuracy and convergence rate.
更多查看译文
关键词
Active learning,Adaptive batch mode active learning,Classification model,Deep neural network,Deep learning
AI 理解论文
溯源树
样例
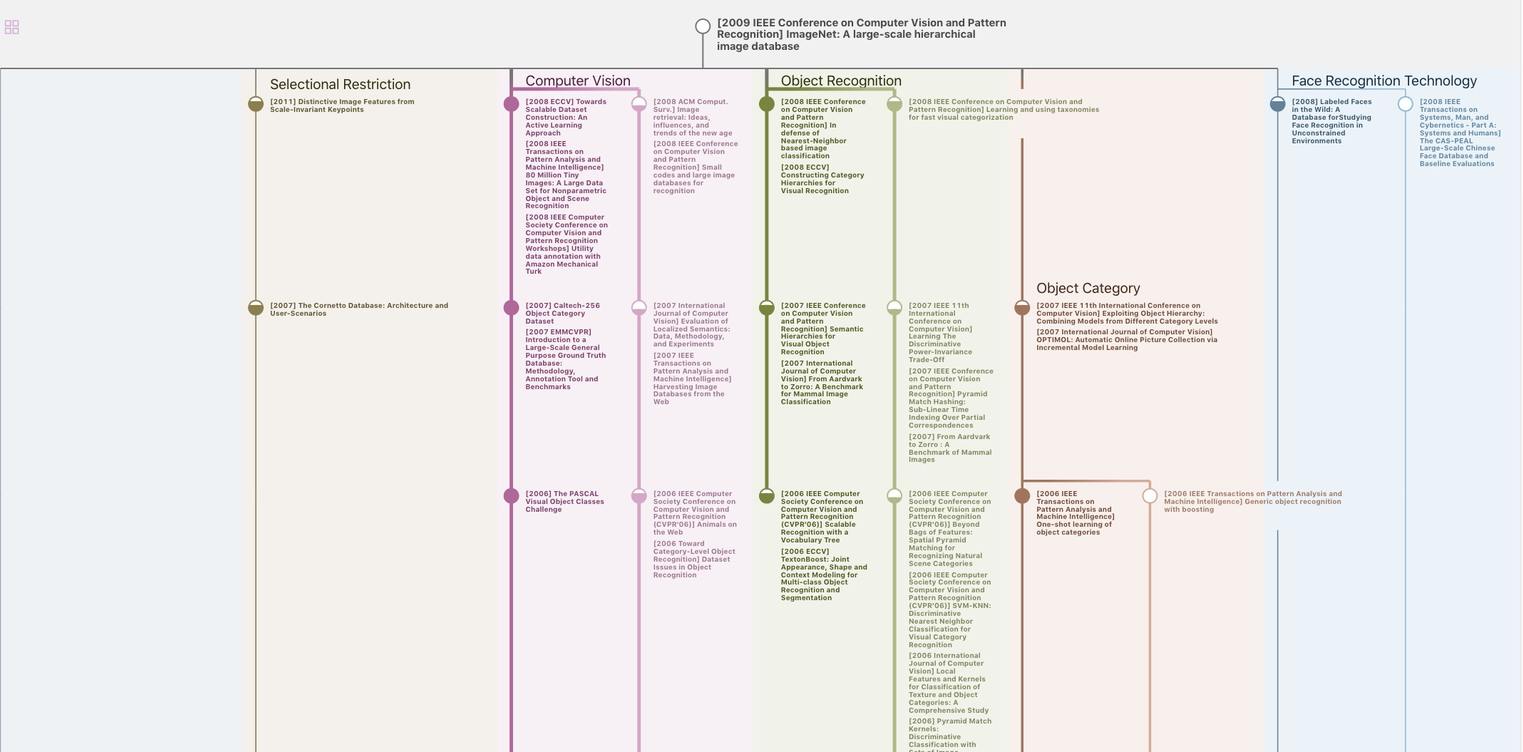
生成溯源树,研究论文发展脉络
Chat Paper
正在生成论文摘要