A Generalized Supervised Contrastive Learning Framework for Integrative Multi-omics Prediction Models
bioRxiv (Cold Spring Harbor Laboratory)(2023)
摘要
Recent technological advances have highlighted the significant impact of the human microbiome and metabolites on physiological conditions. Integrating microbiome and metabolite data has shown promise in predictive capabilities. We developed a new supervised contrastive learning framework, MB-SupCon-cont, that (1) proposes a general contrastive learning framework for continuous outcomes and (2) improves prediction accuracy over models using single omics data. Simulation studies confirmed the improved performance of MB-SupCon-cont, and applied scenarios in type 2 diabetes and high-fat diet studies also showed improved prediction performance. Overall, MB-SupCon-cont is a versatile research tool for multi-omics prediction models.
### Competing Interest Statement
The authors have declared no competing interest.
更多查看译文
关键词
contrastive learning,prediction,multi-omics
AI 理解论文
溯源树
样例
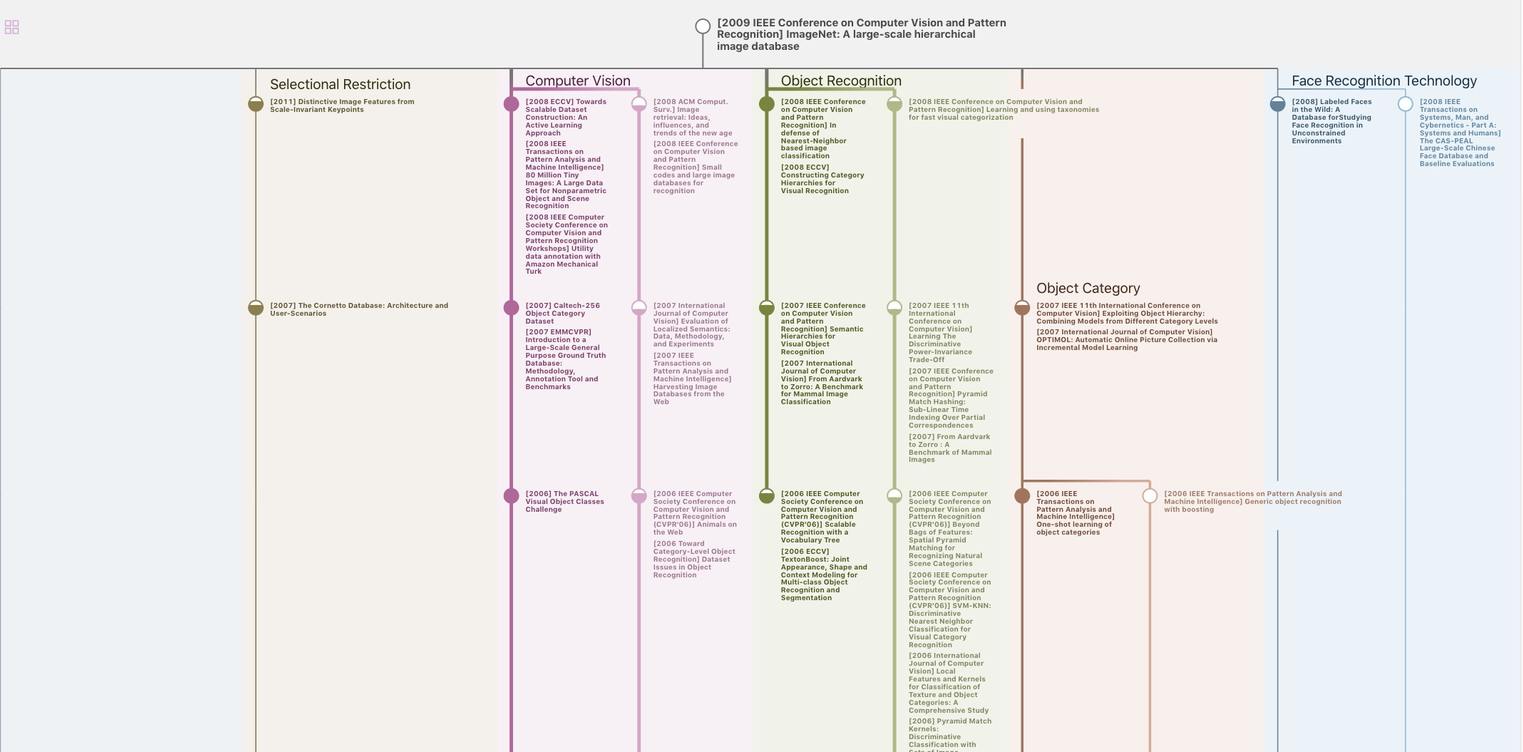
生成溯源树,研究论文发展脉络
Chat Paper
正在生成论文摘要