lociPARSE: a locality-aware invariant point attention model for scoring RNA 3D structures.
bioRxiv : the preprint server for biology(2023)
摘要
A scoring function that can reliably assess the accuracy of a 3D RNA structural model in the absence of experimental structure is not only important for model evaluation and selection but also useful for scoring-guided conformational sampling. However, high-fidelity RNA scoring has proven to be difficult using conventional knowledge-based statistical potentials and currently-available machine learning-based approaches. Here we present lociPARSE, a locality-aware invariant point attention architecture for scoring RNA 3D structures. Unlike existing machine learning methods that estimate superposition-based root mean square deviation (RMSD), lociPARSE estimates Local Distance Difference Test (lDDT) scores capturing the accuracy of each nucleotide and its surrounding local atomic environment in a superposition-free manner, before aggregating information to predict global structural accuracy. Tested on multiple datasets including CASP15, lociPARSE significantly outperforms existing statistical potentials (rsRNASP, cgRNASP, DFIRE-RNA, and RASP) and machine learning methods (ARES and RNA3DCNN) across complementary assessment metrics. lociPARSE is freely available at https://github.com/Bhattacharya-Lab/lociPARSE.
更多查看译文
关键词
rna,attention,3d,locality-aware
AI 理解论文
溯源树
样例
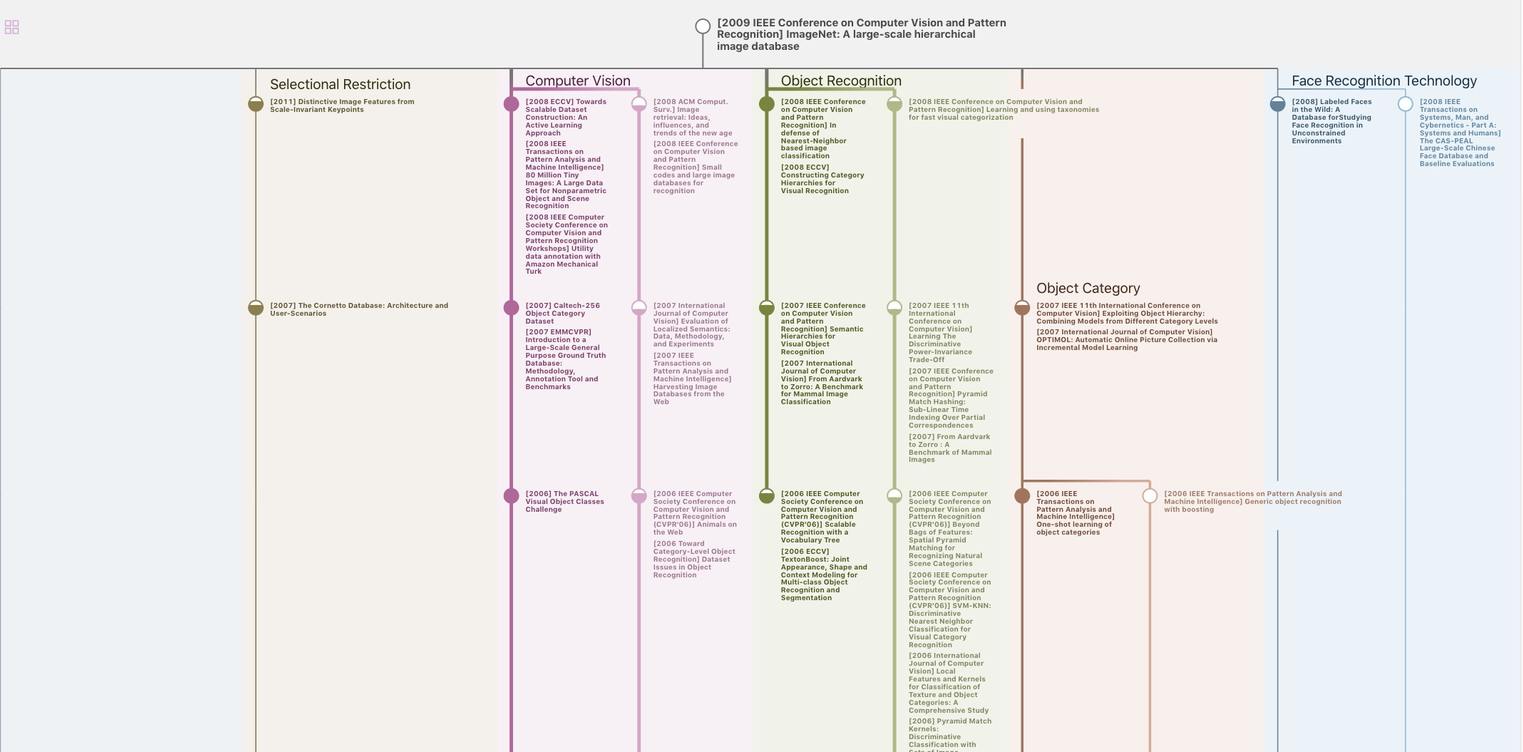
生成溯源树,研究论文发展脉络
Chat Paper
正在生成论文摘要