Three-Dimensional Residual Neural Architecture Search for Ultrasonic Defect Detection
IEEE TRANSACTIONS ON ULTRASONICS FERROELECTRICS AND FREQUENCY CONTROL(2024)
摘要
This study presents a deep-learning (DL) methodology using 3-D convolutional neural networks (CNNs) to detect defects in carbon fiber-reinforced polymer (CFRP) composites through volumetric ultrasonic testing (UT) data. Acquiring large amounts of ultrasonic training data experimentally is expensive and time-consuming. To address this issue, a synthetic data generation method was extended to incorporate volumetric data. By preserving the complete volumetric data, complex preprocessing is reduced, and the model can utilize spatial and temporal information that is lost during imaging. This enables the model to utilize important features that might be overlooked otherwise. The performance of three architectures was compared. The first architecture is prevalent in the literature for the classification of volumetric datasets. The second demonstrated a hand-designed approach to architecture design, with modifications to the first architecture to address the challenges of this specific task. A key modification was the use of cuboidal kernels to account for the large aspect ratios seen in ultrasonic data. The third architecture was discovered through neural architecture search (NAS) from a modified 3-D residual neural network (ResNet) search space. In addition, domain-specific augmentation methods were incorporated during training, resulting in significant improvements in model performance, with a mean accuracy improvement of 22.4% on the discovered architecture. The discovered architecture demonstrated the best performance with a mean accuracy increase of 7.9% over the second-best model. It was able to consistently detect all defects while maintaining a model size smaller than most 2-D ResNets. Each model had an inference time of less than 0.5 s, making them efficient for the interpretation of large amounts of data. [GRAPHICS]
更多查看译文
关键词
Image processing and computer vision,neural architecture search (NAS),nondestructive evaluation (NDE),synthetic data,ultrasonic testing (UT),volumetric
AI 理解论文
溯源树
样例
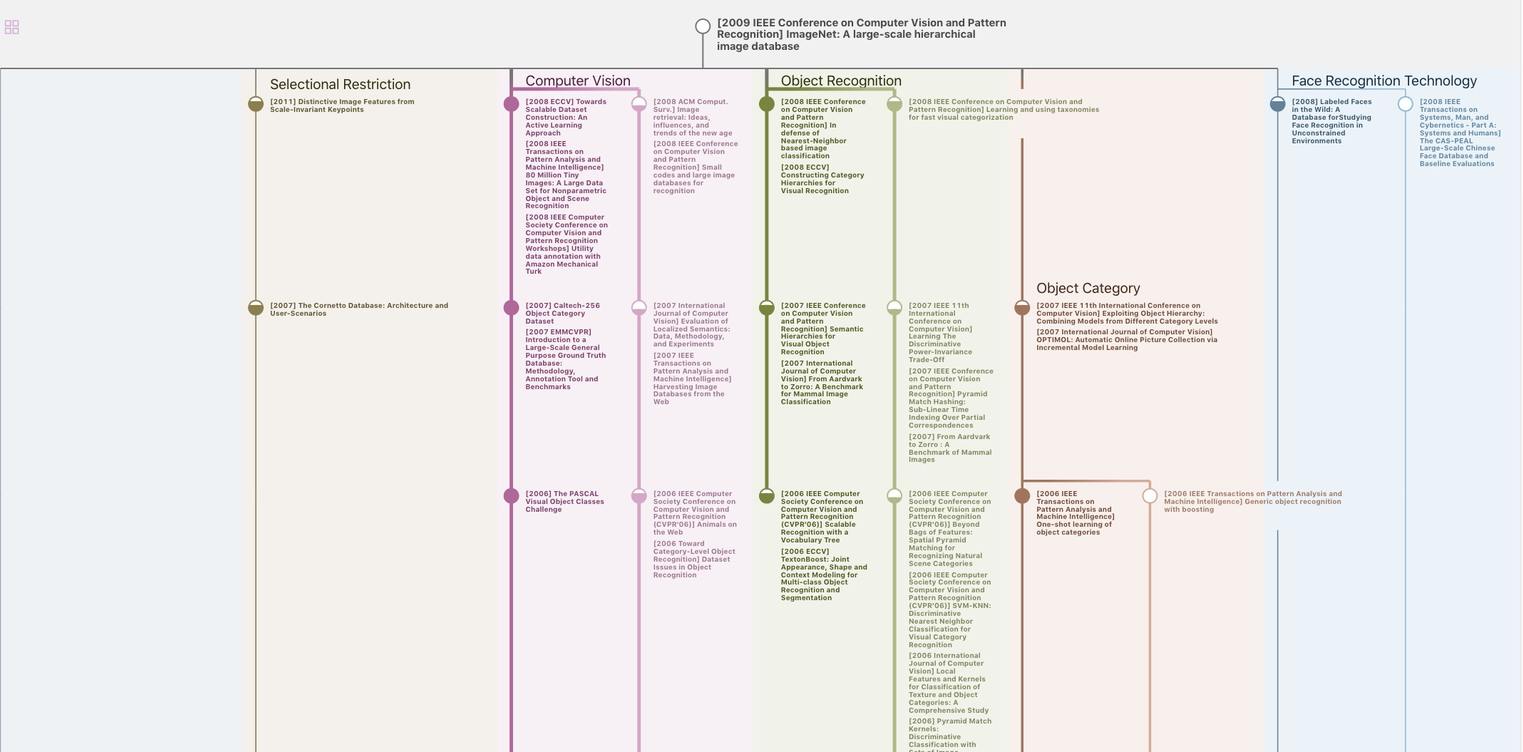
生成溯源树,研究论文发展脉络
Chat Paper
正在生成论文摘要