Wide kernels and their DCT compression in convolutional networks for nuclei segmentation
Informatics in Medicine Unlocked(2023)
摘要
The locality and spatial field of view of image operators have played a major role in image analysis, from hand-crafted to deep learning methods. In Convolutional Neural Networks (CNNs), the field of view is traditionally set to very small values (e.g. 3 × 3 pixels) for individual kernels and grown throughout the network by cascading layers. Automatically learning or adapting the best spatial support of the kernels can be done by using large kernels. Due to the computation requirements of standard CNN architectures, this has been little investigated in the literature. However, if large receptive fields are needed to capture wider contextual information on a given task, it could be learned from the data. Obtaining an optimal receptive field with few layers is very relevant in applications with a limited amount of annotated training data, e.g. in medical imaging.
更多查看译文
关键词
Convolutional network,Segmentation,Receptive field,Discrete cosine transform
AI 理解论文
溯源树
样例
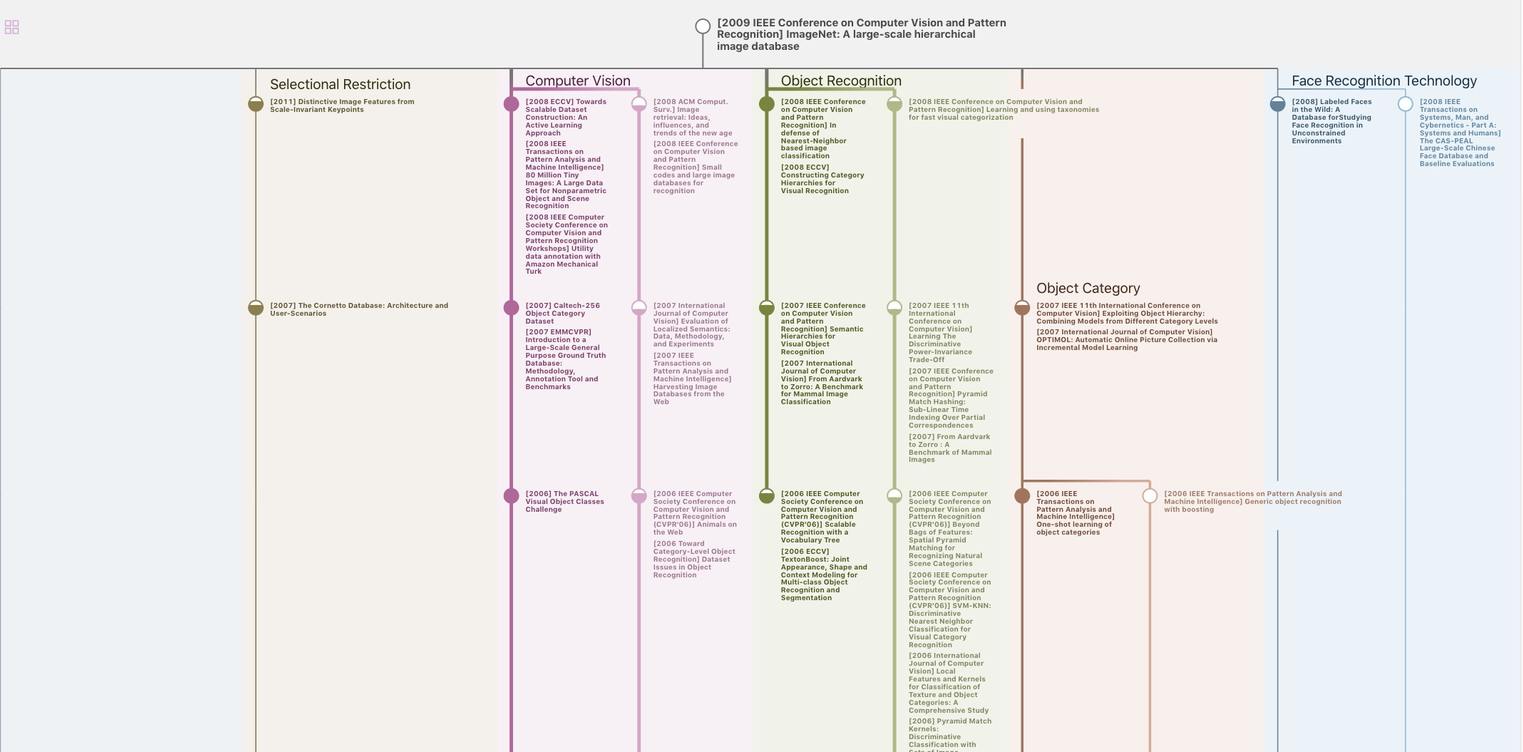
生成溯源树,研究论文发展脉络
Chat Paper
正在生成论文摘要