Short-term wind speed combined forecasting model based on multi-decomposition algorithms and frameworks
ELECTRIC POWER SYSTEMS RESEARCH(2024)
摘要
The normal operation of the electrical grid is directly impacted by the precision of short-term wind speed forecasting. However, nonlinearities in wind velocities make their forecasting challenging. In previous research, the singularity of decomposition methods and frameworks in the model hampered the precision of forecasting. This study proposes an integration model that incorporates multiple decomposition algorithms and frameworks to address this issue. In the preprocessing stage, the model employs multiple decomposition algorithms to decompose the original wind speed data, which effectively reduces the nonlinearity of the wind speed data. Meanwhile, the multiscale decomposition gives more diverse inputs to the sub-model. During the forecasting stage, we utilized multiple frameworks in combination with the new hybrid model to construct sub-models. Using multiple frameworks, we can fully explore the potential information and relationships among subsequences of varying frequencies and their connections with related variables. The Proposed Hybrid model improves the forecasting performance of sub-models. Lastly, the sub-models are recombined using a deep learning nonlinear combination strategy to obtain the final result. The experimental data were obtained from real wind farms. The final forecasts were evaluated using four evaluation metrics. The RMSE of the combined model is 0.1583, the MAE is 0.1241, the MAPE (%) is 1.9119, and the R2 is 0.9948. The proposed combined forecasting model outperforms all baseline models and it makes wind speed forecasting more accurate. In the future, the model could help wind farm maintenance and facilitate power system development.
更多查看译文
关键词
Decomposition technique,Wind speed forecasting,Deep learning,Combined forecasting
AI 理解论文
溯源树
样例
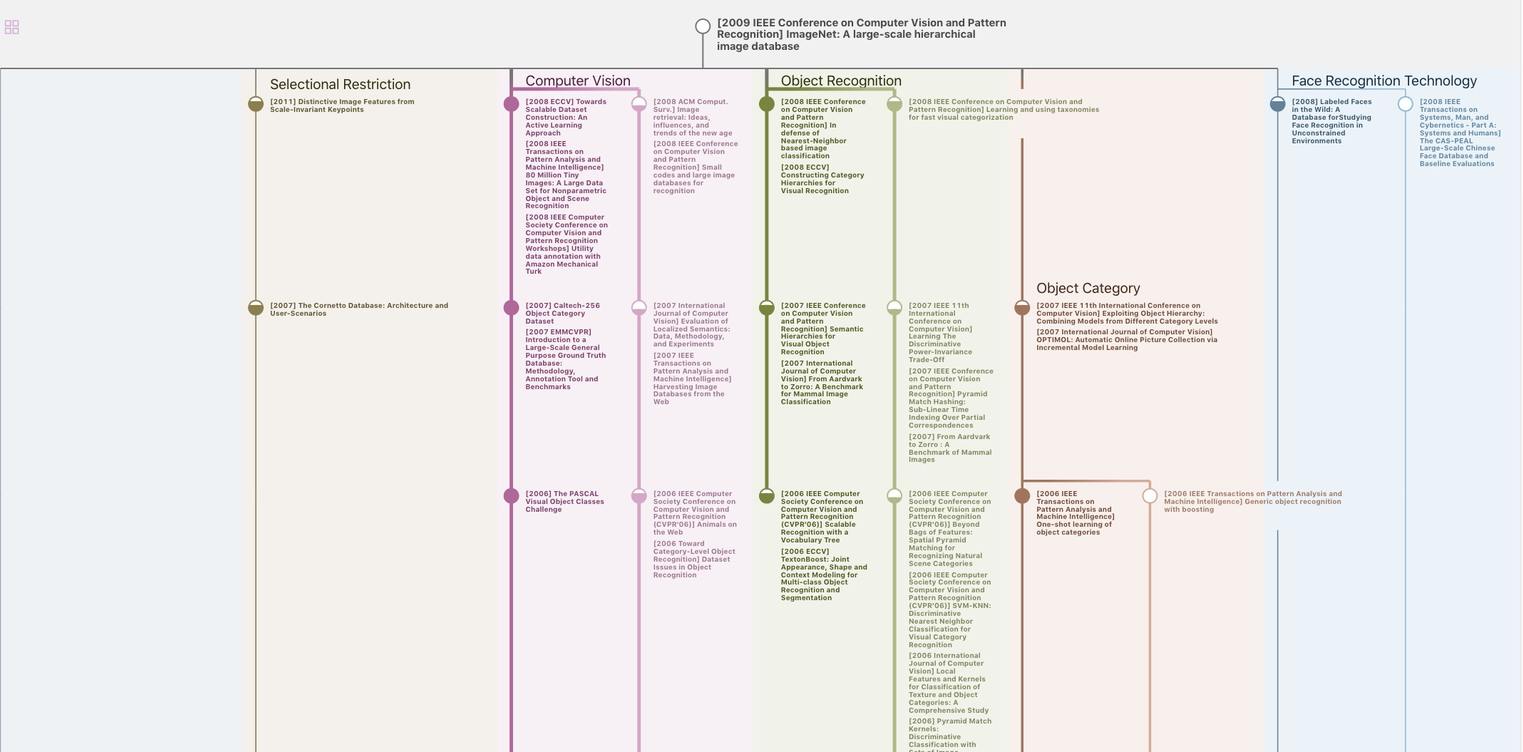
生成溯源树,研究论文发展脉络
Chat Paper
正在生成论文摘要