Revisiting multi-view learning: A perspective of implicitly heterogeneous Graph Convolutional Network
NEURAL NETWORKS(2024)
摘要
Graph Convolutional Network (GCN) has become a hotspot in graph-based machine learning due to its powerful graph processing capability. Most of the existing GCN-based approaches are designed for single-view data. In numerous practical scenarios, data is expressed through multiple views, rather than a single view. The ability of GCN to model homogeneous graphs is indisputable, while it is insufficient in facing the heterophily property of multi-view data. In this paper, we revisit multi-view learning to propose an implicit heterogeneous graph convolutional network that efficiently captures the heterogeneity of multi-view data while exploiting the powerful feature aggregation capability of GCN. We automatically assign optimal importance to each view when constructing the meta-path graph. High-order cross-view meta-paths are explored based on the obtained graph, and a series of graph matrices are generated. Combining graph matrices with learnable global feature representation to obtain heterogeneous graph embeddings at various levels. Finally, in order to effectively utilize both local and global information, we introduce a graph-level attention mechanism at the meta-path level that allocates private information to each node individually. Extensive experimental results convincingly support the superior performance of the proposed method compared to other state-of-the-art approaches.
更多查看译文
关键词
Multi-view learning,Graph convolutional network,Heterogeneous graph,Meta-path
AI 理解论文
溯源树
样例
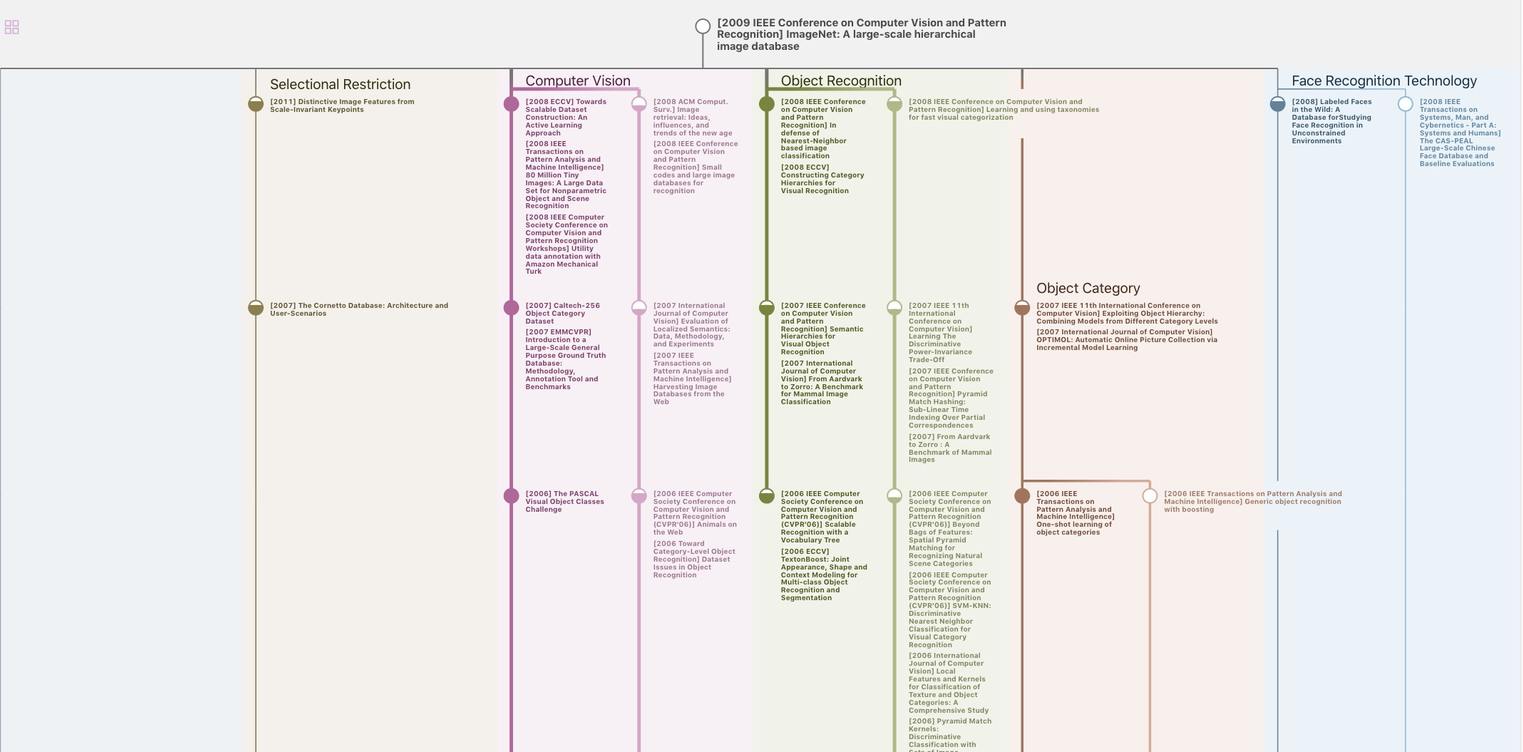
生成溯源树,研究论文发展脉络
Chat Paper
正在生成论文摘要