Lost Your Style? Navigating with Semantic-Level Approach for Text-to-Outfit Retrieval
2024 IEEE/CVF Winter Conference on Applications of Computer Vision (WACV)(2023)
摘要
Fashion stylists have historically bridged the gap between consumers' desires and perfect outfits, which involve intricate combinations of colors, patterns, and materials. Although recent advancements in fashion recommendation systems have made strides in outfit compatibility prediction and complementary item retrieval, these systems rely heavily on pre-selected customer choices. Therefore, we introduce a groundbreaking approach to fashion recommendations: text-to-outfit retrieval task that generates a complete outfit set based solely on textual descriptions given by users. Our model is devised at three semantic levels-item, style, and outfit-where each level progressively aggregates data to form a coherent outfit recommendation based on textual input. Here, we leverage strategies similar to those in the contrastive language-image pretraining model to address the intricate-style matrix within the outfit sets. Using the Maryland Polyvore and Polyvore Outfit datasets, our approach significantly outperformed state-of-the-art models in text-video retrieval tasks, solidifying its effectiveness in the fashion recommendation domain. This research not only pioneers a new facet of fashion recommendation systems, but also introduces a method that captures the essence of individual style preferences through textual descriptions.
更多查看译文
关键词
Applications,Commercial / retail
AI 理解论文
溯源树
样例
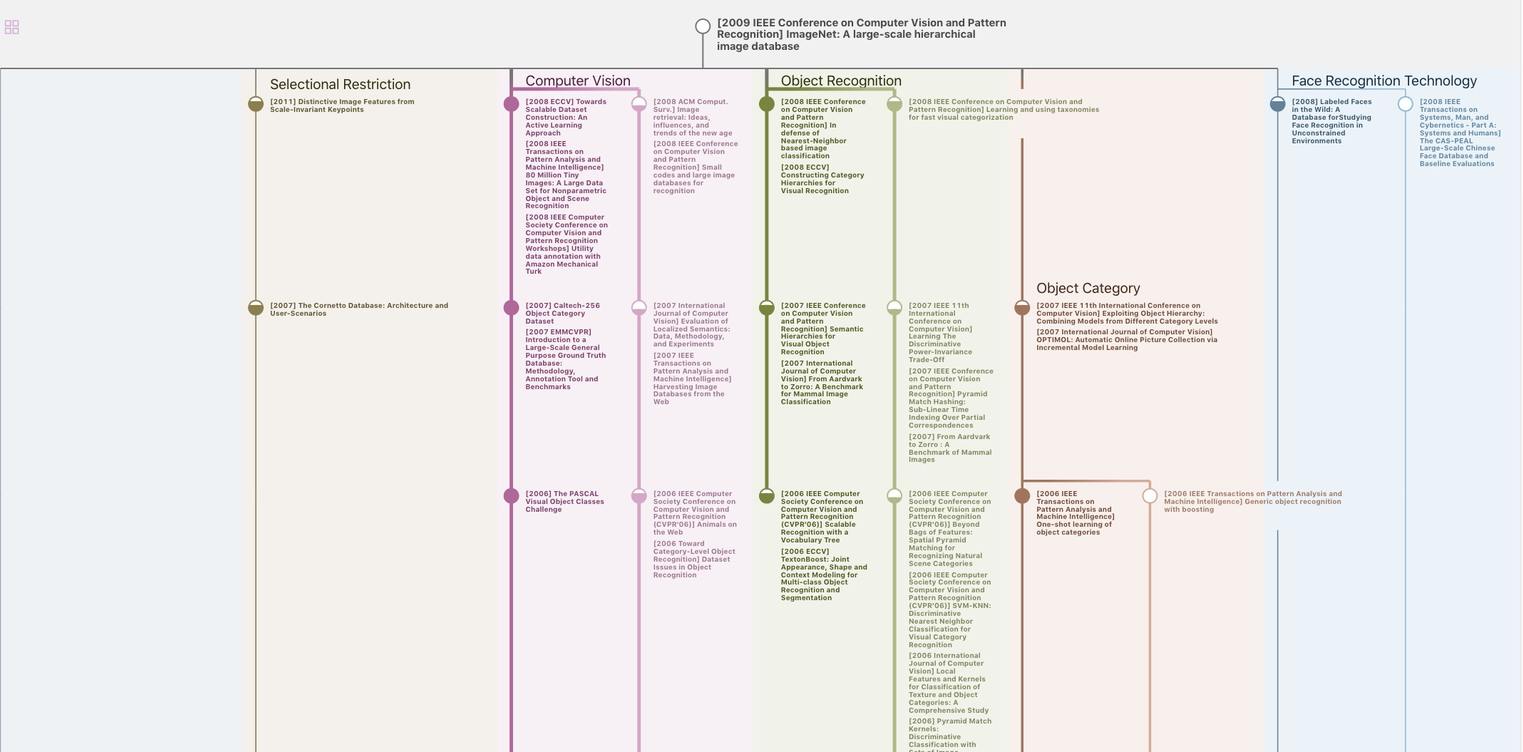
生成溯源树,研究论文发展脉络
Chat Paper
正在生成论文摘要