Using General Value Functions to Learn Domain-Backed Inventory Management Policies.
CoRR(2023)
摘要
We consider the inventory management problem, where the goal is to balance conflicting objectives such as availability and wastage of a large range of products in a store. We propose a reinforcement learning (RL) approach that utilises General Value Functions (GVFs) to derive domain-backed inventory replenishment policies. The inventory replenishment decisions are modelled as a sequential decision making problem, which is challenging due to uncertain demand and the existence of aggregate (cross-product) constraints. In existing literature, GVFs have primarily been used for auxiliary task learning. We use this capability to train GVFs on domain-critical characteristics such as prediction of stock-out probability and wastage quantity. Using this domain expertise for more effective exploration, we train an RL agent to compute the inventory replenishment quantities for a large range of products (up to 6000 in the reported experiments), which share aggregate constraints such as the total weight/volume per delivery. Additionally, we show that the GVF predictions can be used to provide additional domain-backed insights into the decisions proposed by the RL agent. Finally, since the environment dynamics are fully transferred, the trained GVFs can be used for faster adaptation to vastly different business objectives (for example, due to the start of a promotional period or due to deployment in a new customer environment).
更多查看译文
关键词
inventory
management,management policies,general value functions,domain-backed
AI 理解论文
溯源树
样例
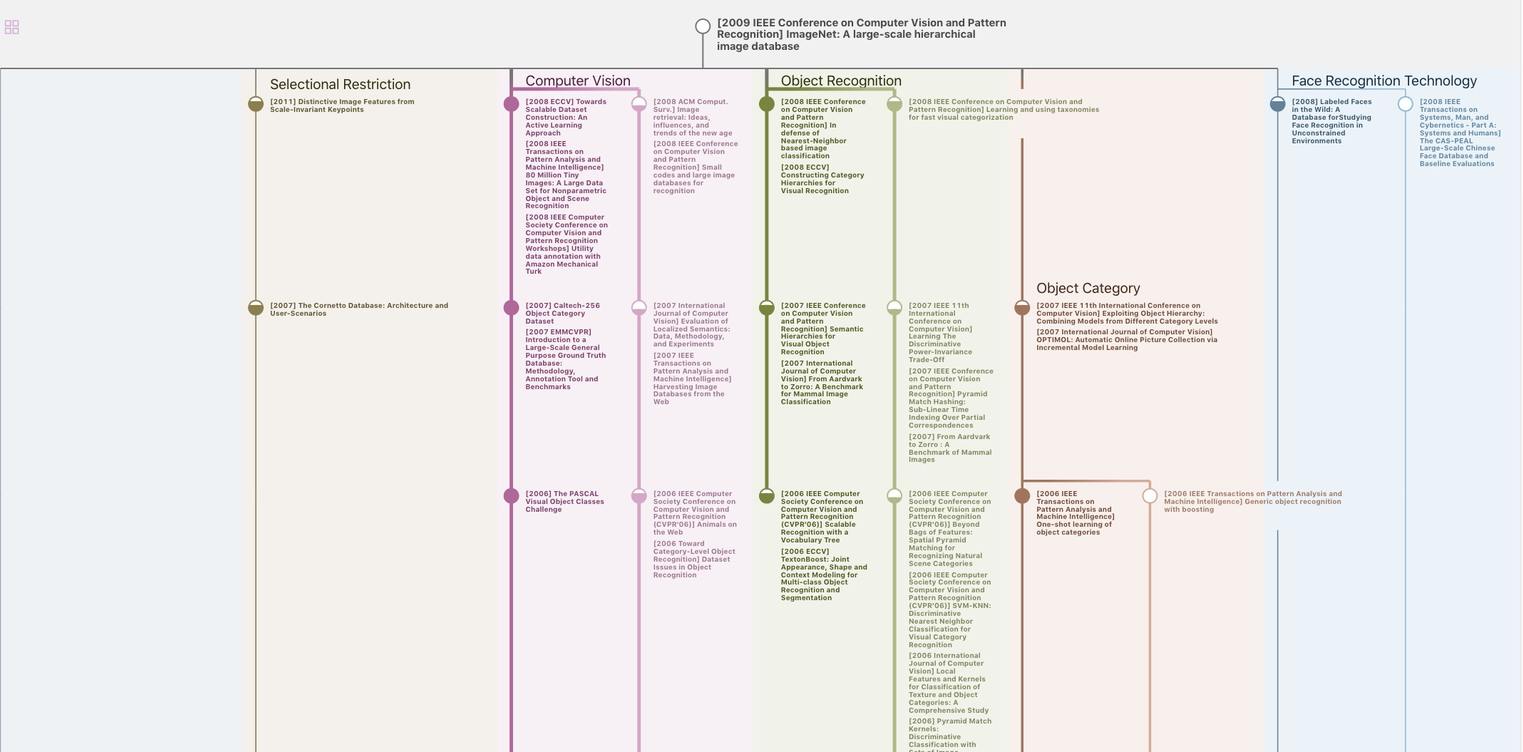
生成溯源树,研究论文发展脉络
Chat Paper
正在生成论文摘要