FragXsiteDTI: Revealing Responsible Segments in Drug-Target Interaction with Transformer-Driven Interpretation.
CoRR(2023)
摘要
Drug-Target Interaction (DTI) prediction is vital for drug discovery, yet challenges persist in achieving model interpretability and optimizing performance. We propose a novel transformer-based model, FragXsiteDTI, that aims to address these challenges in DTI prediction. Notably, FragXsiteDTI is the first DTI model to simultaneously leverage drug molecule fragments and protein pockets. Our information-rich representations for both proteins and drugs offer a detailed perspective on their interaction. Inspired by the Perceiver IO framework, our model features a learnable latent array, initially interacting with protein binding site embeddings using cross-attention and later refined through self-attention and used as a query to the drug fragments in the drug's cross-attention transformer block. This learnable query array serves as a mediator and enables seamless information translation, preserving critical nuances in drug-protein interactions. Our computational results on three benchmarking datasets demonstrate the superior predictive power of our model over several state-of-the-art models. We also show the interpretability of our model in terms of the critical components of both target proteins and drug molecules within drug-target pairs.
更多查看译文
关键词
interaction
AI 理解论文
溯源树
样例
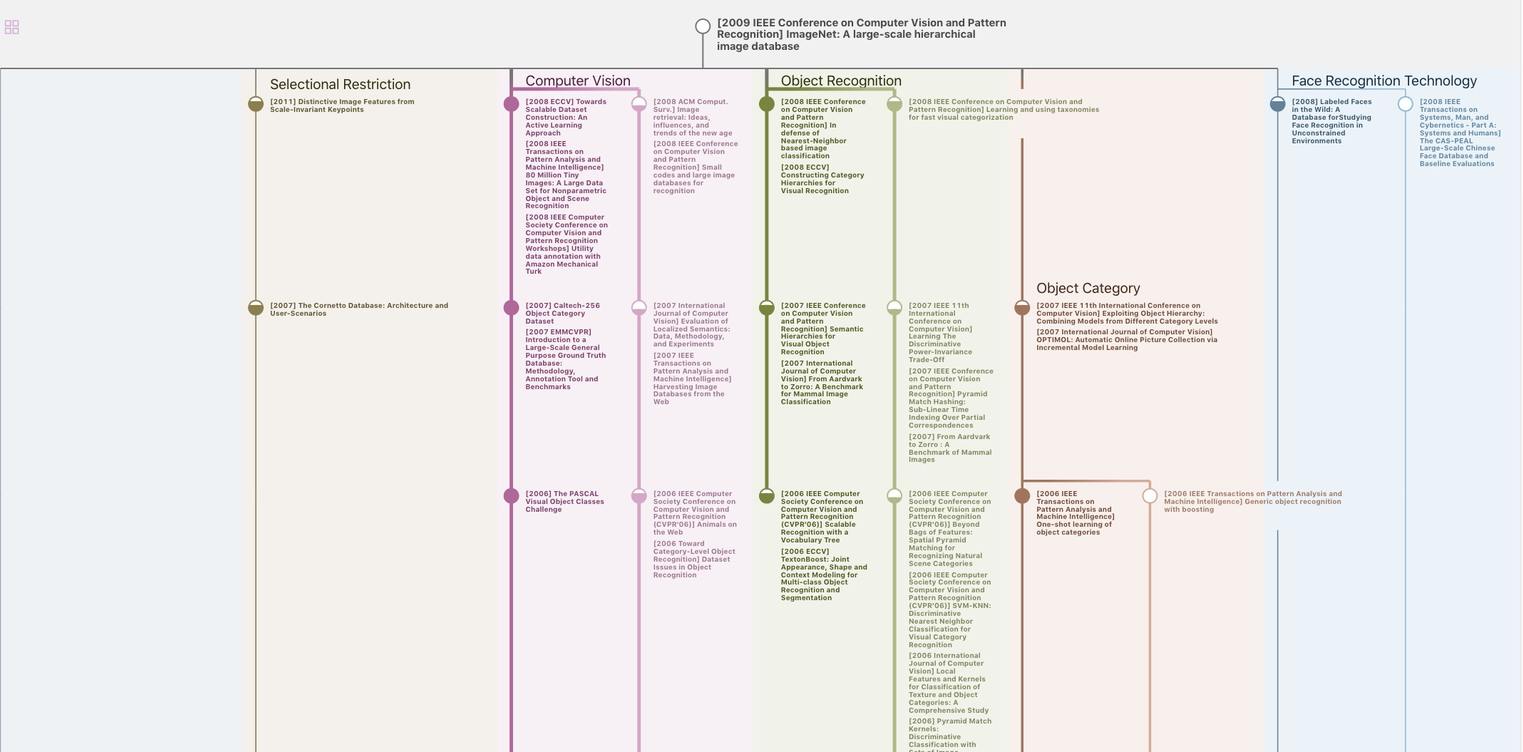
生成溯源树,研究论文发展脉络
Chat Paper
正在生成论文摘要