Octavius: Mitigating Task Interference in MLLMs via LoRA-MoE
arxiv(2023)
摘要
Recent studies have demonstrated Large Language Models (LLMs) can extend
their zero-shot generalization capabilities to multimodal learning through
instruction tuning. As more modalities and downstream tasks are introduced,
negative conflicts and interference may have a worse impact on performance.
While this phenomenon has been overlooked in previous work, we propose a novel
and extensible framework, called Octavius, for comprehensive studies and
experimentation on multimodal learning with Multimodal Large Language Models
(MLLMs). Specifically, we combine the well-known Mixture-of-Experts (MoE) and
one of the representative PEFT techniques, i.e., LoRA, designing a novel
LLM-based decoder, called LoRA-MoE, for multimodal learning. To the best of our
knowledge, we are one of the pioneering efforts to introduce MoE into MLLMs to
address this problem. The experimental results (about 20
shown the effectiveness and versatility of our design in various 2D and 3D
downstream tasks. Code and datasets are available at
https://openlamm.github.io/paper_list/Octavius.
更多查看译文
AI 理解论文
溯源树
样例
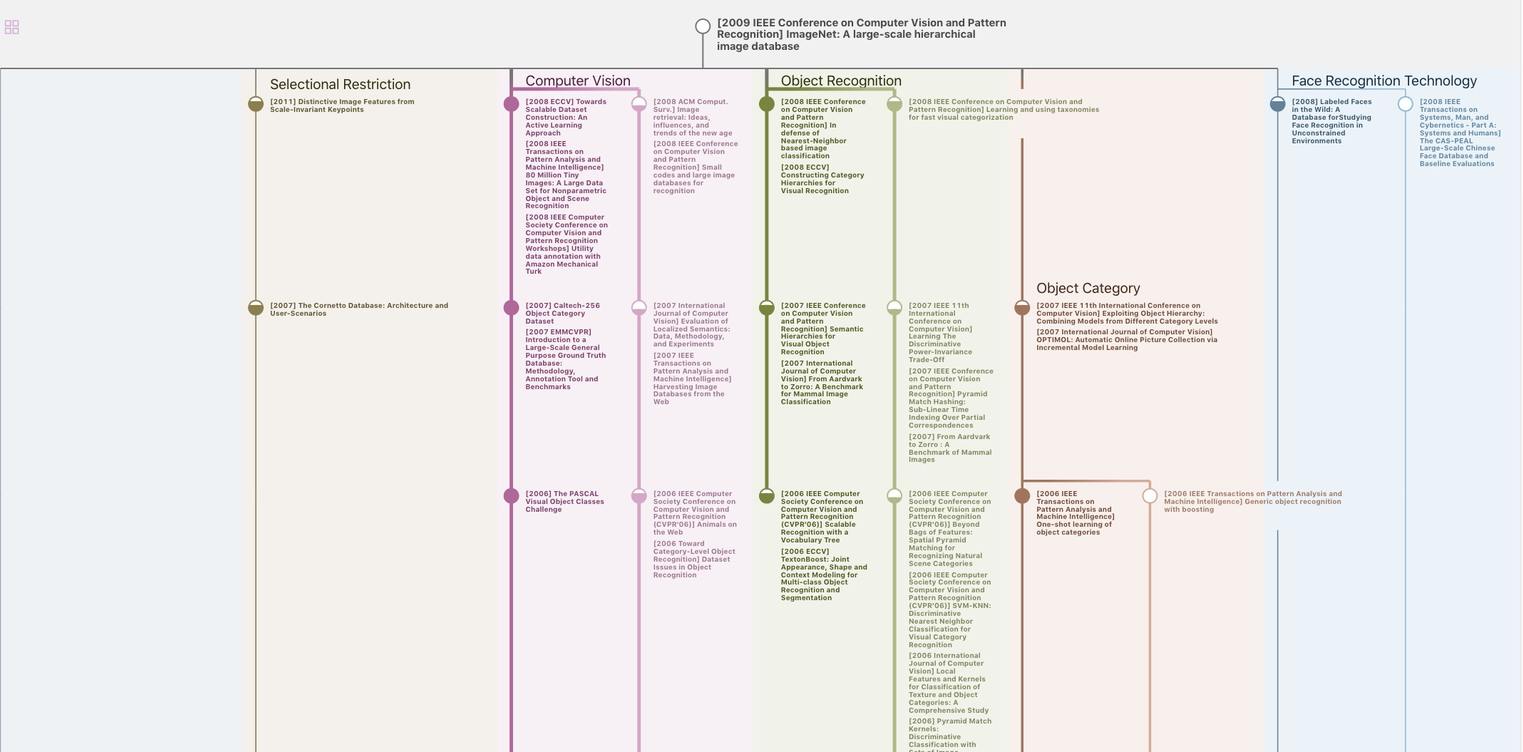
生成溯源树,研究论文发展脉络
Chat Paper
正在生成论文摘要