AttentioNet: Monitoring Student Attention Type in Learning with EEG-Based Measurement System
2023 11TH INTERNATIONAL CONFERENCE ON AFFECTIVE COMPUTING AND INTELLIGENT INTERACTION, ACII(2023)
摘要
Student attention is an indispensable input for uncovering their goals, intentions, and interests, which prove to be invaluable for a multitude of research areas, ranging from psychology to interactive systems. However, most existing methods to classify attention fail to model its complex nature. To bridge this gap, we propose AttentioNet, a novel Convolutional Neural Network-based approach that utilizes Electroencephalography (EEG) data to classify attention into five states: Selective, Sustained, Divided, Alternating, and relaxed state. We collected a dataset of 20 subjects through standard neuropsychological tasks to elicit different attentional states. The average across-student accuracy of our proposed model at this configuration is 92.3% (SD=3.04), which is well-suited for end-user applications. Our transfer learning-based approach for personalizing the model to individual subjects effectively addresses the issue of individual variability in EEG signals, resulting in improved performance and adaptability of the model for real-world applications. This represents a significant advancement in the field of EEG-based classification. Experimental results demonstrate that AttentioNet outperforms a popular EEGnet baseline (p-value < 0.05) in both subject-independent and subject-dependent settings, confirming the effectiveness of our proposed approach despite the limitations of our dataset. These results highlight the promising potential of AttentioNet for attention classification using EEG data.
更多查看译文
关键词
EEG,Attention,Affective Computing,Cognitive Engagement Assessment
AI 理解论文
溯源树
样例
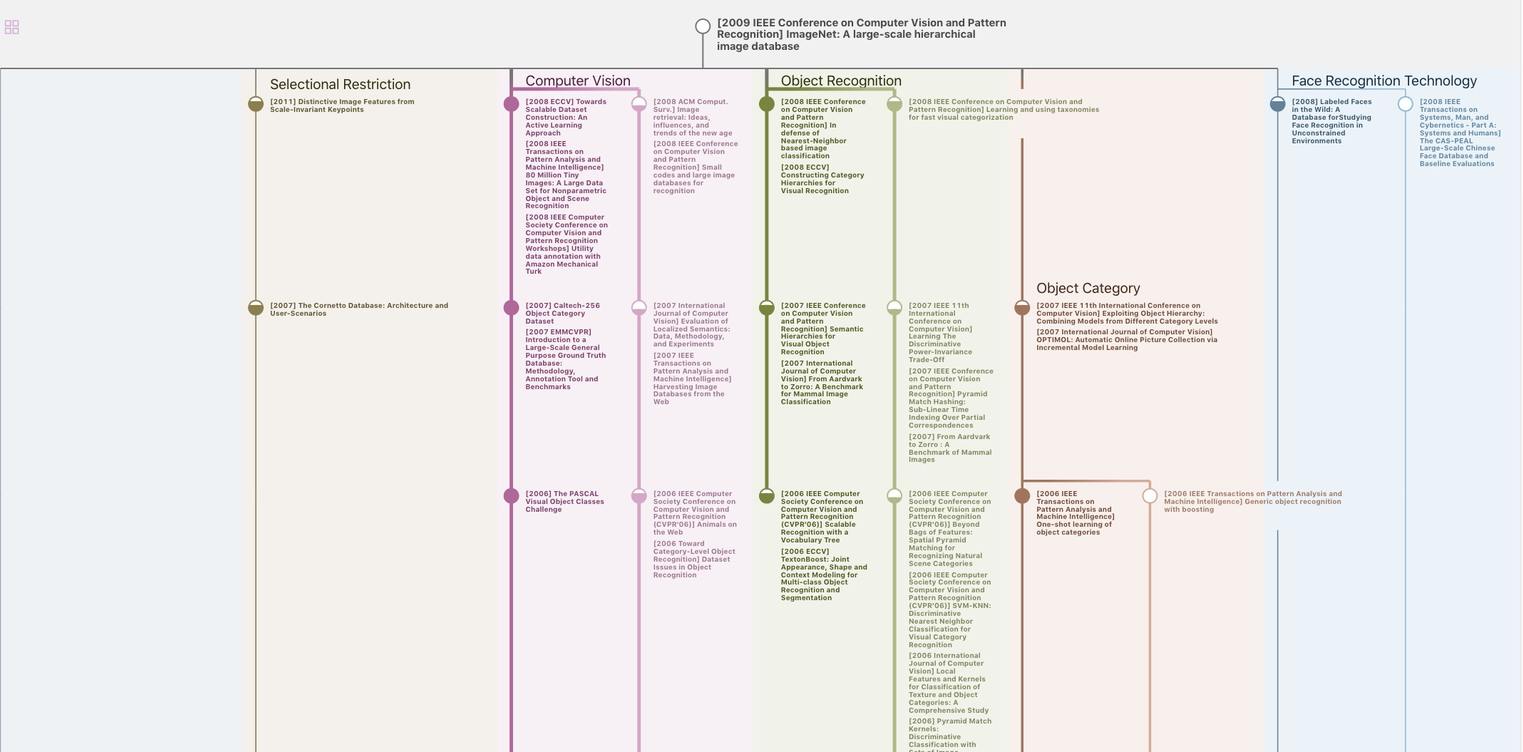
生成溯源树,研究论文发展脉络
Chat Paper
正在生成论文摘要