Modeling blazar broadband emission with convolutional neural networks - I. Synchrotron self-Compton model
arXiv (Cornell University)(2023)
摘要
Modeling the multiwavelength spectral energy distributions (SEDs) of blazars provides key insights into the underlying physical processes responsible for the emission. While SED modeling with self-consistent models is computationally demanding, it is essential for a comprehensive understanding of these astrophysical objects. We introduce a novel, efficient method for modeling the SEDs of blazars by the mean of a convolutional neural network (CNN). In this paper, we trained the CNN on a leptonic model that incorporates synchrotron and inverse Compton emissions, as well as self-consistent electron cooling and pair creation-annihilation processes. The CNN is capable of reproducing the radiative signatures of blazars with high accuracy. This approach significantly reduces computational time, thereby enabling real-time fitting to multi-wavelength datasets. As a demonstration, we used the trained CNN with MultiNest to fit the broadband SEDs of Mrk 421 and 1ES 1959+650, successfully obtaining their parameter posterior distributions. This novel framework for fitting the SEDs of blazars will be further extended to incorporate more sophisticated models based on external Compton and hadronic scenarios, allowing for multi-messenger constraints in the analysis. The models will be made publicly available via a web interface, the Markarian Multiwavelength Datacenter, to facilitate self-consistent modeling of multi-messenger data from blazar observations.
更多查看译文
关键词
blazar broadband emission,convolutional neural networks,self-compton
AI 理解论文
溯源树
样例
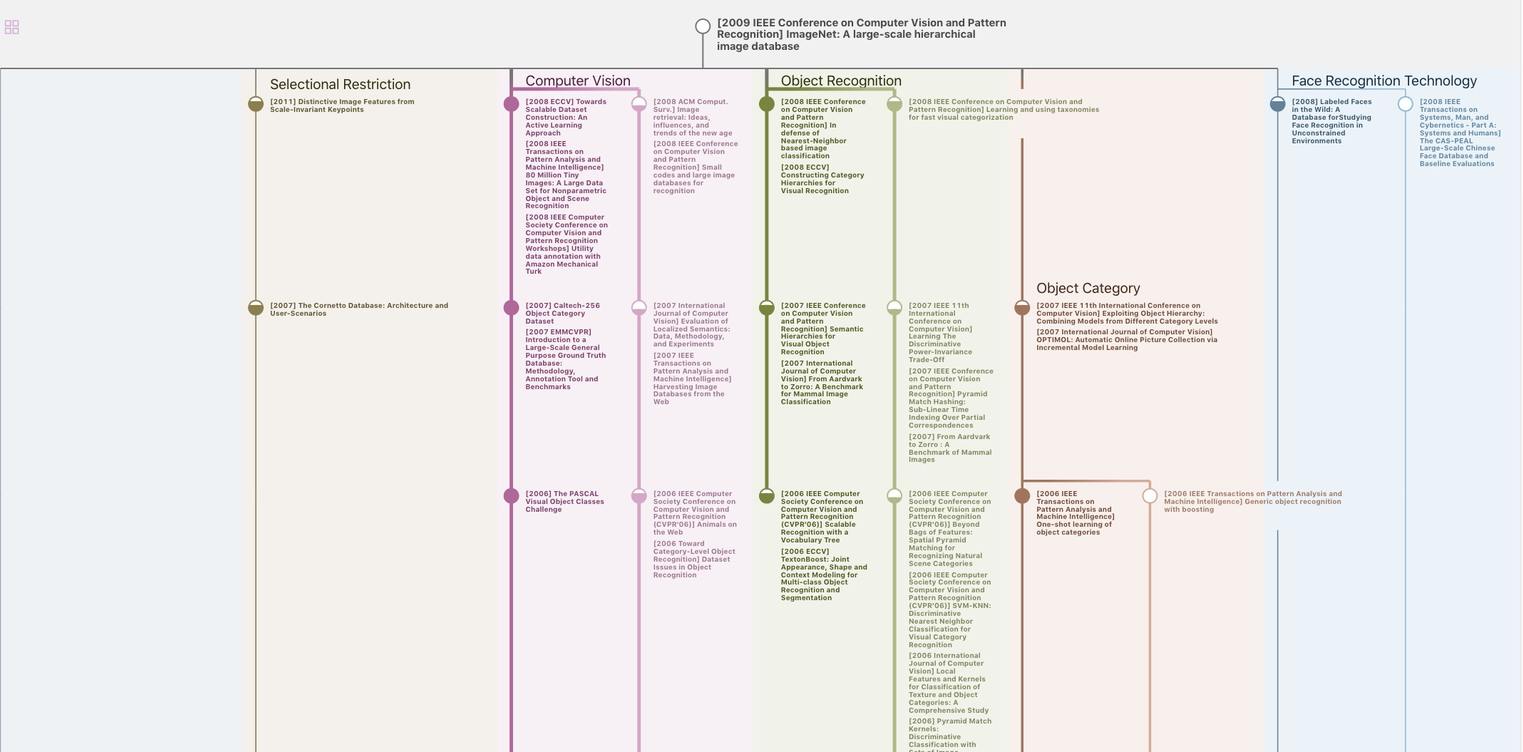
生成溯源树,研究论文发展脉络
Chat Paper
正在生成论文摘要