Leveraging Transformers to Improve Breast Cancer Classification and Risk Assessment with Multi-modal and Longitudinal Data.
CoRR(2023)
摘要
Breast cancer screening, primarily conducted through mammography, is often supplemented with ultrasound for women with dense breast tissue. However, existing deep learning models analyze each modality independently, missing opportunities to integrate information across imaging modalities and time. In this study, we present Multi-modal Transformer (MMT), a neural network that utilizes mammography and ultrasound synergistically, to identify patients who currently have cancer and estimate the risk of future cancer for patients who are currently cancer-free. MMT aggregates multi-modal data through self-attention and tracks temporal tissue changes by comparing current exams to prior imaging. Trained on 1.3 million exams, MMT achieves an AUROC of 0.943 in detecting existing cancers, surpassing strong uni-modal baselines. For 5-year risk prediction, MMT attains an AUROC of 0.826, outperforming prior mammography-based risk models. Our research highlights the value of multi-modal and longitudinal imaging in cancer diagnosis and risk stratification.
更多查看译文
关键词
breast cancer classification,risk
assessment,breast cancer,multi-modal
AI 理解论文
溯源树
样例
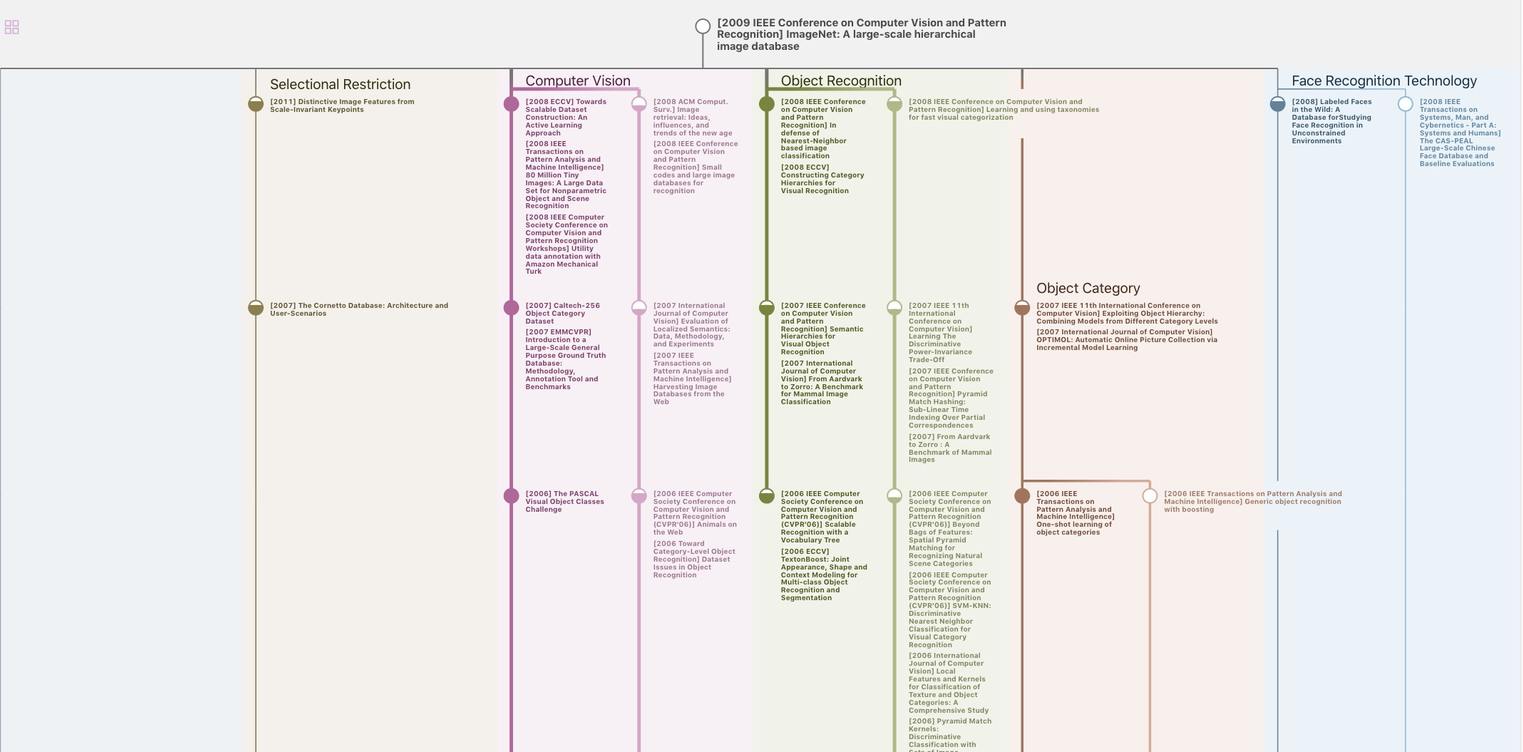
生成溯源树,研究论文发展脉络
Chat Paper
正在生成论文摘要