Segmentation of Drone Collision Hazards in Airborne RADAR Point Clouds Using PointNet.
CoRR(2023)
摘要
The integration of unmanned aerial vehicles (UAVs) into shared airspace for beyond visual line of sight (BVLOS) operations presents significant challenges but holds transformative potential for sectors like transportation, construction, energy and defense. A critical prerequisite for this integration is equipping UAVs with enhanced situational awareness to ensure safe operations. Current approaches mainly target single object detection or classification, or simpler sensing outputs that offer limited perceptual understanding and lack the rapid end-to-end processing needed to convert sensor data into safety-critical insights. In contrast, our study leverages radar technology for novel end-to-end semantic segmentation of aerial point clouds to simultaneously identify multiple collision hazards. By adapting and optimizing the PointNet architecture and integrating aerial domain insights, our framework distinguishes five distinct classes: mobile drones (DJI M300 and DJI Mini) and airplanes (Ikarus C42), and static returns (ground and infrastructure) which results in enhanced situational awareness for UAVs. To our knowledge, this is the first approach addressing simultaneous identification of multiple collision threats in an aerial setting, achieving a robust 94% accuracy. This work highlights the potential of radar technology to advance situational awareness in UAVs, facilitating safe and efficient BVLOS operations.
更多查看译文
关键词
drone collision hazards,airborne radar point clouds
AI 理解论文
溯源树
样例
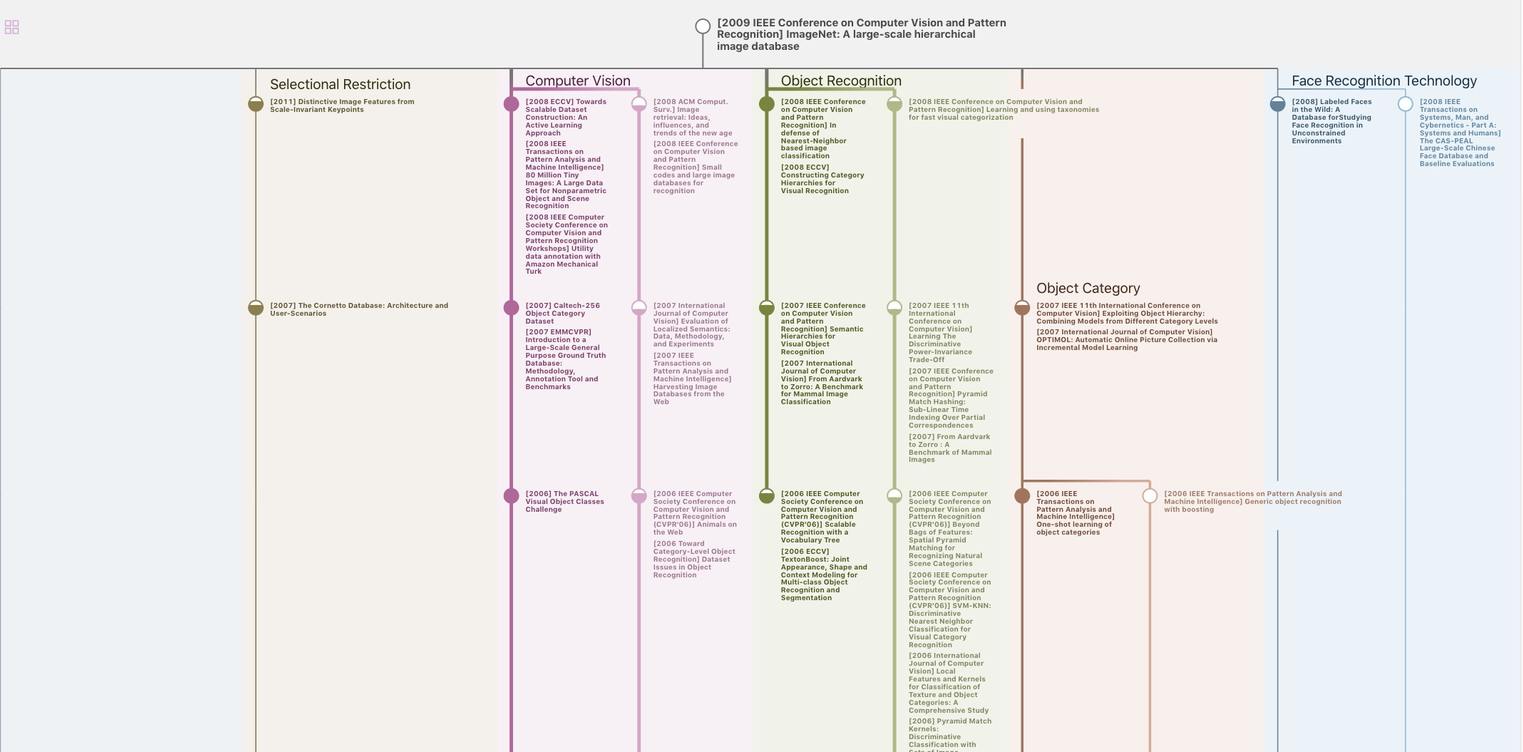
生成溯源树,研究论文发展脉络
Chat Paper
正在生成论文摘要