IC-VAE: A Novel Deep Learning Framework for Interpreting Multiplexed Tissue Imaging Data
bioRxiv (Cold Spring Harbor Laboratory)(2023)
摘要
Interpreting protein expression in multiplexed tissue imaging data presents a significant challenge due to the high dimensionality of the resulting images, the variety of intracellular structures, cell shapes resulting from 2-D tissue sectioning, and the presence of technological noise and imaging artifacts. Here, we introduce the Information-Controlled Variational Autoencoder (IC-VAE), a deep generative model designed to tackle this challenge. The contribution of IC-VAE to the VAE framework is the ability to control the shared information among latent subspaces. We use IC-VAE to factorize each cell’s image into its true protein expression, various cellular components, and background noise, while controlling the shared information among some of these components. Compared with other normalization methods, this approach leads to superior results in downstream analysis, such as analyzing the expression of biomarkers, classification for cell types, or visualizing cell clusters using t-SNE/UMAP techniques.
### Competing Interest Statement
Authors are working at BioTuring Inc., a Computational Biology company.
更多查看译文
关键词
deep learning,imaging,novel deep learning framework,ic-vae
AI 理解论文
溯源树
样例
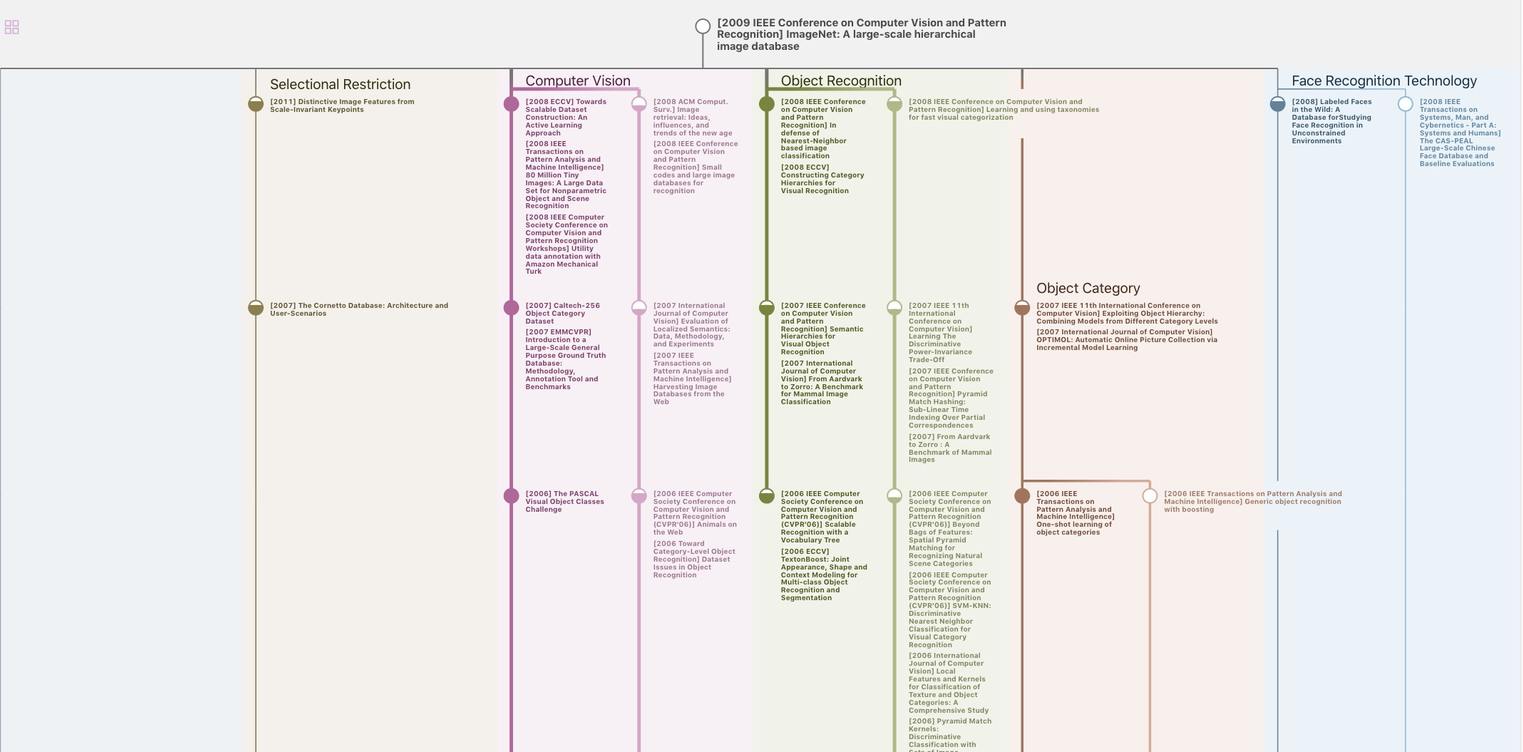
生成溯源树,研究论文发展脉络
Chat Paper
正在生成论文摘要