Identifying local associations in biological time series: algorithms, statistical significance, and applications
BRIEFINGS IN BIOINFORMATICS(2023)
摘要
Local associations refer to spatial-temporal correlations that emerge from the biological realm, such as time-dependent gene co-expression or seasonal interactions between microbes. One can reveal the intricate dynamics and inherent interactions of biological systems by examining the biological time series data for these associations. To accomplish this goal, local similarity analysis algorithms and statistical methods that facilitate the local alignment of time series and assess the significance of the resulting alignments have been developed. Although these algorithms were initially devised for gene expression analysis from microarrays, they have been adapted and accelerated for multi-omics next generation sequencing datasets, achieving high scientific impact. In this review, we present an overview of the historical developments and recent advances for local similarity analysis algorithms, their statistical properties, and real applications in analyzing biological time series data. The benchmark data and analysis scripts used in this review are freely available at http://github.com/labxscut/lsareview.
更多查看译文
关键词
Time series data,Local association,Local alignment,Local similarity analysis,Local trend analysis,Statistical significance
AI 理解论文
溯源树
样例
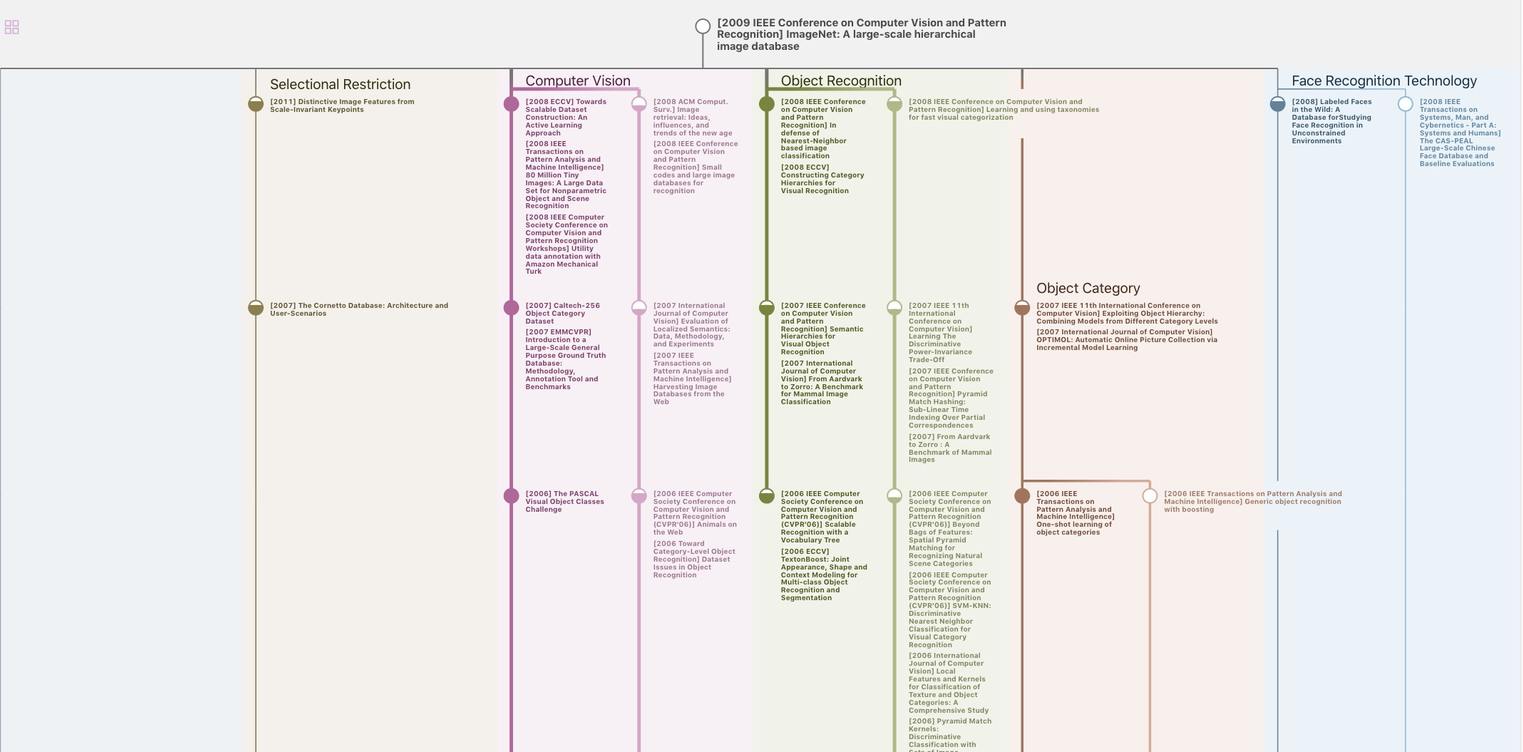
生成溯源树,研究论文发展脉络
Chat Paper
正在生成论文摘要