Unraveling the Potential of Heart Rate Variability for Type 1 Diabetes Prediction
2023 International Workshop on Biomedical Applications, Technologies and Sensors (BATS)(2023)
摘要
Type 1 diabetes (T1D) is an irremediable chronic disease with reduced metabolic functions of pancreatic
$\beta$
-cells, causing insulin diminution and elevated blood glucose concentration. Automatic diagnosis of T1D is crucial in early clinical interventions followed by appropriate management initiatives. This work explores different machine learning (ML) techniques and the ensemble of ML to non-invasively detect T1D patients exploiting the heart rate variability (HRV) captured from ECG. The ensemble classifiers are formed by assigning weights to each ML model, where the area under the receiver operating characteristics curve (AUC) is used as weights, while soft voting generates classification results. An extensive experiment has been carried out to find the appropriate hyperparameters for each ML model through grid search, while AUC is selected as the performance metric due to unbiasedness to class imbalance. A total of 115 ECG recordings (36 diabetics, 79 healthy) from 29 subjects (20 healthy, 9 T1D patients) of the D1NAMO dataset are used in the experiments. The highest classification performance is achieved using the k-nearest neighbor (KNN) classifier achieving an AUC of
$0.750\pm 0.090$
. The outcome of this work will enhance the understanding of distinct HRV features of diabetic and healthy individuals in T1D diagnosis and characterize the impacts of T1D on cardiac asynchrony.
更多查看译文
关键词
electrocardiogram,heart rate variability,machine learning,non-invasive detection,type 1 diabetes
AI 理解论文
溯源树
样例
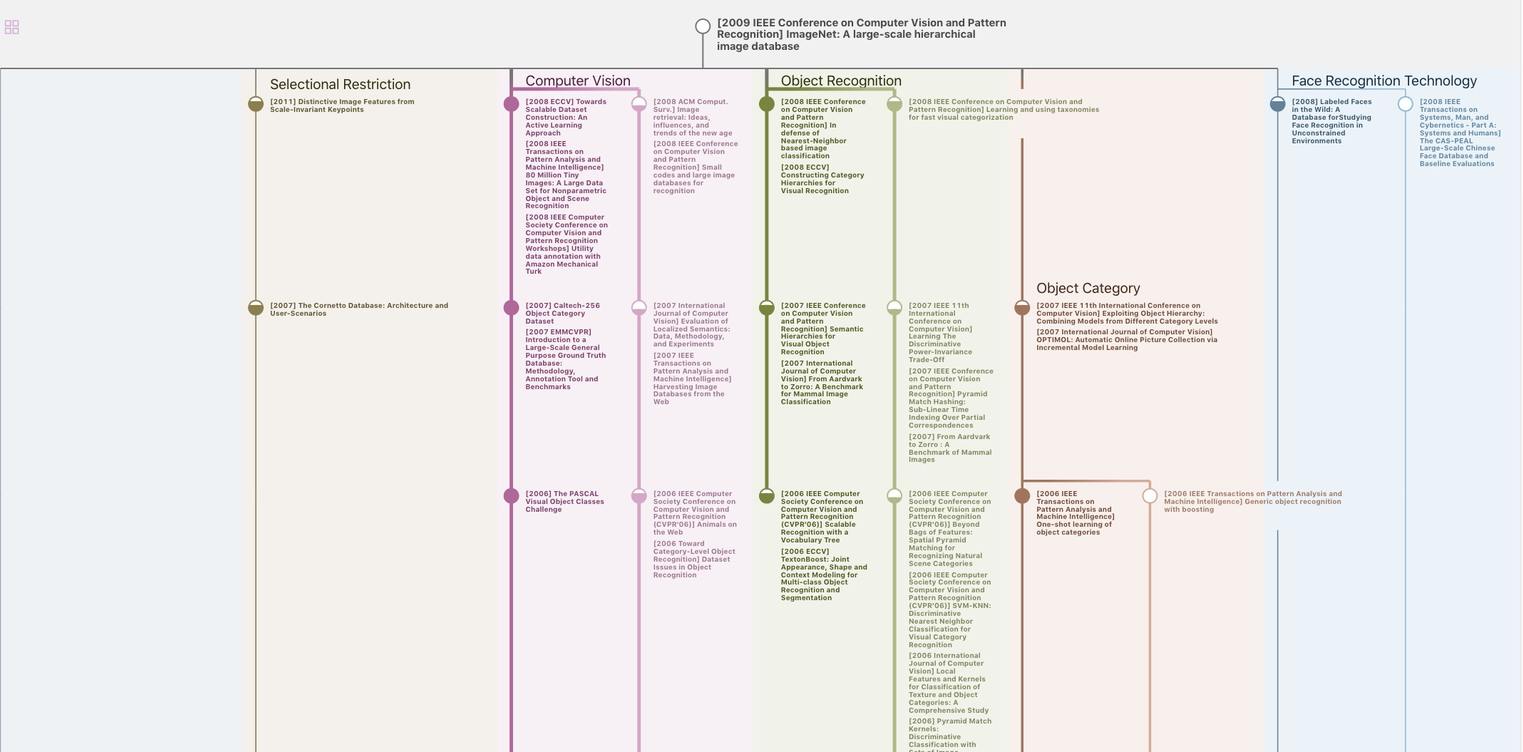
生成溯源树,研究论文发展脉络
Chat Paper
正在生成论文摘要