An Improved AdaBoost Method in Imbalanced Data Learning
2023 International Conference on Cyber-Physical Social Intelligence (ICCSI)(2023)
摘要
Learning from imbalanced datasets has recently received increasing research attention. Despite the remarkable results of AdaBoost in balanced situation, the imbalance problem remains to be solved. To address this, this paper proposes a novel method to improve the AdaBoost using a new error factor and a weighted voting parameter for a few classes and weak classifiers. Our method can weaken the dominant role of the majority class in iterative training by improving the classification ability of the minority class. The superiority of the proposed algorithm is finally proved theoretically and practically. Several real-world imbalance datasets in experiments as specific support, and our method outperforms previous algorithms, especially in the F-1 metric.
更多查看译文
关键词
Highly imbalanced learning,adaptive boosting,weighted parameters adjustment,area under curve
AI 理解论文
溯源树
样例
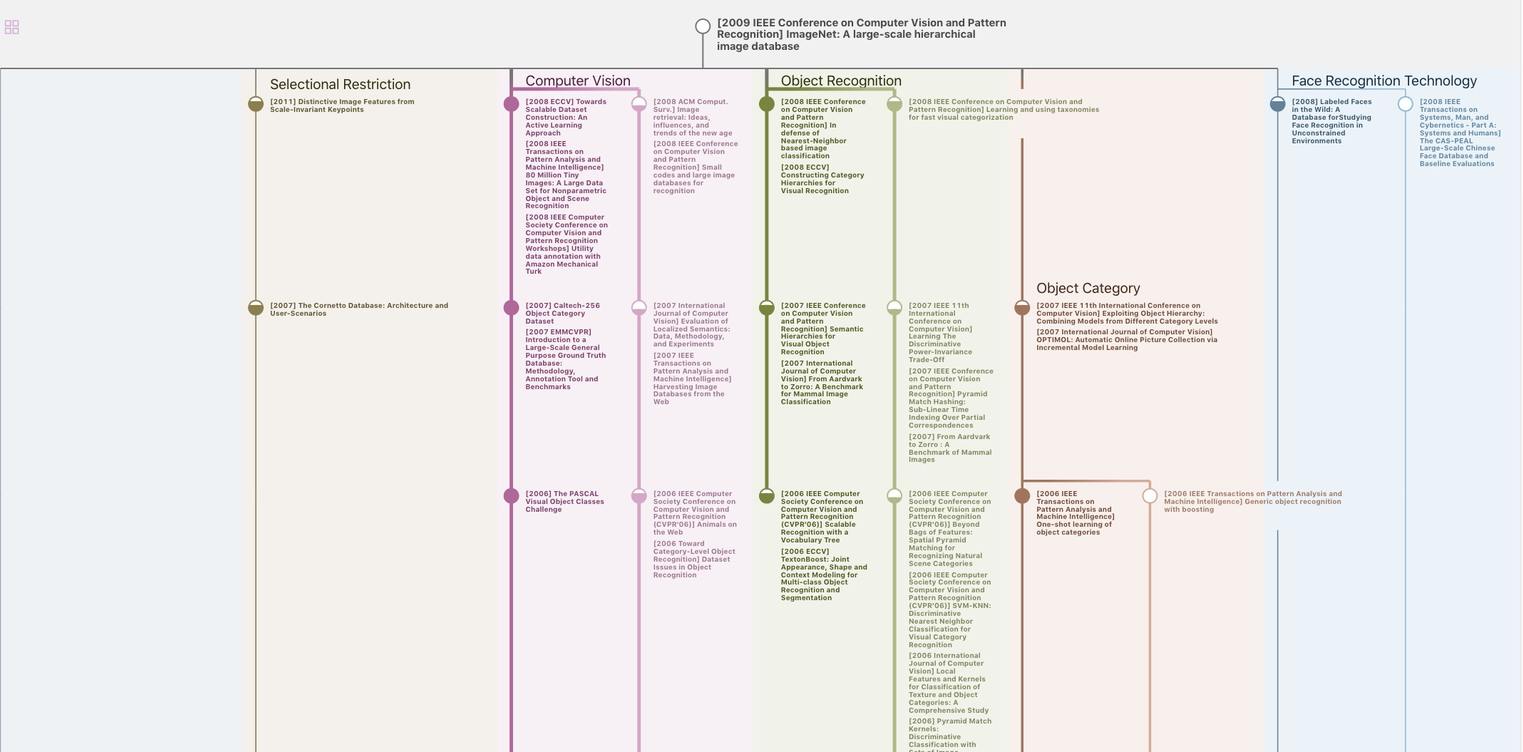
生成溯源树,研究论文发展脉络
Chat Paper
正在生成论文摘要