Radio Frequency Fingerprints Identification based on GAN Networks
2023 IEEE 24th International Workshop on Signal Processing Advances in Wireless Communications (SPAWC)(2023)
摘要
In this work, we tackle challenges in Radio Frequency Fingerprint Identification (RFFI) related to insufficient training data and out-of-data (OOD) situations. The great success of generative-based models in deep learning, i.e., the great potential of generative adversarial networks (GANs) for deep learning-based data augmentation and OOD detection, prompted us to propose GAN-based approaches to improve the recognition accuracy of the supervised learning tasks in RFFI systems, as well as improving the success rate in anomalous data detection. Due to the temporal correlation of electromagnetic signals, we propose a one-dimensional convolution-based Auxiliary Classifier GAN (AC-GAN) network for data augmentation and a one-dimensional convolution-based RF-GANomaly network for anomalous data detection. The experimental results on real-world data show that the proposed GAN-based data augmentation method significantly improves recognition accuracy, and the proposed RF-GANomaly model achieves superior anomalous data detection performance compared to our baseline GAN-based anomalous data detection methods.
更多查看译文
关键词
RF fingerprint identification,GAN,data augmentation,anomalous data detection
AI 理解论文
溯源树
样例
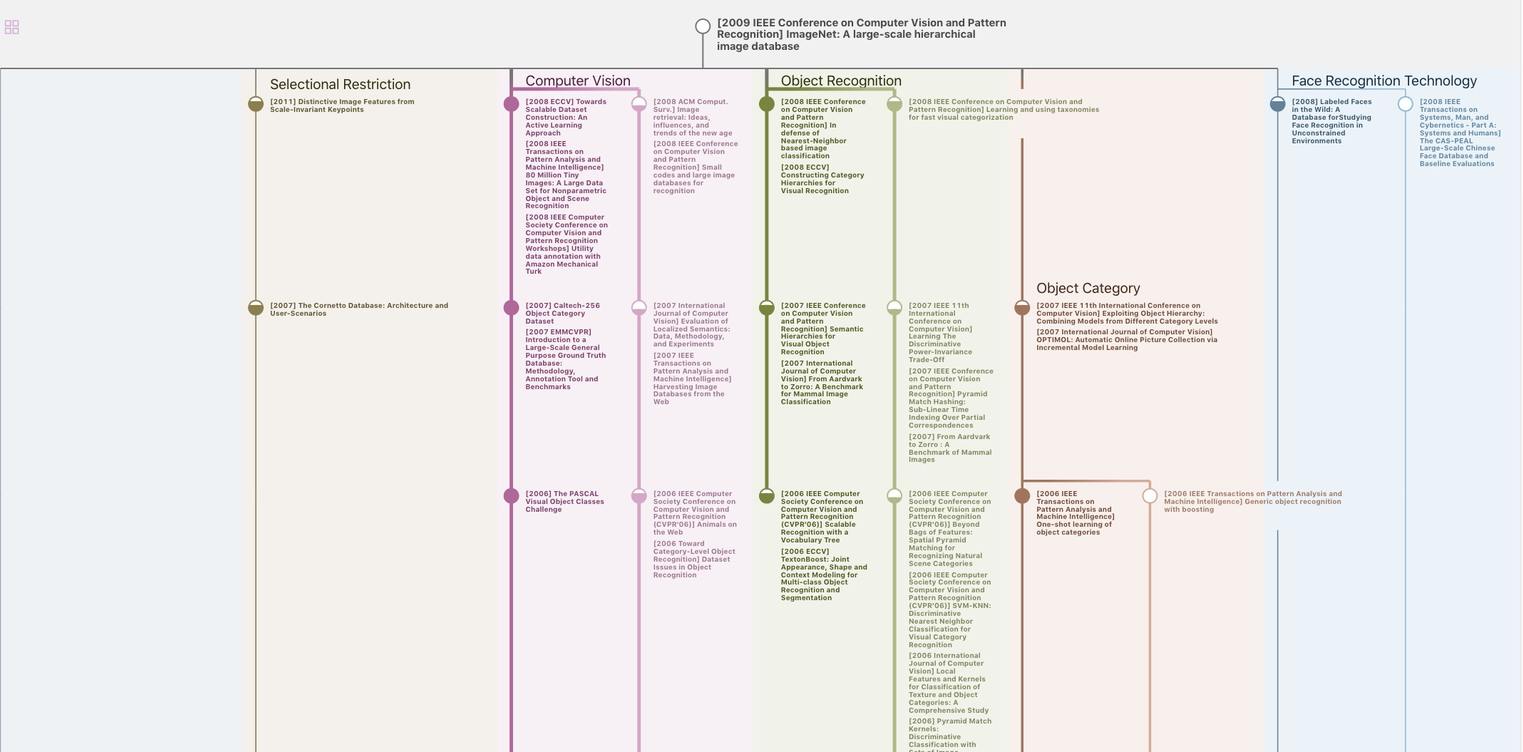
生成溯源树,研究论文发展脉络
Chat Paper
正在生成论文摘要