Privacy-Preserving User Abnormal Behavior Detection in 5G Networks
2023 IEEE International Black Sea Conference on Communications and Networking (BlackSeaCom)(2023)
摘要
Network Data Analytics Function (NWDAF) plays a key role in autonomous network management and security using machine learning (ML) techniques. However, not all ML methods such as convolutional neural networks, deep language models can be deployed in the core network due to their high computational requirements. Besides, telcos may prefer handing over ML analytics to experienced third parties instead of implementing themselves. In this paper, we show that delay-tolerant tasks of NWDAF can be offloaded to public third party cloud providers while preserving user and data privacy by employing homomorphic encryption (HE). We focus on the problem of abnormal behaviour detection for a group of user equipment (UE) and train various DL models with plaintext data. We demonstrate that inferencing on encrypted data is as accurate and precise as plaintext inferencing and we quantify the performance degradation in terms of running times. We believe that offloading NWDAF tasks to the cloud allows telcos to focus on expanding their core capabilities, and reduce capital and operational expenditures.
更多查看译文
关键词
5G,NWDAF,Privacy-Preserving,Deep Learning,Homomorphic Encryption
AI 理解论文
溯源树
样例
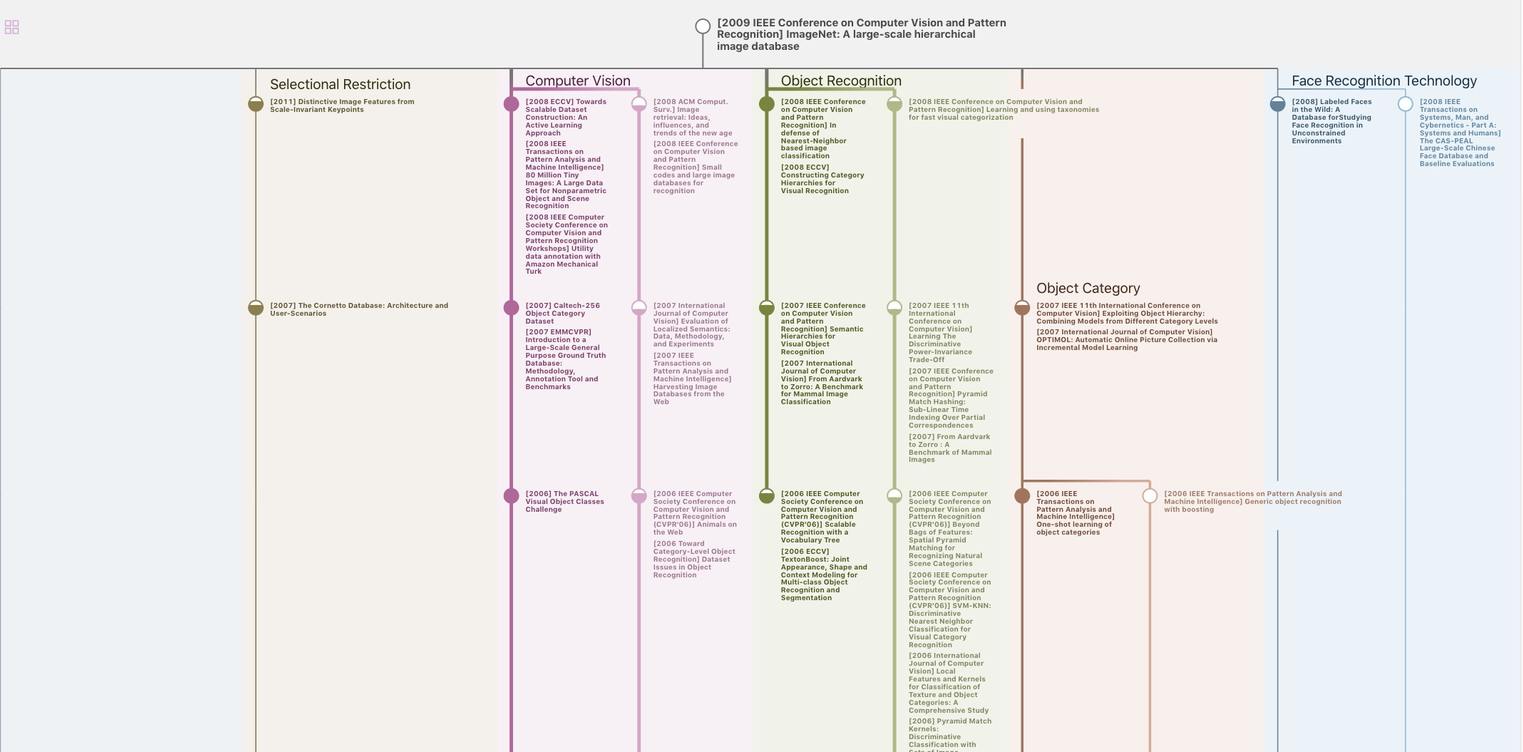
生成溯源树,研究论文发展脉络
Chat Paper
正在生成论文摘要