Solving Inverse Problems in Compressive Imaging with Score-Based Generative Models
2023 IEEE 10th International Conference on Data Science and Advanced Analytics (DSAA)(2023)
摘要
Snapshot Compressive Imaging (SCI) is a technique for capturing high-dimensional data through snapshot measurements using a two-dimensional (2D) detector. This approach is accomplished via coded aperture compressive temporal imaging (CACTI), which involves applying a temporally variant mask to spatially encode each sequential signal before aggregating the encoded information into a single compressed measurement. The objective of our work is to develop algorithms capable of reconstructing each video frame as a 3D data cube from its 2D measurement. To achieve this goal, we introduce multiple approaches that utilize unconditional and pre-trained 2D score models for video frame reconstruction. Our method involves modeling both the forward perturbation process of the data distribution and its reverse process as stochastic differential equations (SDEs). We also employ score-based deep learning models to estimate the scores of the data distribution across different time steps. Differing from many applications, our sampling process relies on the observed measurement, which directly corresponds to pixel values, rather than class labels. We demonstrate that employing traditional score-based generative methods with 2D score models in SCI, or integrating them into the plug-and-play framework as a deep generative prior, presents challenges. Furthermore, we propose ideas to address these limitations for future research.
更多查看译文
关键词
Snapshot Compressive Imaging,Compressive Imaging,Computational Imaging,Inverse Problem,Generative Models
AI 理解论文
溯源树
样例
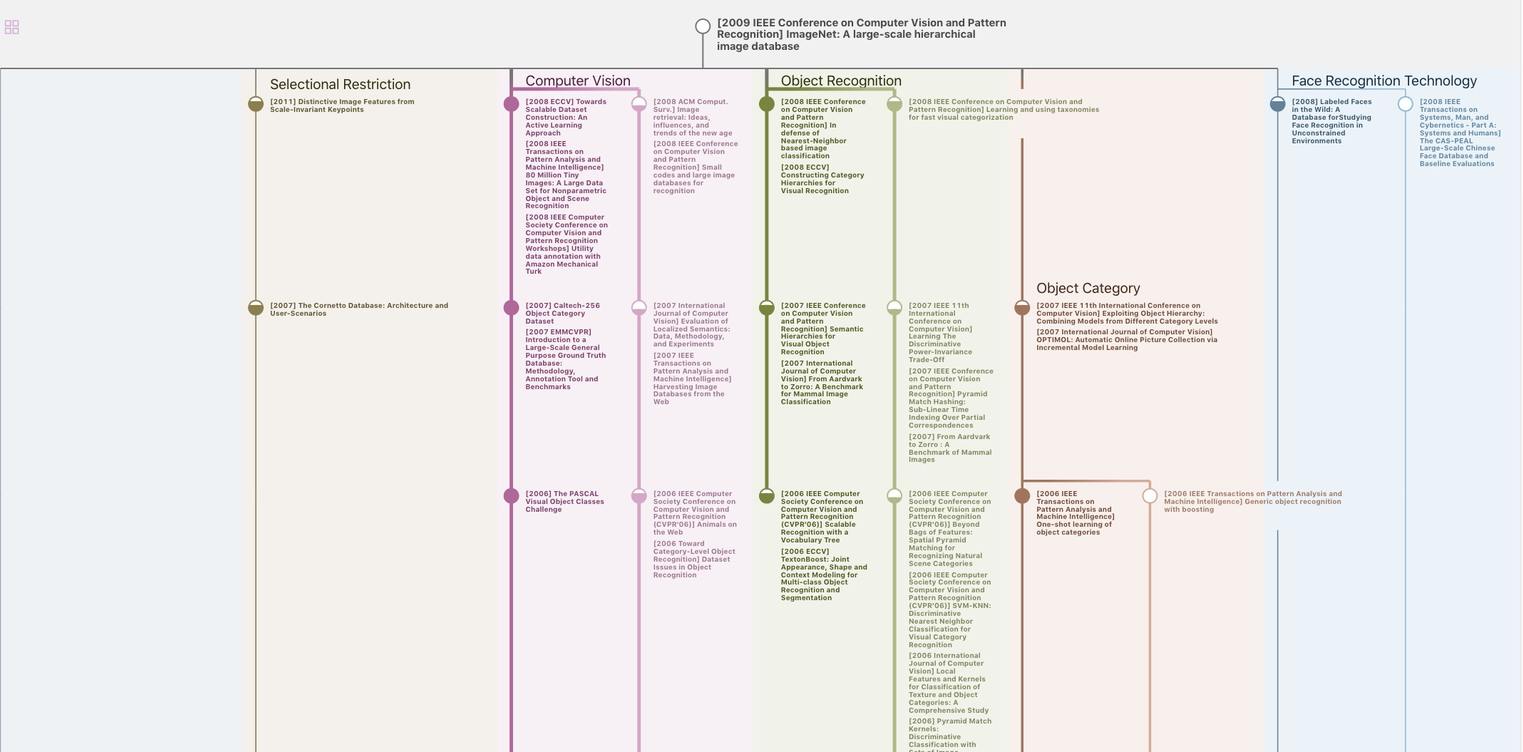
生成溯源树,研究论文发展脉络
Chat Paper
正在生成论文摘要