Unsupervised Contrastive Learning-Based Adversarial Domain Adaptation
2023 15th International Conference on Communication Software and Networks (ICCSN)(2023)
摘要
According to recent research, applying deep domain adaptation techniques has yielded promising results in effectively addressing cross-domain hyperspectral image (HSI) classification challenges. However, most current deep domain adaptation methods for HSI classification solely focus on either the spectral or spatial characteristics of hyperspectral data, and fail to consider their synergistic impact. Moreover, numerous existing adversarial domain adaptation methods only consider the transferability of the model to the data, without considering the separability and discriminability of the model to the target domain samples. To address the above issues, we propose an unsupervised contrastive learning-based adversarial domain adaptation (UCLADA) architecture. Firstly, we utilize a spectral-spatial feature extraction network (SSFEN) and a discriminator to achieve adversarial domain adaptation. Secondly, an unsupervised contrastive learning method is implemented in the target domain to increase the separability of the model towards the target samples. Finally, a trusted sample selection strategy is proposed, which further improves the discriminability of the model against target samples by fine-tuning the model using trusted sample labels. Experiments have shown that our method outperforms other existing methods in cross-domain HSI classification tasks.
更多查看译文
关键词
classification,unsupervised contrastive learning,domain adaptation,hyperspectral image (HSI)
AI 理解论文
溯源树
样例
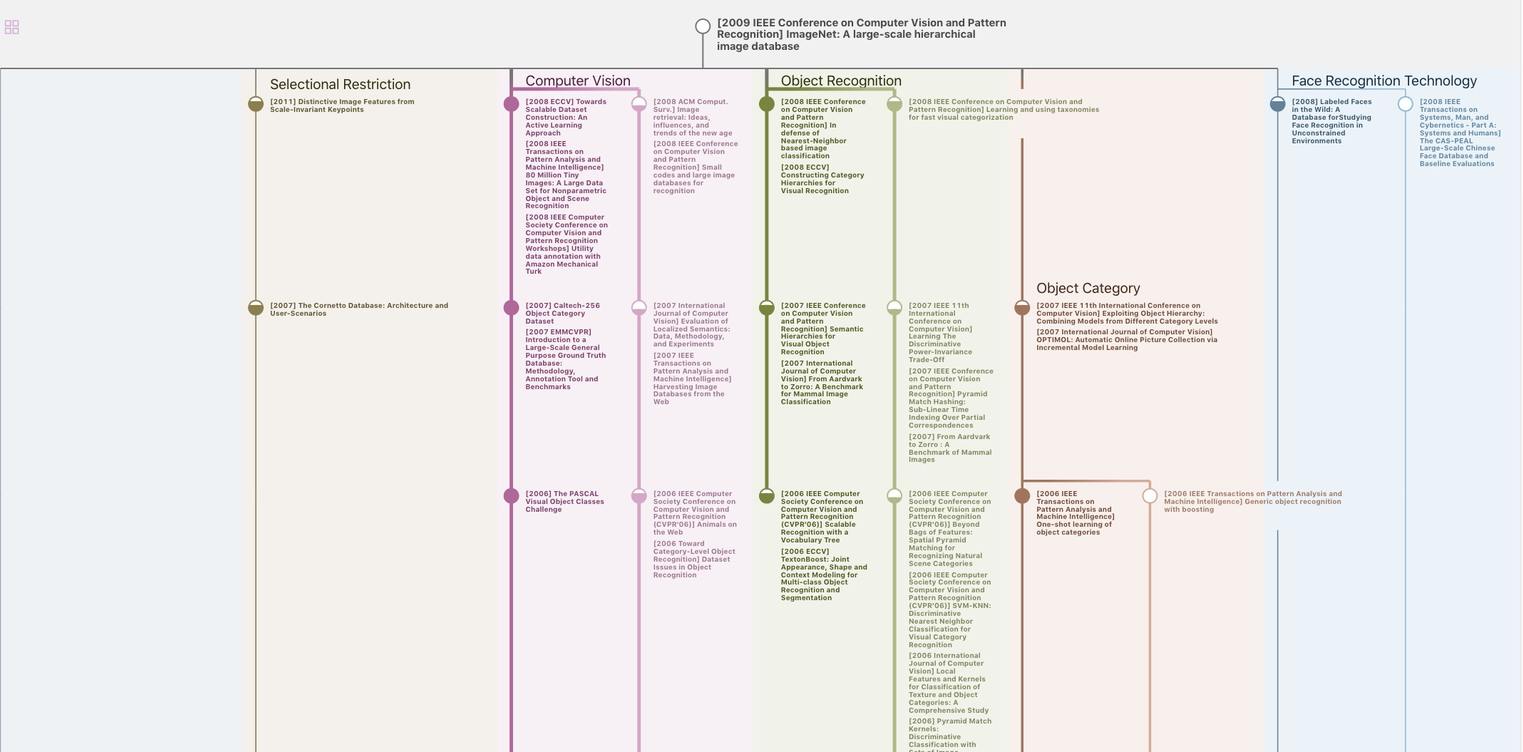
生成溯源树,研究论文发展脉络
Chat Paper
正在生成论文摘要