Feature Selection Methods for Effective Diagnosis of Rotavirus Infection: A Comparative Evaluation
2023 15th International Conference on Knowledge and Systems Engineering (KSE)(2023)
摘要
Rotavirus causes severe diarrhea in children worldwide. Correct diagnosis is essential to manage the condition, especially in resource-poor areas effectively. This study analyses Rotavirus feature selection strategies to help detect and cure the infection early. The study found that wrapper and embedding approaches outperform filter methods, notably for the Logistic Regression (LR) and Support Vector Machine (SVM) models, employing two machine learning models and the AUC measure. Rotavirus infection can be diagnosed early by considering age, season, blood stool, and blood signs. The report acknowledges data and categorization constraints. Future research endeavors will focus on exploring additional methods and enhancing diagnostic accuracy. This research contributes to advancing feature selection techniques in the domain of Rotavirus diagnosis, addressing the pressing need for improved diagnostic capabilities in infectious diseases.
更多查看译文
关键词
Rotavirus infection,Clinical diagnosis,Machine learning,Feature selection,Filter,Wrapper,Embedded
AI 理解论文
溯源树
样例
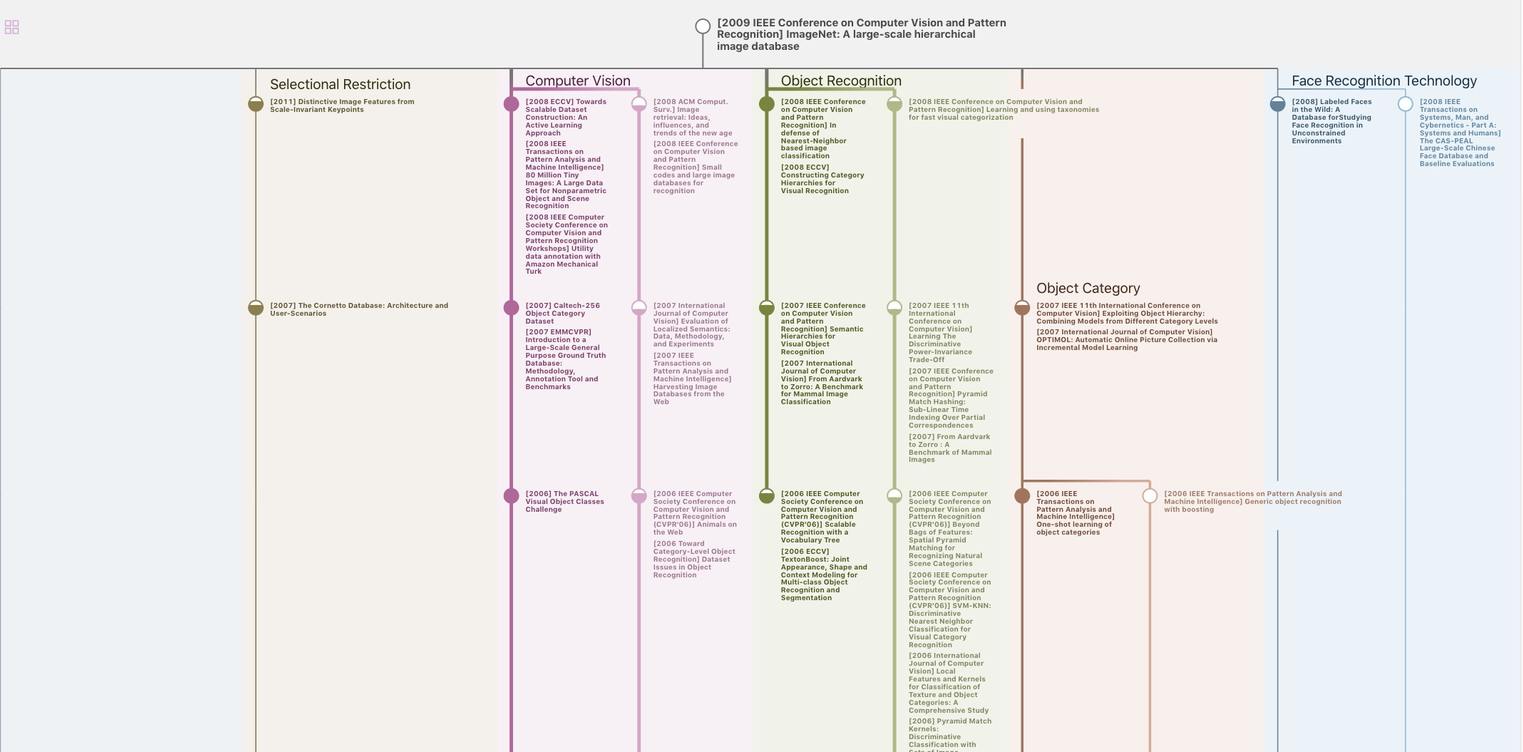
生成溯源树,研究论文发展脉络
Chat Paper
正在生成论文摘要