An accelerated exact distributed first-order algorithm for optimization over directed networks.
J. Frankl. Inst.(2023)
摘要
Distributed optimization over networked agents has emerged as an advanced paradigm to address large-scale control, optimization, and signal-processing problems. In the last few years, the distributed first-order gradient methods have witnessed significant progress and enrichment due to the simplicity of using only the first derivatives of local functions. An exact first-order algorithm is developed in this work for distributed optimization over general directed networks with only row-stochastic weighted matrices. It employs the rescaling gradient method to address unbalanced information diffusion among agents, where the weights on the received information can be arbitrarily assigned. Moreover, uncoordinated step sizes are employed to magnify the autonomy of agents, and an error compensation term and a heavy-ball momentum are incorporated to accelerate convergency. A linear convergence rate is rigorously proven for strongly-convex objective functions with Lipschitz continuous gradients. Explicit upper bounds of step-size and momentum parameter are provided. Finally, simulations illustrate the performance of the proposed algorithm. & COPY; 2023 The Franklin Institute. Published by Elsevier Inc. All rights reserved.
更多查看译文
关键词
optimization,algorithm,networks,accelerated exact,first-order
AI 理解论文
溯源树
样例
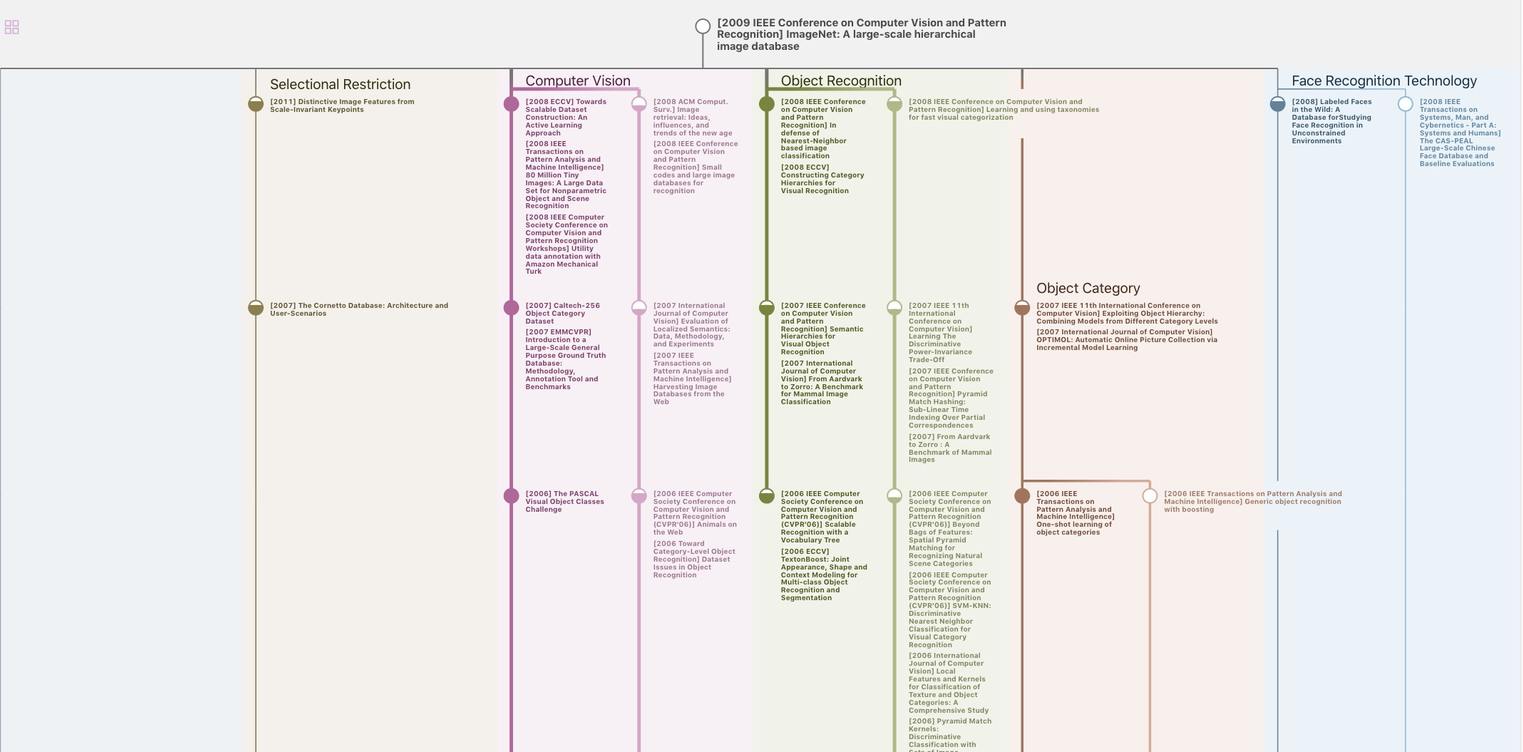
生成溯源树,研究论文发展脉络
Chat Paper
正在生成论文摘要