Oversampling method based on GAN for tabular binary classification problems
Intell. Data Anal.(2023)
摘要
Data-imbalanced problems are present in many applications. A big gap in the number of samples in different classes induces classifiers to skew to the majority class and thus diminish the performance of learning and quality of obtained results. Most data level imbalanced learning approaches generate new samples only using the information associated with the minority samples through linearly generating or data distribution fitting. Different from these algorithms, we propose a novel oversampling method based on generative adversarial networks (GANs), named OS-GAN. In this method, GAN is assigned to learn the distribution characteristics of the minority class from some selected majority samples but not random noise. As a result, samples released by the trained generator carry information of both majority and minority classes. Furthermore, the central regularization makes the distribution of all synthetic samples not restricted to the domain of the minority class, which can improve the generalization of learning models or algorithms. Experimental results reported on 14 datasets and one high-dimensional dataset show that OS-GAN outperforms 14 commonly used resampling techniques in terms of G-mean, accuracy and F1-score.
更多查看译文
关键词
Oversampling,GAN,imbalanced learning
AI 理解论文
溯源树
样例
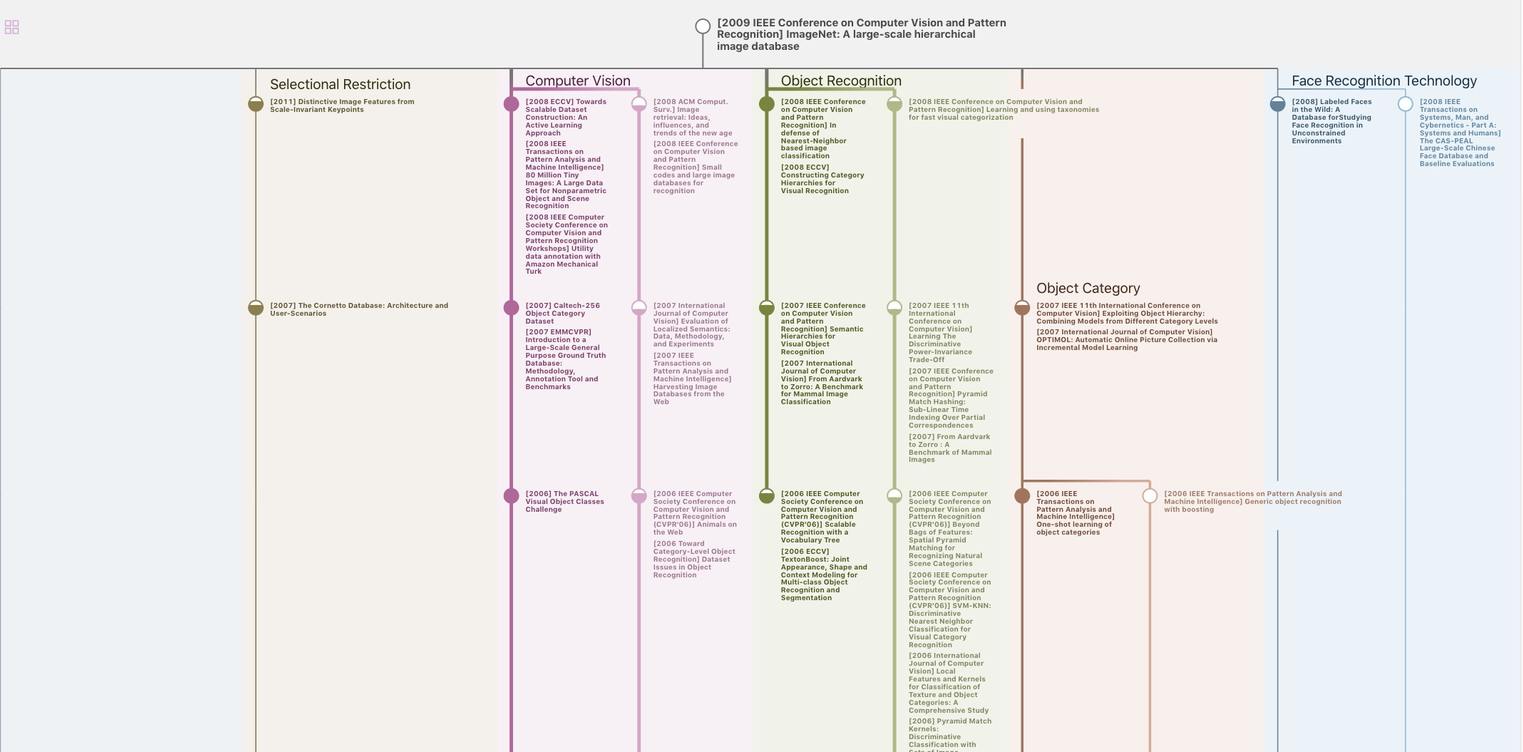
生成溯源树,研究论文发展脉络
Chat Paper
正在生成论文摘要