A framework of regularized low-rank matrix models for regression and classification
Statistics and Computing(2023)
摘要
While matrix-covariate regression models have been studied in many existing works, classical statistical and computational methods for the analysis of the regression coefficient estimation are highly affected by high dimensional matrix-valued covariates. To address these issues, this paper proposes a framework of matrix-covariate regression models based on a low-rank constraint and an additional regularization term for structured signals, with considerations of models of both continuous and binary responses. We propose an efficient Riemannian-steepest-descent algorithm for regression coefficient estimation. We prove that the consistency of the proposed estimator is in the order of O(√(r(q+m)+p)/√(n)) , where r is the rank, p× m is the dimension of the coefficient matrix and p is the dimension of the coefficient vector. When the rank r is small, this rate improves over O(√(qm+p)/√(n)) , the consistency of the existing work (Li et al. in Electron J Stat 15:1909-1950, 2021) that does not apply a rank constraint. In addition, we prove that all accumulation points of the iterates have similar estimation errors asymptotically and substantially attaining the minimax rate. We validate the proposed method through a simulated dataset on two-dimensional shape images and two real datasets of brain signals and microscopic leucorrhea images.
更多查看译文
关键词
Electroencephalography (EEG),Generalized linear model (GLM),High dimensionality,norm,Microscopic leucorrhea images,Rank constraint,Riemannian steepest descent,Sparsity
AI 理解论文
溯源树
样例
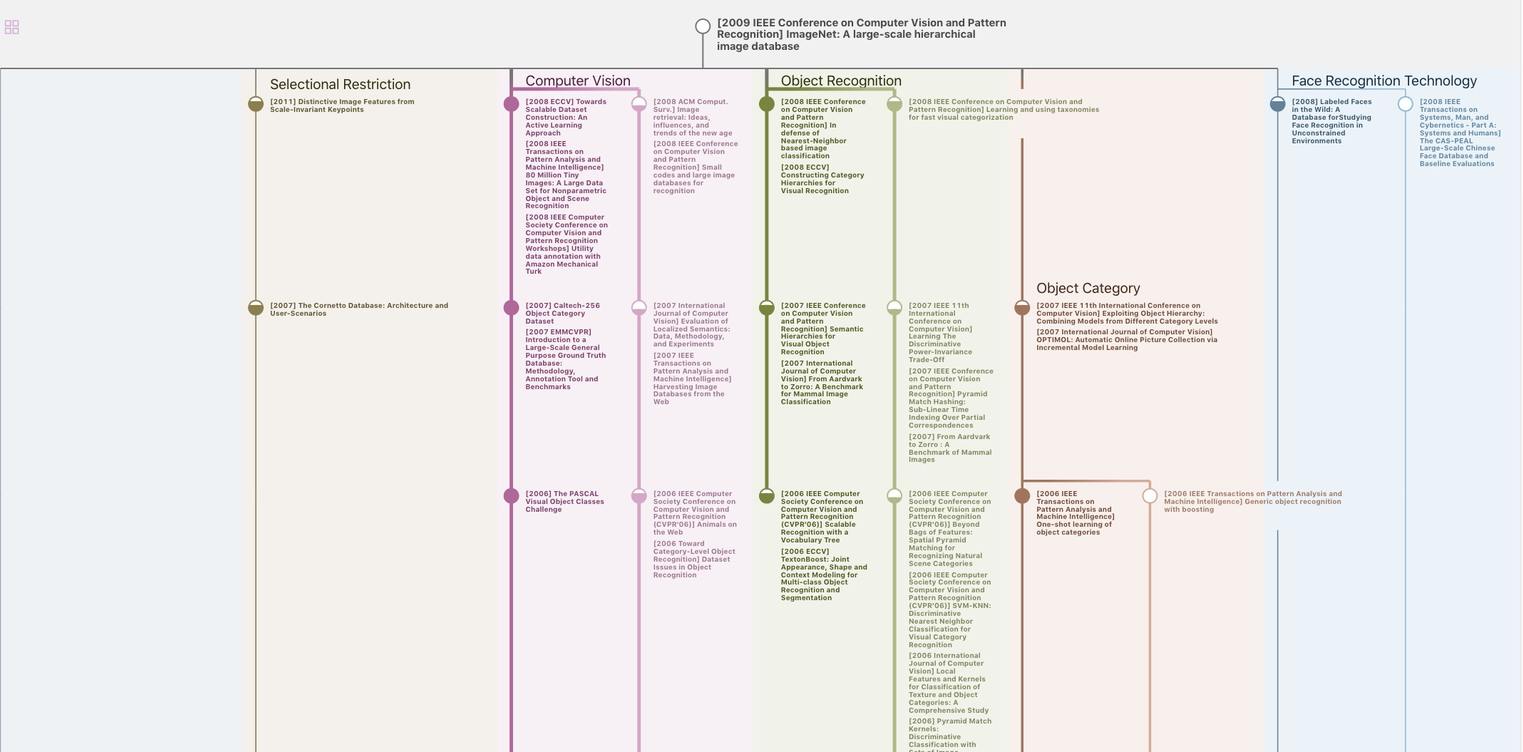
生成溯源树,研究论文发展脉络
Chat Paper
正在生成论文摘要