Measuring dense false positive regions from segmentation result for whole slide tissue histology image
J. Vis. Commun. Image Represent.(2023)
摘要
Evaluating a segmentation model is critical for constructing computer-aided diagnosis (CAD) systems, in which the segmented regions will be used for downstream analysis. However, existing segmentation evaluation metrics may not reflect every aspect of a trained segmentation model performance in the context of the whole slide tissue histology images (WSIs). Specifically, existing segmentation metrics generally ignore the impact of densely packed false positive pixels (DFP) in the WSI segmentation result. This study presents a new and efficient metric, named MAFaR, accounting for DFP regions in digital WSI segmentation. The proposed metric consists of two modules: 1) Estimation of DFP regions; 2) Calculation of MAFaR score. In module 1, a Gaussian Kernel Density Estimation method was used to estimate the density of the false positive (FP) regions in segmentation result (SR). Then a two-step Mean-shift clustering algorithm was applied to the high-density FP regions to estimate the DFP regions. In module 2, the ratio of DFP regions area to the positive regions area were used for MAFaR calculation. We conducted two experiments to evaluate the effectiveness of the MAFaR score. In the first experiment, we compared MAFaR with existing metrics related to FP regions, the proposed MAFaR score can reflect the impact of DFP regions. In the second experiment, MAFaR scores were compared with the manual evaluation scores given by three experienced engineers and found high correlation (Spearman’s rank correlation coefficients greater than 0.7) and high agreement (Kendall coefficient = 0.839). Therefore, the MAFaR can be used with other segmentation metrics for evaluating WSI segmentation.
更多查看译文
关键词
Image segmentation,Evaluation metric,Histology image
AI 理解论文
溯源树
样例
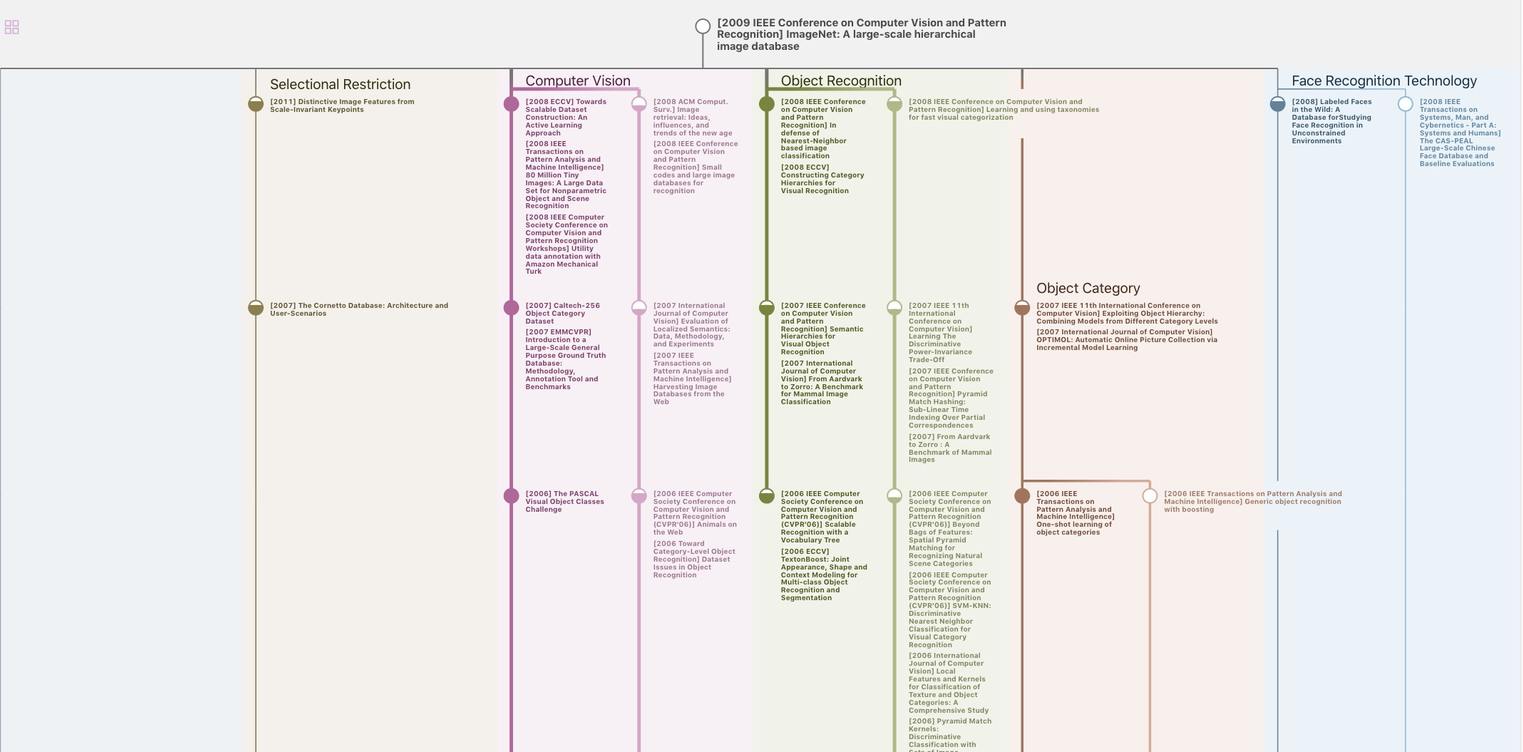
生成溯源树,研究论文发展脉络
Chat Paper
正在生成论文摘要