Class-Specific Autoaugment Architecture Based on Schmidt Mathematical Theory for Imbalanced Hyperspectral Classification.
IEEE Trans. Geosci. Remote. Sens.(2023)
摘要
Hyperspectral image classification (HSIC) often suffers from severe imbalanced category distribution in real applications, which causes bias toward the dominated categories. As an effective method, the deep generative model (DGM) can be used to augment the features of imbalanced data through a learnable method to achieve superior classification performance. However, the features extracted by DGM are preset as a standard Gaussian distribution which results in low interclass difference. Besides, the generated features are too consistent with the original ones, which cannot play a positive role in the discriminability of minority categories (MCs). To conquer these drawbacks, we propose a class-specific autoaugment architecture based on Schmidt mathematical theory (CACS) for the challenging of imbalanced data which consists of two stages: one is training a superior features extractor, and the other one is augmenting features. The class-specific features of the whole HSI are extracted in stage one that supports the following feature augmented module. Specifically, we weighted the classifier in the first phase according to cost-sensitive learning, to prevent the classifier from overfitting. To expand the dispersion between categories, we construct feature prototypes obeying different Gaussian distributions for each class, respectively, and generate class-specific features. Then, the features are augmented in the second phase based on Schmidt’s mathematical theory, which enhances the discriminability of minority class features, thus further improving the classification accuracy with interpretability. Extensive experimental results on three benchmarking datasets demonstrate that CACS is outstanding in comparison algorithms, especially in MCs.
更多查看译文
关键词
Imbalanced data,interclass difference,Schmidt mathematical theory,weight balance
AI 理解论文
溯源树
样例
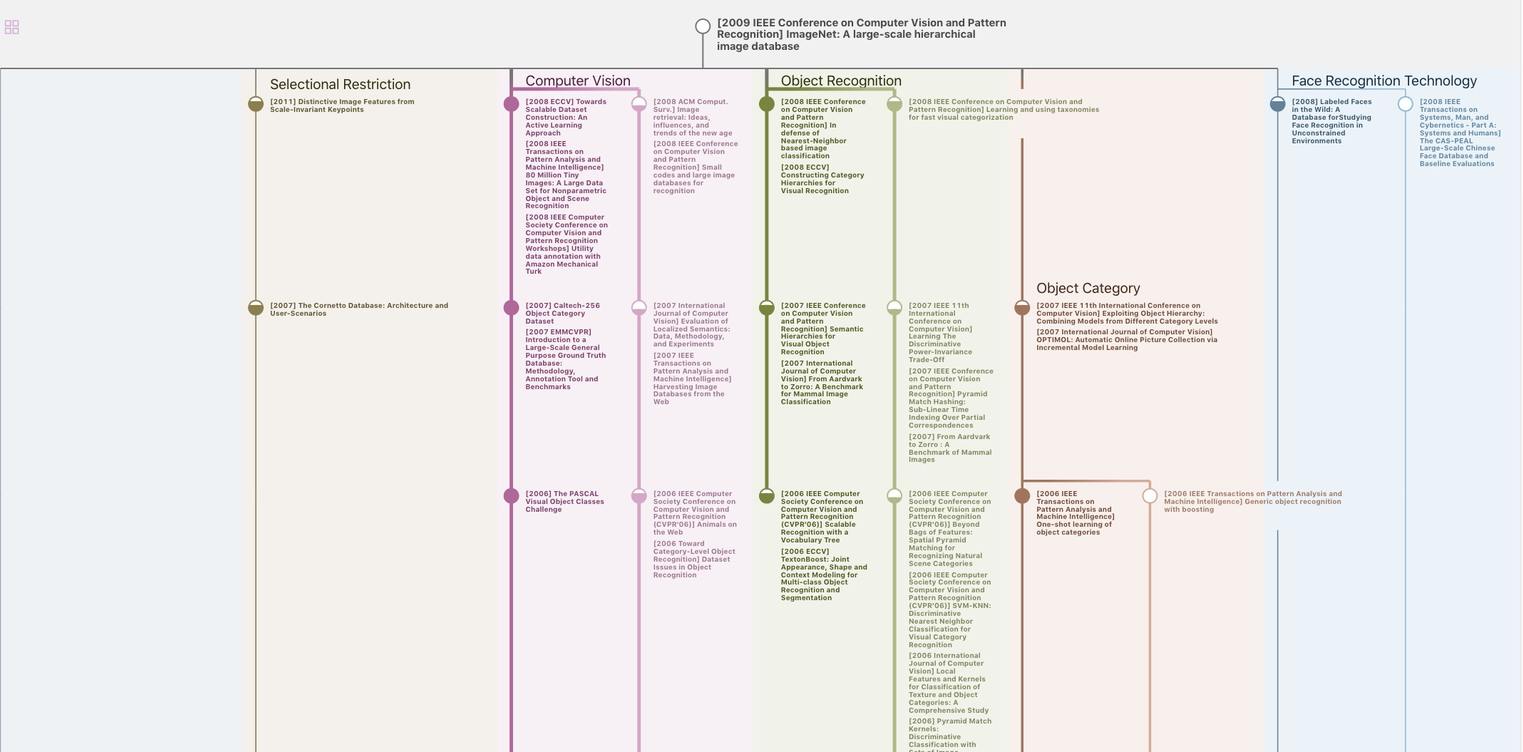
生成溯源树,研究论文发展脉络
Chat Paper
正在生成论文摘要