Hyperspectral Denoising via Global Variation and Local Structure Low-Rank Model.
IEEE Trans. Geosci. Remote. Sens.(2023)
摘要
Hyperspectral images (HSIs) are often disturbed by various kinds of noises. This article proposes a global variation and local structure low-rank model (GLLR) for HSI denoising by integrating spatial segmentation smoothing and spectral low-rank (LR) properties. Compared with existing denoising methods, the proposed method considers not only the global LR property but also the local structure LR property of HSIs. Specifically, the GLLR describes the global correlation and segmental smoothing structure of the HSI by the correlated total variation (CTV). In addition, we construct a new structural LR prior, called the local minimum difference (LMD) LR. With LMD LR property of HSI, GLLR can remove noise while retaining useful structural information in the HSI. Then, an augmented lagrange multiplier (ALM)-based optimization algorithm is devised to solve the objective functions for the presented model. Finally, comparison experiments with existing methods are conducted on synthetic and real datasets to demonstrate the effectiveness and superiority of the proposed method.
更多查看译文
关键词
Alternating iterative optimization, denoise, hyperspectral image (HSI), local structure, low-rank (LR)
AI 理解论文
溯源树
样例
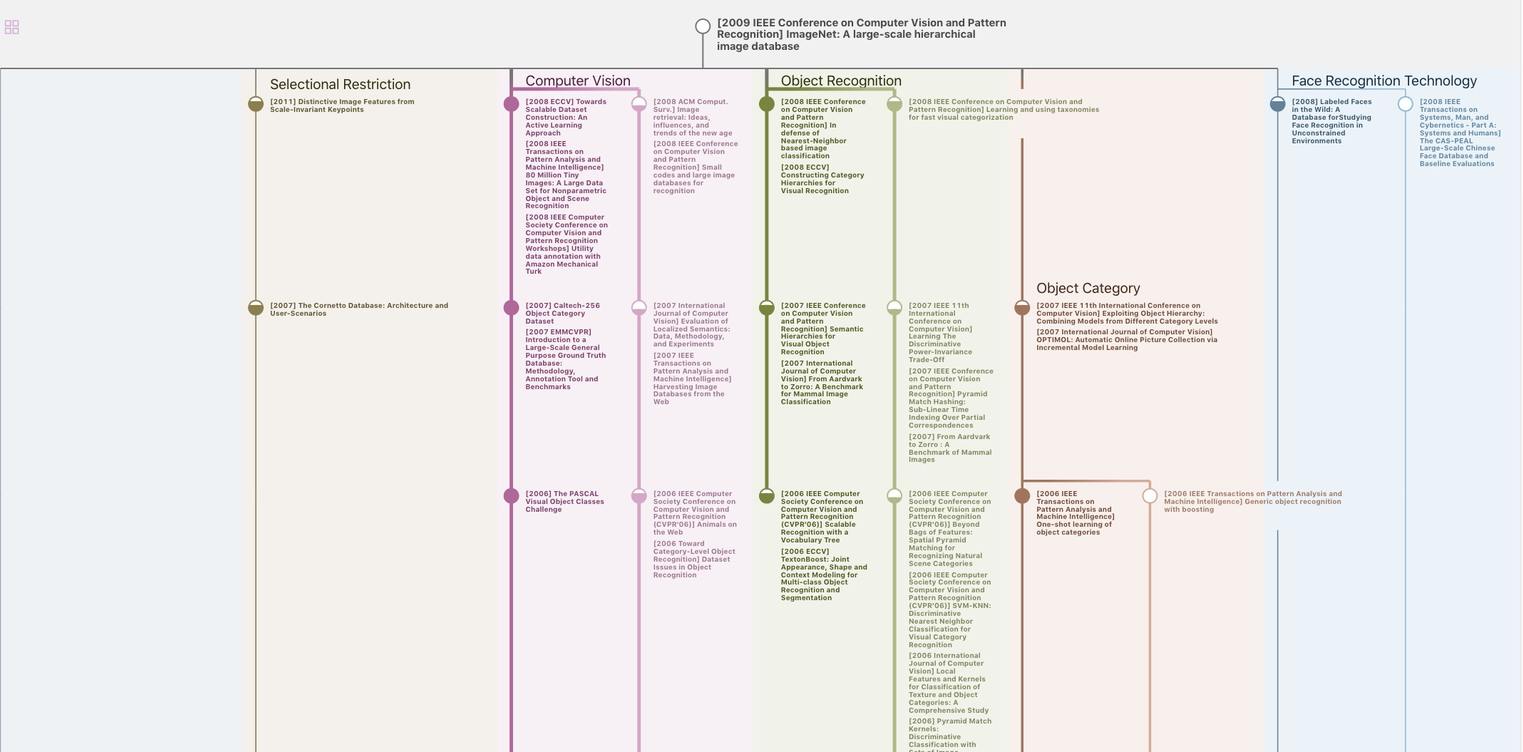
生成溯源树,研究论文发展脉络
Chat Paper
正在生成论文摘要