Temporal Chain Network With Intuitive Attention Mechanism for Long-Term Series Forecasting.
IEEE Trans. Instrum. Meas.(2023)
摘要
Long-term series forecasting (LTSF) plays an important role in real-world applications in the economy, the weather, and the industrial process. At present, many transformer-based methods have made promising progress. However, the nature of the permutation-invariant self-attention mechanism inevitably results in temporal information loss, which hinders the prediction performance of the transformer-based LTSF methods. Therefore, this article proposed a novel temporal chain network (TCNet) with an intuitive attention mechanism for LTSF. Based on the chain forward propagation structure of time series, a one-way chain graph neural network (GNN) is constructed to avoid the permutation-invariance of the self-attention mechanism. Meanwhile, based on the natural forgetting mechanism of time series, the prior intuitive attention is proposed as the edge weight (attention) for information propagation and then the series model is obtained by the GNN. Furthermore, the proposed method achieves the LTSF task by linear forecasting of the trend component and nonlinear forecasting of the seasonal component of the series. Extensive experiments on five benchmarks and a real-world chemical process dataset are conducted to demonstrate the effectiveness of the TCNet. Comparison experiment results show that the TCNet achieves state-of-the-art results compared to current baselines and reduces average prediction error by 9.52% and 5.97% on transformer-based and nontransformer-based multivariate LTSF baselines, respectively. Moreover, the temporal information loss for the TCNet due to the permutation-invariance of the self-attention mechanism is not the main reason hindering transformer-based LTSF methods, whose bottleneck comes mainly from the complex architecture of the decoder.
更多查看译文
关键词
temporal chain network,forecasting,intuitive attention mechanism,series,long-term
AI 理解论文
溯源树
样例
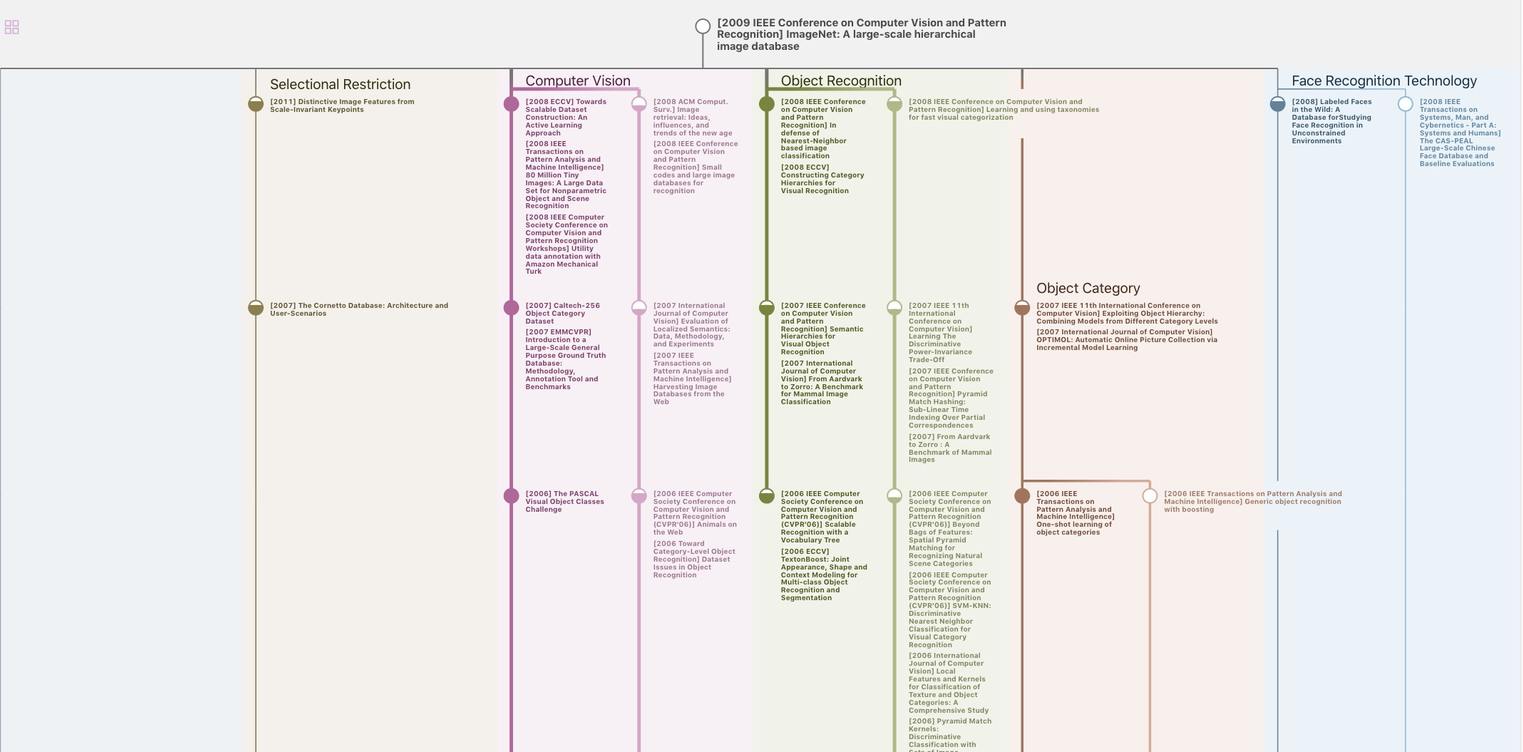
生成溯源树,研究论文发展脉络
Chat Paper
正在生成论文摘要