An Interpretable Dynamic Inference System Based on Fuzzy Broad Learning
IEEE Trans. Instrum. Meas.(2023)
摘要
Fuzzy broad learning system (FBLS) is a fuzzy neural model proposed in recent years, which combines the efficient performance of broad learning and the interpretability of fuzzy systems. However, the current research on the interpretability of fuzzy broad learning is only limited to the partial network layer, which hinders the model’s ability to express rules and extract knowledge. A Takagi–Sugeno–Kang (TSK) fuzzy inference system (FIS) with strong expressive power and adaptive network structure is explored and developed under the fuzzy broad learning framework in this article. It improves the accuracy of neural fuzzy models and addresses the challenge of poor interpretability in fuzzy broad learning. First, to build an expressive power model, a set of TSK fuzzy system outputs is used as a mapped layer, and interpretable linguistic fuzzy rules (ILFRs) are embedded in the enhancement layer. Second, an incremental learning method is proposed to allow the parameters to be adaptively adjusted and the network structure to be optimized for the rule explosion problem and network structure redundancy. Finally, the Schur complement method is used instead of the ridge regression method to find the pseudo-inverse to improve the model learning efficiency. The experiments show that the proposed model has a compact structure and good classifiably while having interpretability.
更多查看译文
关键词
Dynamic inference system,expressive power,fuzzy broad networks,Takagi–Sugeno–Kang (TSK) fuzzy system
AI 理解论文
溯源树
样例
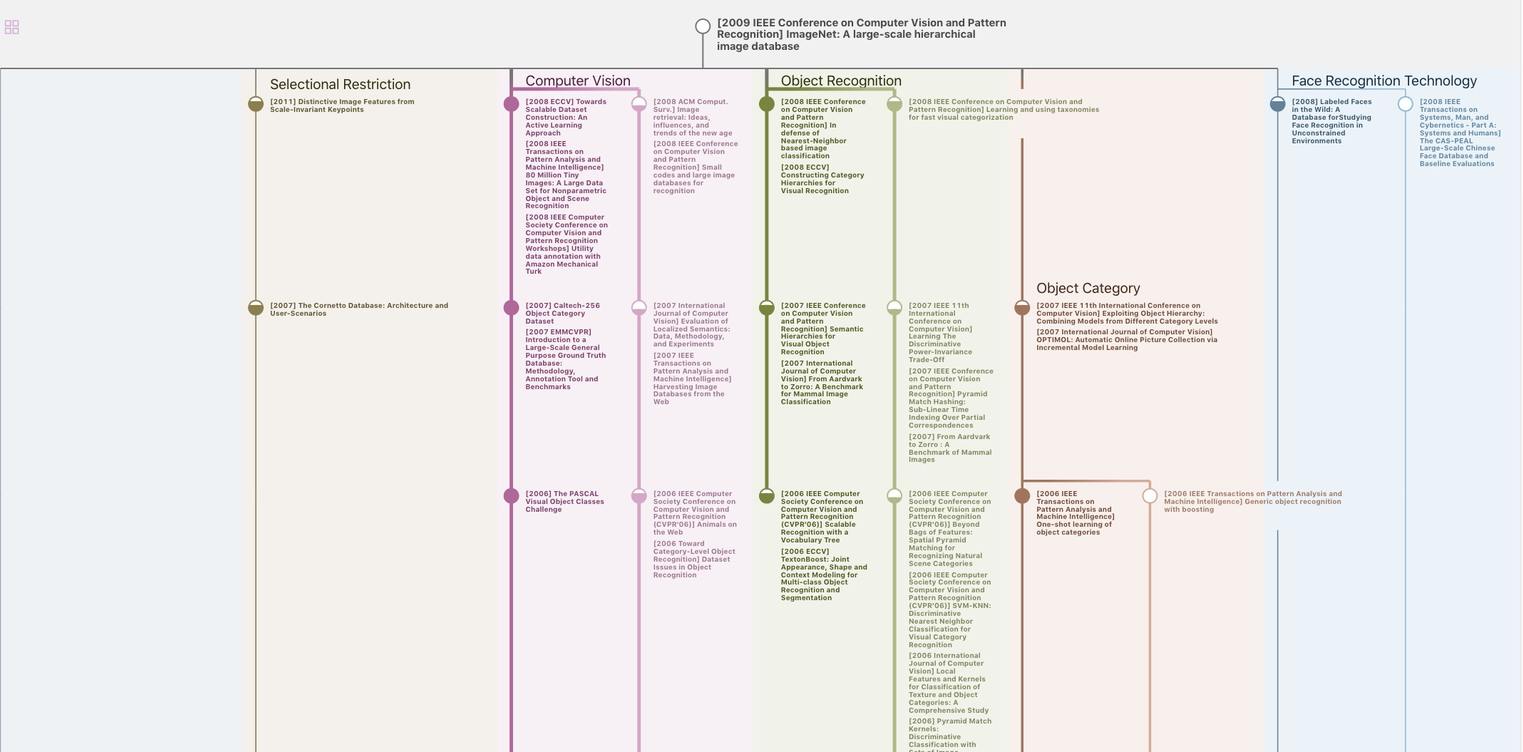
生成溯源树,研究论文发展脉络
Chat Paper
正在生成论文摘要