MSAIF-Net: A Multistage Spatial Attention-Based Invertible Fusion Network for MR Images.
IEEE Trans. Instrum. Meas.(2023)
摘要
In recent years, multimodal medical image fusion has drawn increasing attention, aiming to provide comprehensive information for image understanding and clinical applications. With the development of deep-learning-based models, great success has been achieved in multimodal image fusion. However, most current models still suffer from limited performance and feature loss during fusion. The lack of consensus on evaluation criteria further compromises their clinical values. In this article, to deal with the aforementioned concerns, a novel multistage spatial attention-based invertible fusion network (MSAIF-Net) is proposed for multimodal magnetic resonance (MR) images with improved feature learning and fusion ability. More specifically, it introduces the feature extraction module (FEM) to exploit the foreground and background of feature maps and proposes a multiattention module to aggregate features among different stages. Moreover, an invertible neural network (INN) is designed for feature fusion, so as to better preserve the complementary information of different modalities. In addition to comparing their unsupervised evaluation metrics, a high-level task, the segmentation task, is adopted to test the superiority of fused images. Extensive experiments on different datasets validate the effectiveness and generalization of our proposed method compared with other state-of-the-art (SOTA) image fusion approaches in both supervised and unsupervised metrics.
更多查看译文
关键词
Invertible neural network (INN),magnetic resonance imaging (MRI),medical image fusion,medical image segmentation,spatial attention
AI 理解论文
溯源树
样例
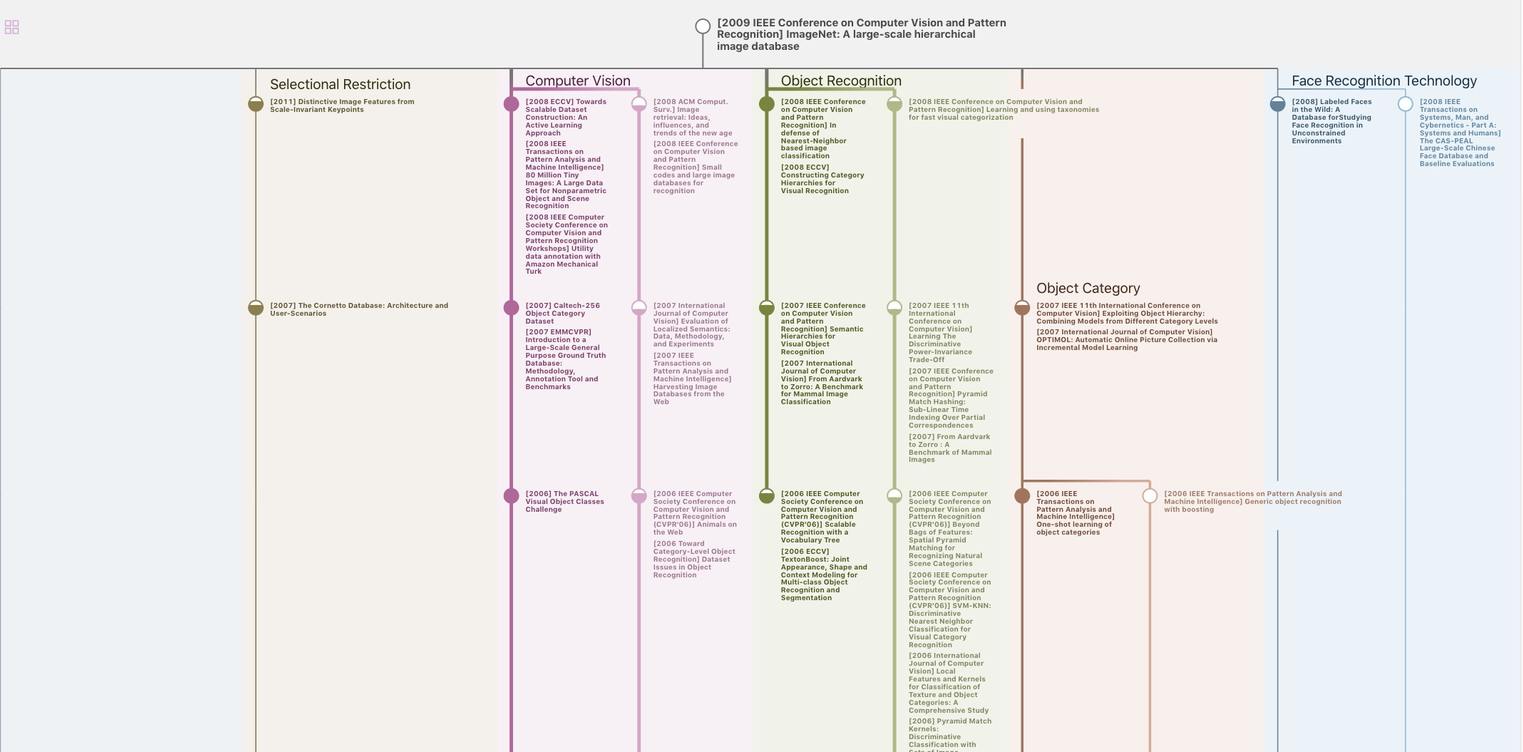
生成溯源树,研究论文发展脉络
Chat Paper
正在生成论文摘要