Compressive Detection of Stochastic Sparse Signals With Unknown Sparsity Degree.
IEEE Signal Process. Lett.(2023)
摘要
In this letter, we investigate the problem of detecting compressed stochastic sparse signals with unknown sparsity degree under Bernoulli–Gaussian model. In addition to the generalized likelihood ratio test (GLRT) proposed in (Hariri and Babaie–Zadeh et al., 2017), the corresponding Rao test and Wald test are derived in this letter. By observing that obtaining their analytical performance is challenging, we further propose a new probability constraint estimator (PCE) of the unknown sparsity degree. Interestingly, by adopting the PCE, the GLRT, Rao and Wald tests are shown to be statistically equivalent and reduce to a new detector (i.e., the detector with PCE) with a simple structure. The analytical performance of the detector with PCE is thus derived, which is verified by Monte Carlo simulations. Finally, numerical experiments illustrate that the proposed Rao test and the detector with PCE outperform the original GLRT.
更多查看译文
关键词
Bernoulli–Gaussian model,compressive detection,Rao test,stochastic sparse signals,Wald test
AI 理解论文
溯源树
样例
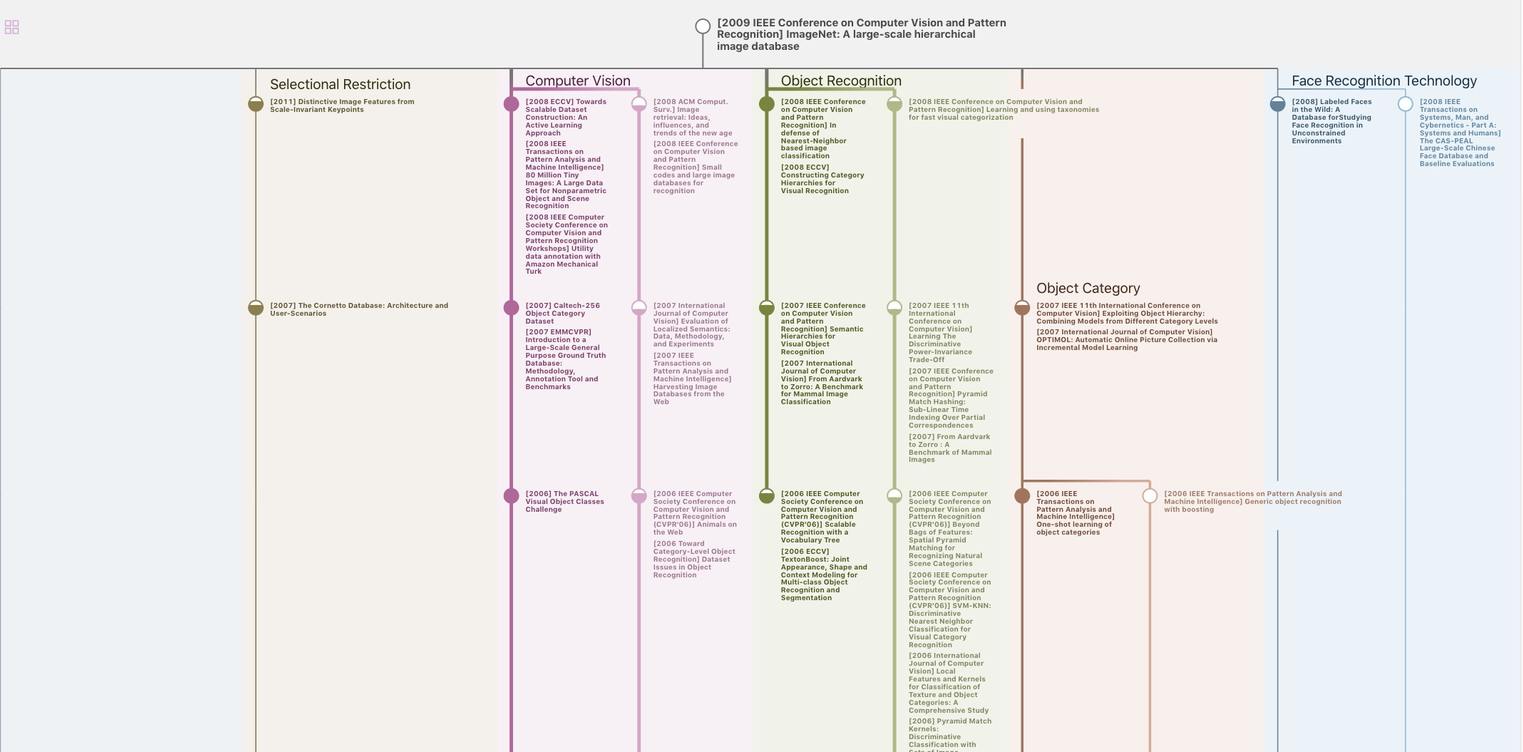
生成溯源树,研究论文发展脉络
Chat Paper
正在生成论文摘要