Image Quality Assessment of Diabetic Retinopathy Based on ADD-Net
IEEE Access(2023)
摘要
Retinal image quality assessment (RIQA) is a precondition for diabetic retinopathy screening and diagnosis. Current RIQA network tend to place too much emphasis on performance improvement, ignoring the number of parameters and computational complexity of the network, and the existing publicly available datasets with unclear classification criteria and unbalanced number of different kinds of images, which affect the training of deep neural networks and large area screening of diabetic retinopathy. To solve these problems. A new quality criteria for retinal images is developed, and the Kaggle retinal image quality dataset is re-labeled and reclassified according to the criteria. And a high-performance lightweight RIQA network ADD-Net is proposed based on the DenseNet, which increases the perceptual field and improves pixel utilization by stacking dilated convolutions in DenseLayer, and incorporates attention mechanisms to further optimize the network performance. The proposed ADD-Net has a parametric number of 8.92M, a computational complexity of 2.76GMac, an accuracy of 97.03%, a precision of 97.06%, and a sensitivity of 98.31%, which are better than those of current mainstream methods. A quality criteria for retinal images that combines image quality features, retinal structural features, and DR lesion features was developed. The RIQA network ADD-Net with low number of parameters, less computational complexity and excellent performance is designed.
更多查看译文
关键词
Attention mechanism,deep learning,dilated convolution,DenseNet,retinal image quality assessment (RIQA)
AI 理解论文
溯源树
样例
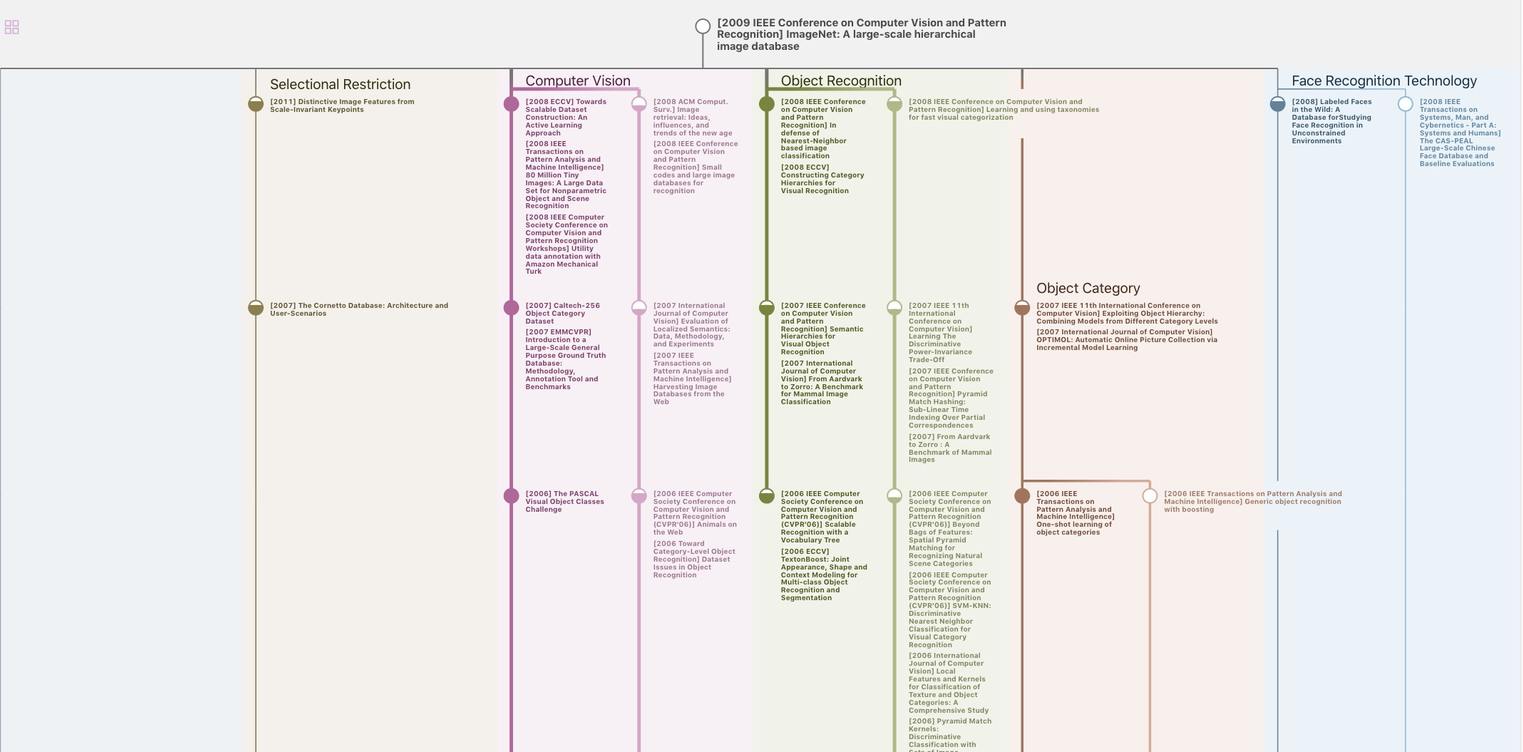
生成溯源树,研究论文发展脉络
Chat Paper
正在生成论文摘要