Enhancement method for non-uniform scattering images of turbid underwater based on neural network
Image Vis. Comput.(2023)
摘要
Due to the influence of light absorption and scattering in the water medium, underwater images often appear with different degrees of blur, color distortion, and so on. The physical model-based method needs to establish image degradation processes and improve image quality by assuming and estimating complex parameters, which limits the generalization ability and practical application effect of these methods. To solve the above problems, this paper proposes an image enhancement method of non-uniform scattering in turbid underwater based on neural network. First, the high-frequency and low-frequency components of underwater images were obtained through the difference of gaussian (DOG) and bilateral filtering (BF), respectively. Then, for the high-frequency component, pulse coupled neural networks (PCNN) to improve the edge details of the image. For low-frequency components, it is carried out in two steps: an adaptive automatic white balance and polynomial regression (AAWBPR) algorithm is proposed, which can effectively eliminate the color bias under different color temperatures and improve the brightness of images; the adaptive color and contrast enhancement (ACCE) method is used to further enhance the color and contrast of images. Finally, Decom-Net and the improved U-Net method are used to reduce the errors caused by the non-uniform scattering effect, weaken the noise interference, and enhance the overall effect of images. Extensive experiments show that the proposed method can effectively improve the quality of turbid underwater non-uniform scattering images, enhance the contrast of images, reduce the complexity of parameters, and has good generalization performance and practical application effects.
更多查看译文
关键词
Visual quality enhancement,Underwater optical image,Background light,Convolutional neural network,Image enhancement
AI 理解论文
溯源树
样例
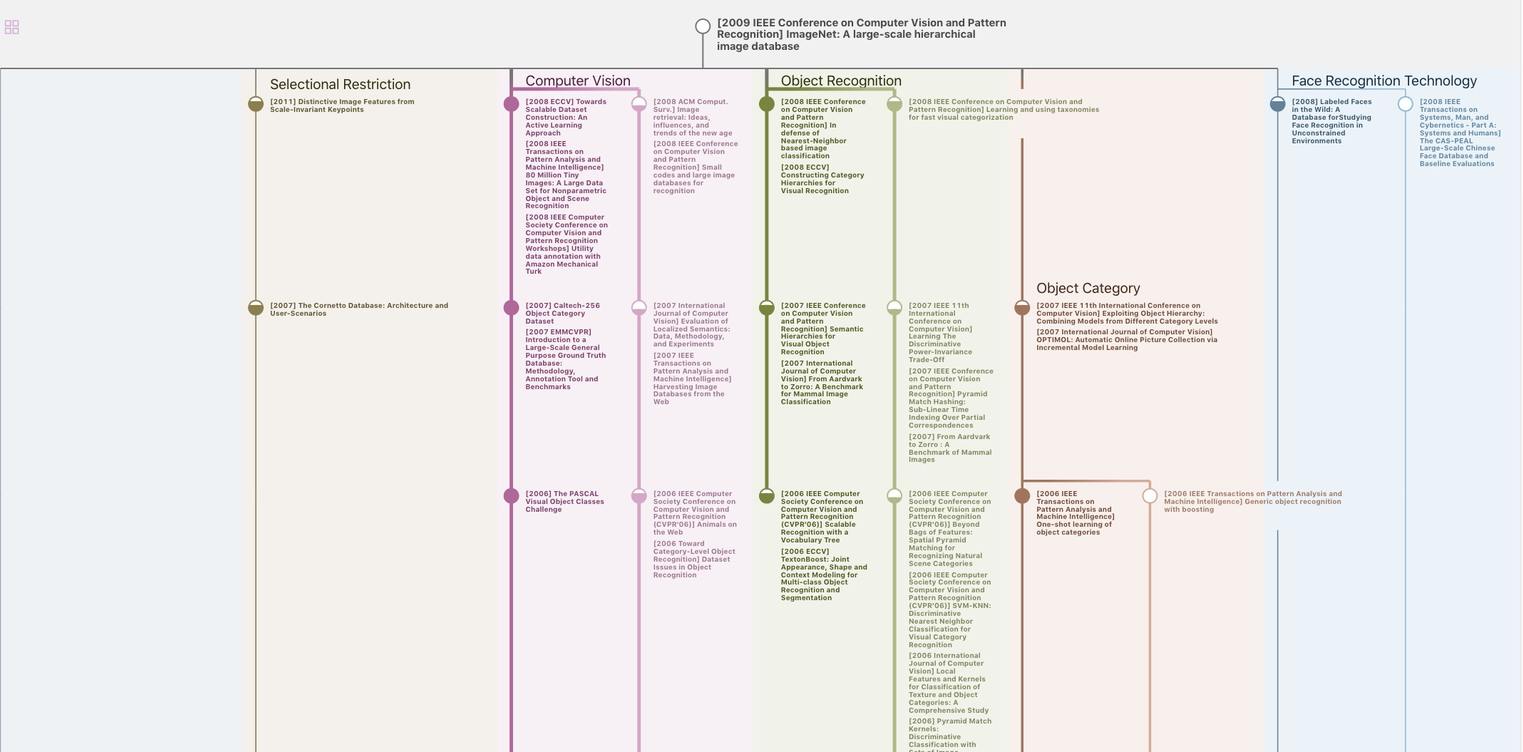
生成溯源树,研究论文发展脉络
Chat Paper
正在生成论文摘要