Towards Addressing Item Cold-Start Problem in Collaborative Filtering by Embedding Agglomerative Clustering and FP-Growth into the Recommendation System
Comput. Sci. Inf. Syst.(2023)
摘要
This paper introduces a frequent pattern mining framework for recommender systems (FPRS) -a novel approach to address the items' cold-start problem. This difficulty occurs when a new item hits the system, and properly handling such a situation is one of the key success factors of any deployment. The article proposes several strategies to combine collaborative and content-based filtering methods with frequent items mining and agglomerative clustering techniques to mitigate the cold-start problem in recommender systems. The experiments evaluated the developed methods against several quality metrics on three benchmark datasets. The conducted study confirmed usefulness of FPRS in providing apt outcomes even for cold items. The presented solution can be integrated with many different approaches and further extended to make up a complete and standalone RS.
更多查看译文
关键词
collaborative filtering,recommendation system,agglomerative clustering,cold-start,fp-growth
AI 理解论文
溯源树
样例
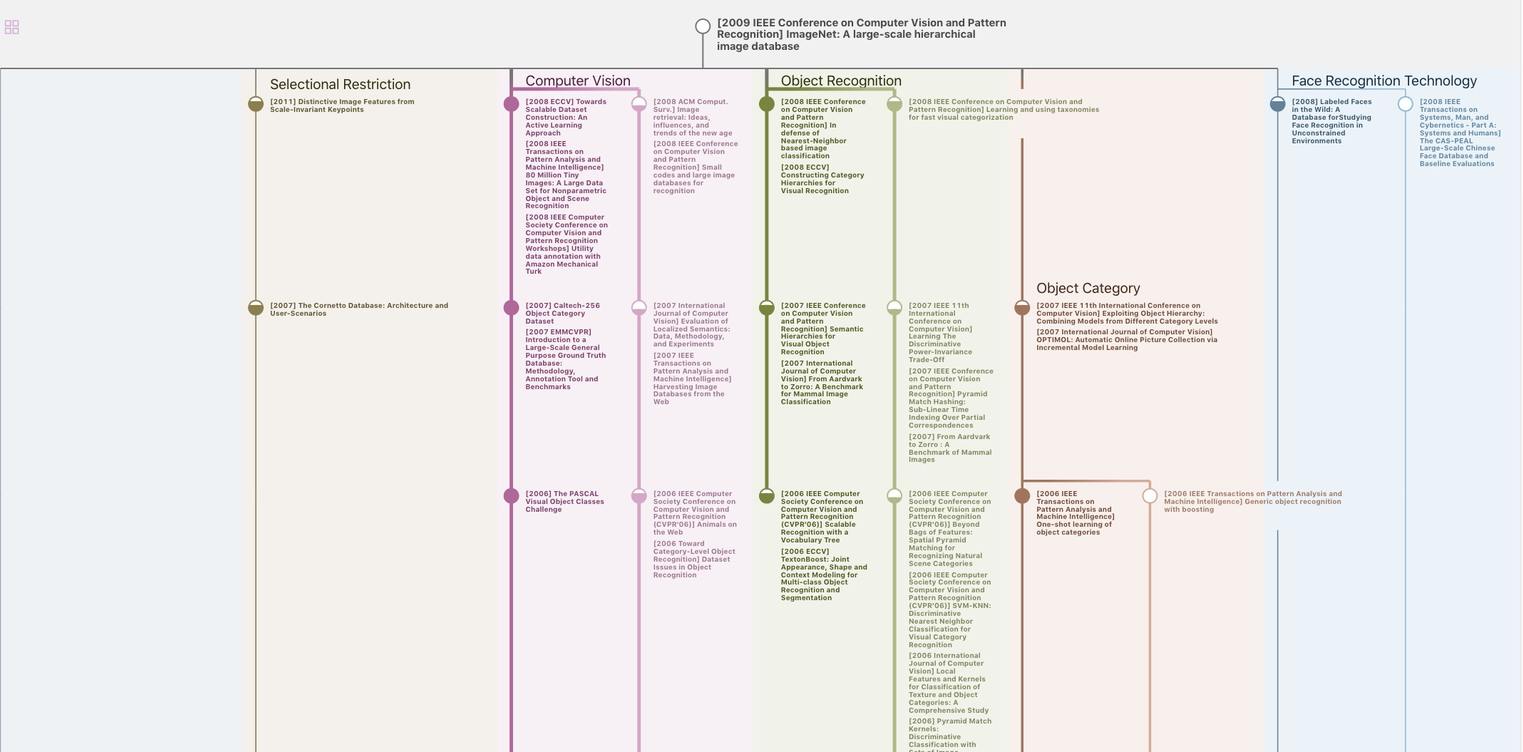
生成溯源树,研究论文发展脉络
Chat Paper
正在生成论文摘要