3DMAE: Joint SAR and Optical Representation Learning With Vertical Masking
IEEE GEOSCIENCE AND REMOTE SENSING LETTERS(2023)
摘要
The remote-sensing (RS) community has shown increasing interest in self-supervised learning for its ability to learn representations without labeled data. These representations can be easily adapted to downstream tasks through pretraining and fine-tuning. Recently, masked autoencoders (MAEs) have achieved better semantic representation by masking out a significant portion of the input image. However, the original design of MAEs for RGB natural images may not be optimal for RS images, which exhibit considerable variation between modalities like synthetic aperture radar (SAR) and optical. To address this, we propose a 3-D mask (3DM) that enhances feature extraction along the vertical dimension. After fine-tuning, our 3DMAE model outperforms state-of-the-art (SOTA) contrastive and MAE-based models on BigEarthNet-MM classification and significantly reduces the input data volume by at least 50% with the vertical mask, resulting in a more efficient model. Generalization experiments show a 5.9% F1-score improvement when applied to the SEN12MS dataset, which has diverse data distributions.
更多查看译文
关键词
Efficient correlation excavation,joint synthetic aperture radar (SAR) and optical modality,self-supervised learning,vertical mask
AI 理解论文
溯源树
样例
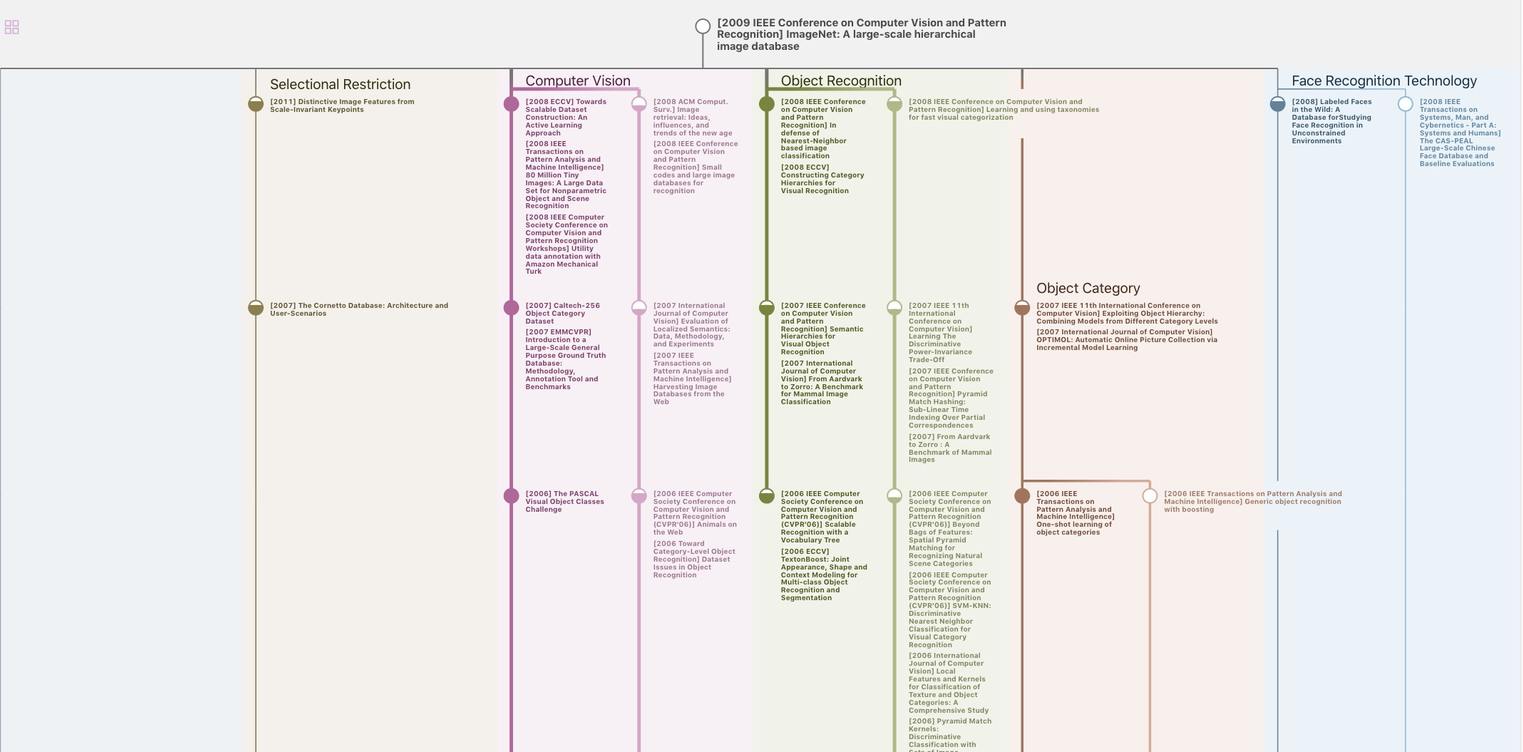
生成溯源树,研究论文发展脉络
Chat Paper
正在生成论文摘要