Subject-Level Membership Inference Attack via Data Augmentation and Model Discrepancy
IEEE Trans. Inf. Forensics Secur.(2023)
摘要
Federated learning (FL) models are vulnerable to membership inference attacks (MIAs), and the requirement of individual privacy motivates the protection of subjects where the individual data is distributed across multiple users in the cross-silo FL setting. In this paper, we propose a subject-level membership inference attack based on data augmentation and model discrepancy. It can effectively infer whether the data distribution of the target subject has been sampled and used for training by specific federated users, even if other users (also) may sample from the same subject and use it as part of their training set. Specifically, the adversary uses a generative adversarial network (GAN) to perform data augmentation on a small amount of priori federation-associated information known in advance. Subsequently, the adversary merges two different outputs from the global and tested user models using an optimal feature construction method. We simulate a controlled federation configuration and conduct extensive experiments on real datasets that include both image and categorical data. Results show that the area under the curve (AUC) is improved by 12.6% to 16.8% compared to the classical membership inference attack. This is at the expense of the test accuracy of the data augmented with GAN, which is at most 3.5% lower than the real test data. We also explore the degree of privacy leakage between overfitted models and well-generalized models in the cross-silo FL setting and conclude experimentally that the former is more likely to leak individual privacy with a subject-level degradation rate of up to 0.43. Finally, we present two possible defense mechanisms to attenuate this newly discovered privacy risk.
更多查看译文
关键词
Data models,Training,Data privacy,Privacy,Distributed databases,Degradation,Data augmentation,Federated learning,subject-level membership inference attacks,privacy degradation,generative adversarial networks
AI 理解论文
溯源树
样例
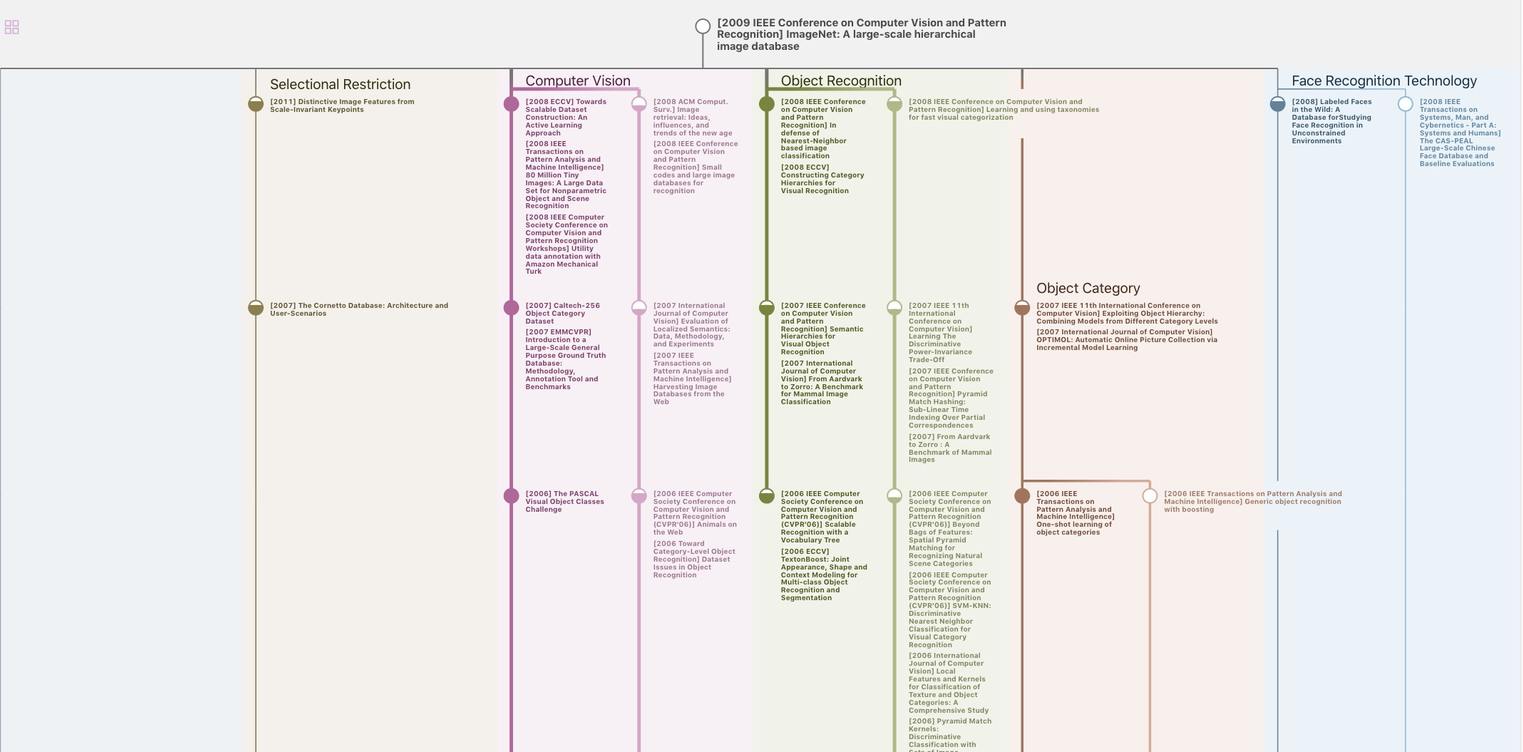
生成溯源树,研究论文发展脉络
Chat Paper
正在生成论文摘要