Characterizing Markov Random Fields and Coefficient of Variations as Measures of Spatial Distributions for Hyperspectral Image Classification.
IEEE Geosci. Remote. Sens. Lett.(2023)
摘要
Characterizing spatial information as reinforcement of spectral signatures can largely assist the performance in hyperspectral image (HSI) classification. Markov random fields (MRFs) are probabilistic image texture models and are capable of encoding contextual dependencies through characterizing local conditional probabilities. As a representative standardized measure of dispersion of image probability distributions, the coefficient of variation (CoV) can be a useful tool for characterizing spatial heterogeneity. Their parameter derivation processes also share strong compatibility with convolutional neural networks (CNNs) that specify spatial correlations in local neighborhoods. In this work, we propose an MRF and CoV-based spectral-spatial convolutional network (MRF-CoV-CNN) for HSI classification. MRF models and CoVs are characterized as measures of spatial distributions and further combined with spectral information. Then the proposed MRF-CoV-CNN takes the fused features as input and produces reliable classification results. Comprehensive experiments have been conducted on the Pavia University dataset and the Salinas dataset to evaluate the proposed method both visually and quantitatively.
更多查看译文
关键词
Coefficient of variation (CoV),convolutional neural networks (CNNs),hyperspectral image (HSI) classification,Markov random field (MRF),spatial distribution
AI 理解论文
溯源树
样例
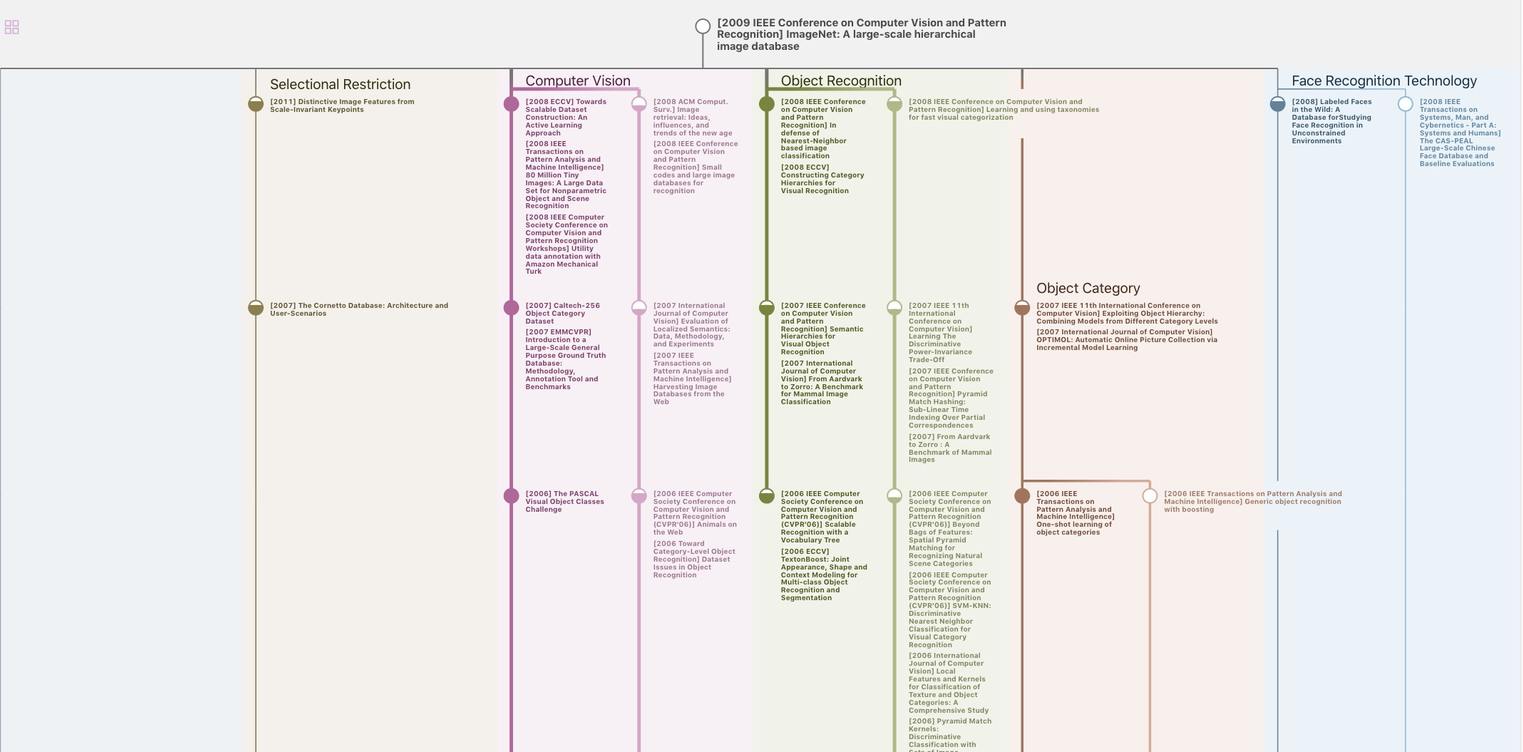
生成溯源树,研究论文发展脉络
Chat Paper
正在生成论文摘要