Sample Efficient Offline-to-Online Reinforcement Learning
IEEE TRANSACTIONS ON KNOWLEDGE AND DATA ENGINEERING(2024)
摘要
Offline reinforcement learning (RL) makes it possible to train the agents entirely from a previously collected dataset. However, constrained by the quality of the offline dataset, offline RL agents typically have limited performance and cannot be directly deployed. Thus, it is desirable to further finetune the pretrained offline RL agents via online interactions with the environment. Existing offline-to-online RL algorithms suffer from the low sample efficiency issue, due to two inherent challenges, i.e., exploration limitation and distribution shift. To this end, we propose a sample-efficient offline-to-online RL algorithm via Optimistic Exploration and Meta Adaptation (OEMA). Specifically, we first propose an optimistic exploration strategy according to the principle of optimism in the face of uncertainty. This allows agents to sufficiently explore the environment in a stable manner. Moreover, we propose a meta learning based adaptation method, which can reduce the distribution shift and accelerate the offline-to-online adaptation process. We empirically demonstrate that OEMA improves the sample efficiency on D4RL benchmark. Besides, we provide in-depth analyses to verify the effectiveness of both optimistic exploration and meta adaptation.
更多查看译文
关键词
Behavioral sciences,Perturbation methods,Uncertainty,Metalearning,Adaptation models,Q-learning,Faces,Meta learning,offline-to-online reinforcement learning,optimistic exploration,sample efficiency
AI 理解论文
溯源树
样例
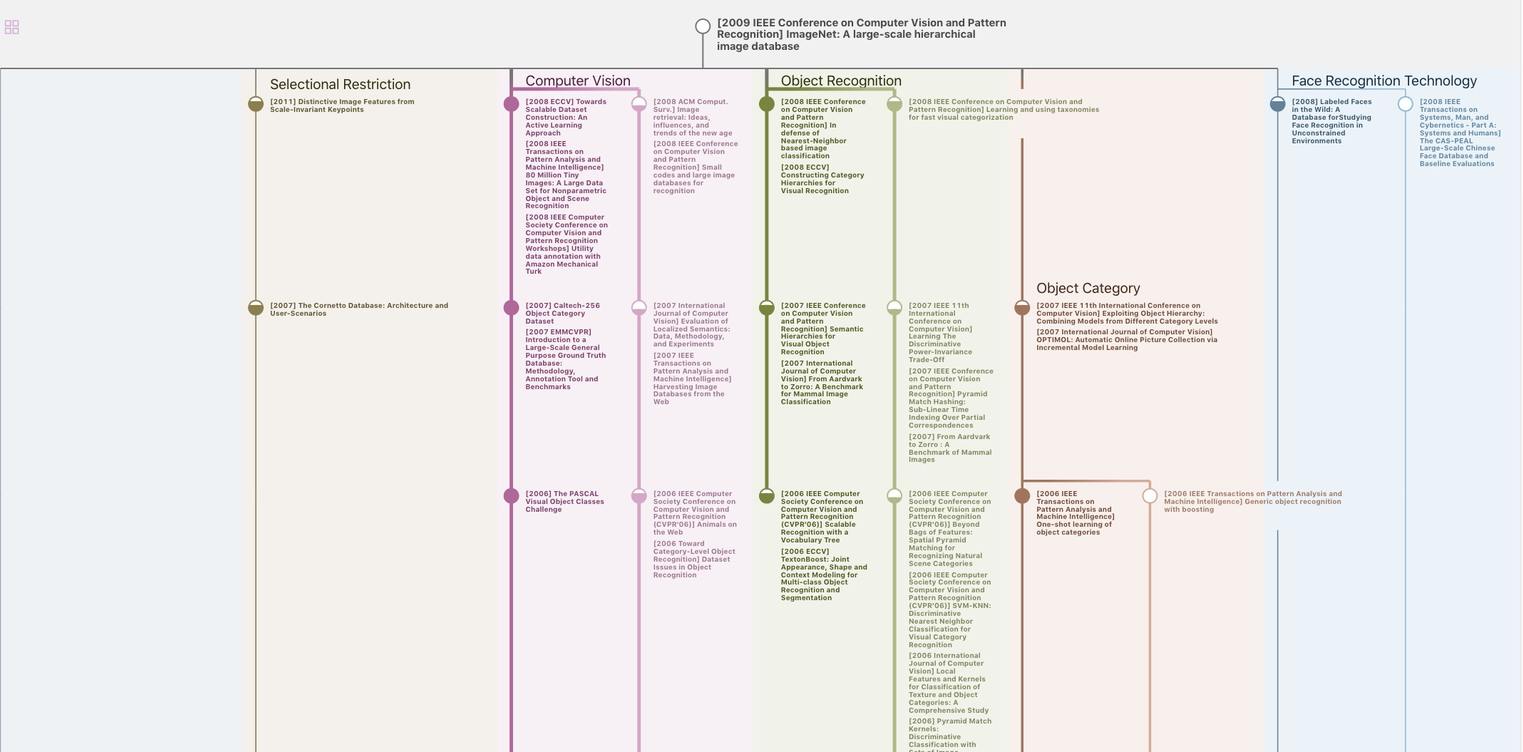
生成溯源树,研究论文发展脉络
Chat Paper
正在生成论文摘要