SimNorth: A Novel Contrastive Learning Approach for Clustering Prenatal Ultrasound Images
ASMUS@MICCAI(2023)
摘要
This paper describes SimNorth, an unsupervised learning approach for classifying non-standard fetal ultrasound images. SimNorth utilizes a deep feature learning model with a novel contrastive loss function to project images with similar characteristics closer together in an embedding space while pushing apart those with different image features. We then use non-linear dimensionality reduction via t-SNE and apply standard clustering algorithms such as k-means and dbscan in 2D embedding space to identify clusters containing similar fetal structures. We compare SimNorth to other unsupervised learning techniques (such as Autoencoders, MoCo, and SimCLR) and demonstrate its superior performance based on cluster purity measures.
更多查看译文
关键词
Unsupervised,clustering,Ultrasound,fetal,blind sweep
AI 理解论文
溯源树
样例
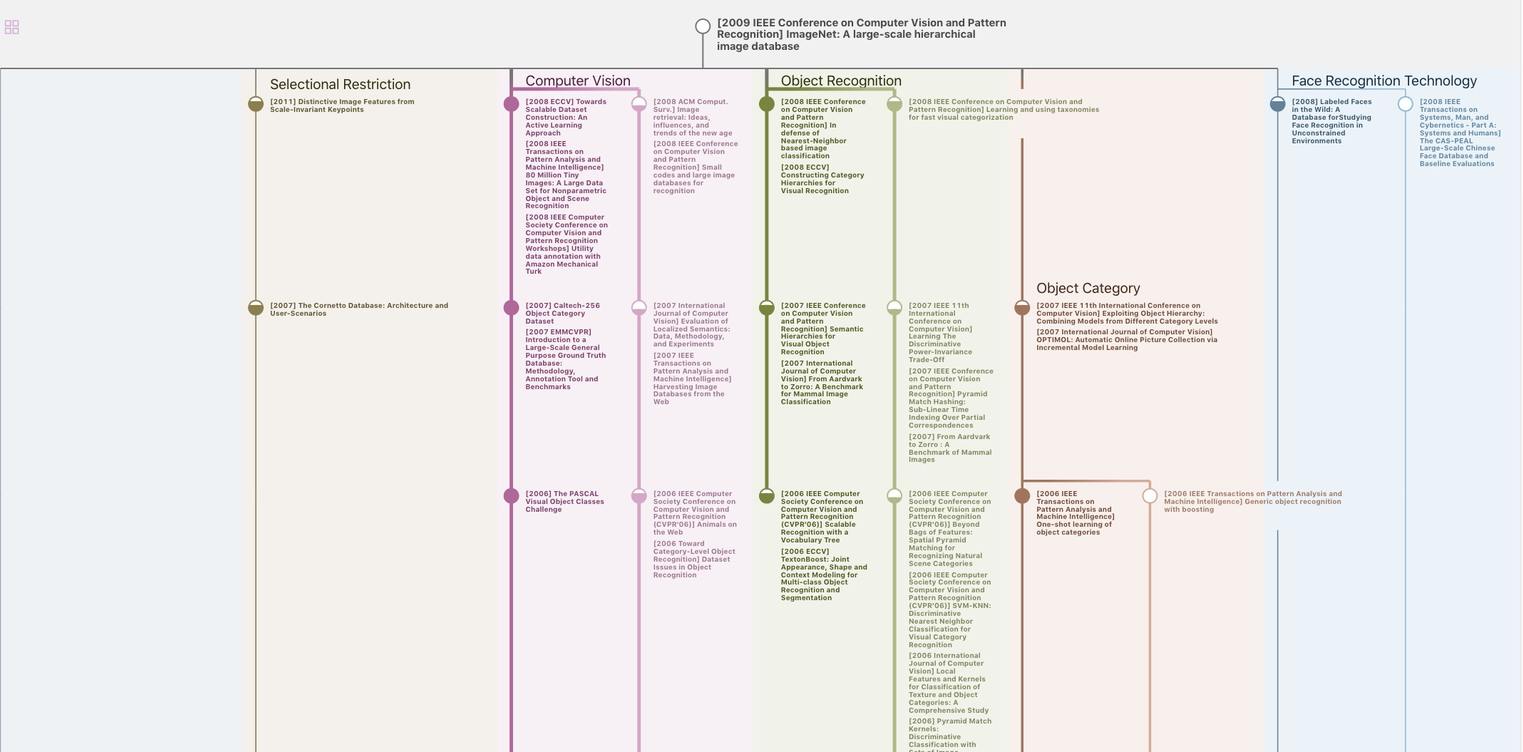
生成溯源树,研究论文发展脉络
Chat Paper
正在生成论文摘要