Virtual Contrast-Enhanced MRI Synthesis with High Model Generalizability Using Trusted Federated Learning (FL-TrustVCE): A Multi-institutional Study
CMMCA@MICCAI(2023)
摘要
In this study, we developed a trusted federated learning framework (FL-TrustVCE) for multi-institutional virtual contrast-enhanced MRI (VCE-MRI) synthesis. The FL-TrustVCE is featured with patient privacy preservation, data poisoning prevention, and multi-institutional data training. For FL-TrustVCE development, we retrospectively collected MRI data from 18 institutions, in total 438 patients were involved. For each patient, T1-weighted MRI, T2-weighted MRI, and corresponding CE-MRI were collected. T1-weighted and T2-weighted MRI were used as input to provide complementary information, and CE-MRI was used as the learning target. Data from 14 institutions were used for FL-TrustVCE model development and internal evaluation, while data from the other 4 institutions were used for external evaluation. The synthetic VCE-MRI was quantitatively evaluated using MAE and PSNR. The data poisoning prevention was visually assessed by reviewing the excluded images after training. Three single institutional models (separately trained with single institutional data), a joint model (jointly trained using multi-institutional data), and two popular federated learning frameworks (FedAvg and FedProx) were used for comparison. Quantitative results show that the proposed FL-TrustVCE outperformed all comparison methods in both internal and external testing datasets, yielding top-ranked average MAE and PSNR of 23.45 +/- 4.93 and 33.23 +/- 1.50 for internal datasets and 30.86 +/- 7.20 and 31.87 +/- 1.71 for external datasets. The poisoned data was successfully excluded. This study demonstrated that the proposed FL-TrustVCE is able to improve the VCE-MRI model generalizability and defend against data poisoning in the setting of multi-institutional model development.
更多查看译文
关键词
Data Poisoning,Federated Learning,Image Synthesis,MRI
AI 理解论文
溯源树
样例
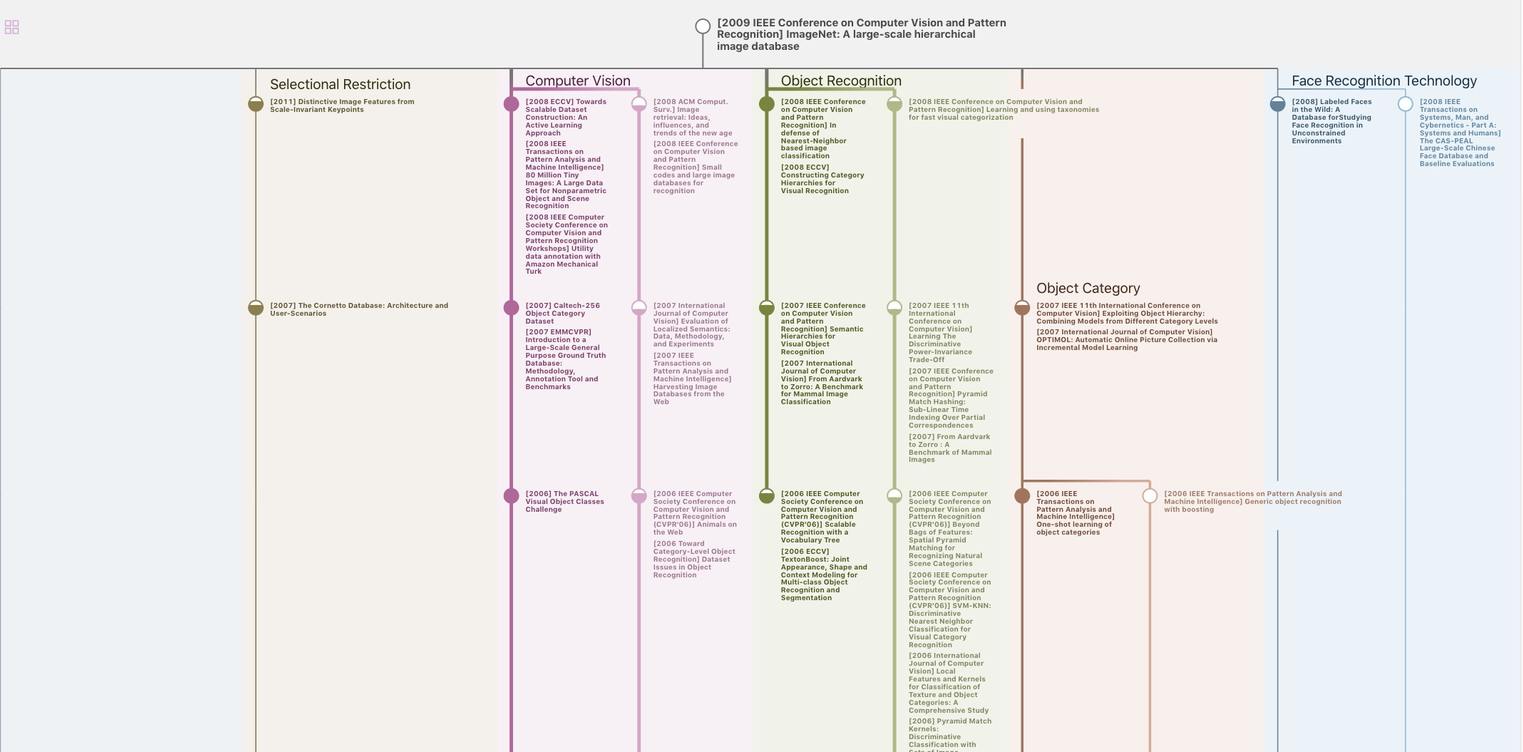
生成溯源树,研究论文发展脉络
Chat Paper
正在生成论文摘要