Continual Learning of a Time Series Model Using a Mixture of HMMs with Application to the IoT Fuel Sensor Verification
Annals of Computer Science and Information Systems(2023)
摘要
This paper presents an application of a mixture of Hidden Markov Models (HMMs) as a tool for verification of IoT fuel sensors. The IoT fuel sensors report the level of fuel in tanks of a petrol station, and are a key component for monitoring system reliability (billing), safety (fuel/oil leak detection) and security (theft prevention). We propose an algorithm for learning a mixture of HMMs based on a continual learning principle, i.e. it adapts the model while monitoring a sensor over time, signalling unexpected or anomalous sensor reports. We have tested the proposed approach on a real-life data of 15 fuel tanks being monitored with the FuelPrime system, where it has shown a very good performance (average area under ROC curve of 0.94) of detecting anomalies in the sensor data. Additionally we show that the proposed method can be used for trend monitoring and present qualitative analysis of the short and long term learning performance. The proposed method has promising performance score, the resulting model has a high degree of explainability, limited memory and computation requirements and can be easily generalized to other domains of sensor verification.
更多查看译文
AI 理解论文
溯源树
样例
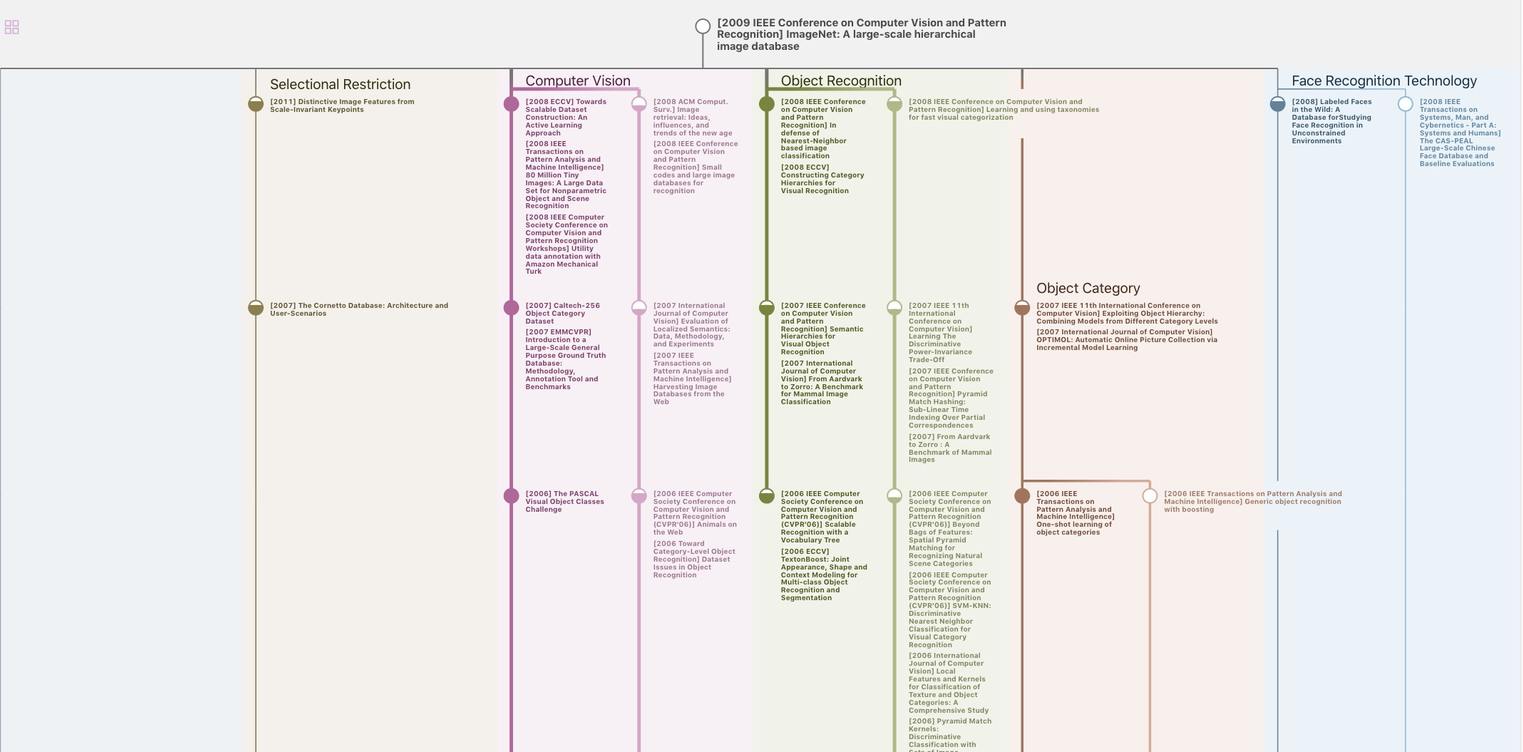
生成溯源树,研究论文发展脉络
Chat Paper
正在生成论文摘要