Inter-slice Consistency for Unpaired Low-Dose CT Denoising Using Boosted Contrastive Learning
MEDICAL IMAGE COMPUTING AND COMPUTER ASSISTED INTERVENTION, MICCAI 2023, PT I(2023)
摘要
The research field of low-dose computed tomography (LDCT) denoising is primarily dominated by supervised learning-based approaches, which necessitate the accurate registration of LDCT images and the corresponding NDCT images. However, since obtaining well-paired data is not always feasible in real clinical practice, unsupervised methods have become increasingly popular for LDCT denoising. One commonly used method is CycleGAN, but the training processing of CycleGAN is memory-intensive and mode collapse may occur. To address these limitations, we propose a novel unsupervised method based on boosted contrastive learning (BCL), which requires only a single generator. Furthermore, the constraints of computational power and memory capacity often force most existing approaches to focus solely on individual slices, leading to inconsistency in the results between consecutive slices. Our proposed BCL-based model integrates inter-slice features while maintaining the computational cost at an acceptable level comparable to most slice-based methods. Two modifications are introduced to the original contrastive learning method, including weight optimization for positive-negative pairs and imposing constraints on difference invariants. Experiments demonstrate that our method outperforms existing several state-of-the-art supervised and unsupervised methods in both qualitative and quantitative metrics.
更多查看译文
关键词
Low-dose computed tomography,unsupervised learning,image denoising,machine learning
AI 理解论文
溯源树
样例
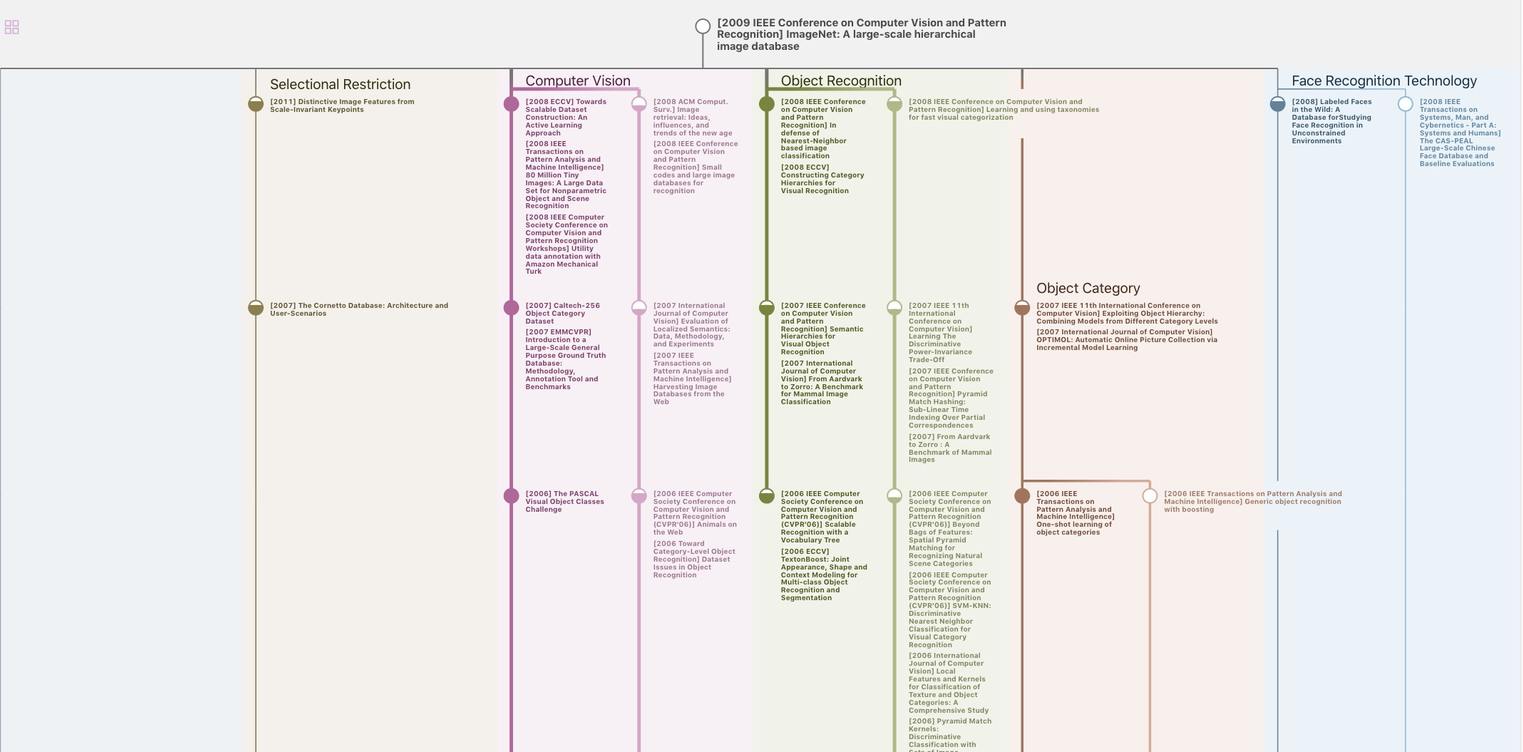
生成溯源树,研究论文发展脉络
Chat Paper
正在生成论文摘要