Deep Learning-Based Air Trapping Quantification Using Paired Inspiratory-Expiratory Ultra-low Dose CT
MICCAI (3)(2023)
摘要
Air trapping (AT) is a frequent finding in early cystic fibrosis (CF) lung disease detectable by imaging. The correct radiographic assessment of AT on paired inspiratory-expiratory computed tomography (CT) scans is laborious and prone to inter-reader variation. Conventional threshold-based methods for AT quantification are primarily designed for adults and less suitable for children. The administered radiation dose, in particular, plays an important role, especially for children. Low dose (LD) CT is considered established standard in pediatric lung CT imaging but also ultra-low dose (ULD) CT is technically feasible and requires comprehensive validation. We investigated a deep learning approach to quantify air trapping on ULDCT in comparison to LDCT and assessed structure-function relationships by cross-validation against multiple breath washout (MBW) lung function testing. A densely connected convolutional neural network (DenseNet) was trained on 2-D patches to segment AT. The mean threshold from radiographic assessments, performed by two trained radiologists, was used as ground truth. A grid search was conducted to find the best parameter configuration. Quantitative AT (QAT), defined as the percentage of AT in the lungs detected by our DenseNet models, correlated strongly between LD and ULD. Structure-function relationships were maintained. The best model achieved a patch-based DICE coefficient of 0.82 evaluated on the test set. AT percentages correlated strongly with MBW results (LD: R = 0.76, p < 0.001; ULD: R = 0.78, p < 0.001). A strong correlation between LD and ULD (R = 0.96, p < 0.001) and small ULD-LD differences (mean difference - 1.04 +/- 3.25%) were observed.
更多查看译文
关键词
Deep Learning,Air Trapping Quantification,Cystic Fibrosis
AI 理解论文
溯源树
样例
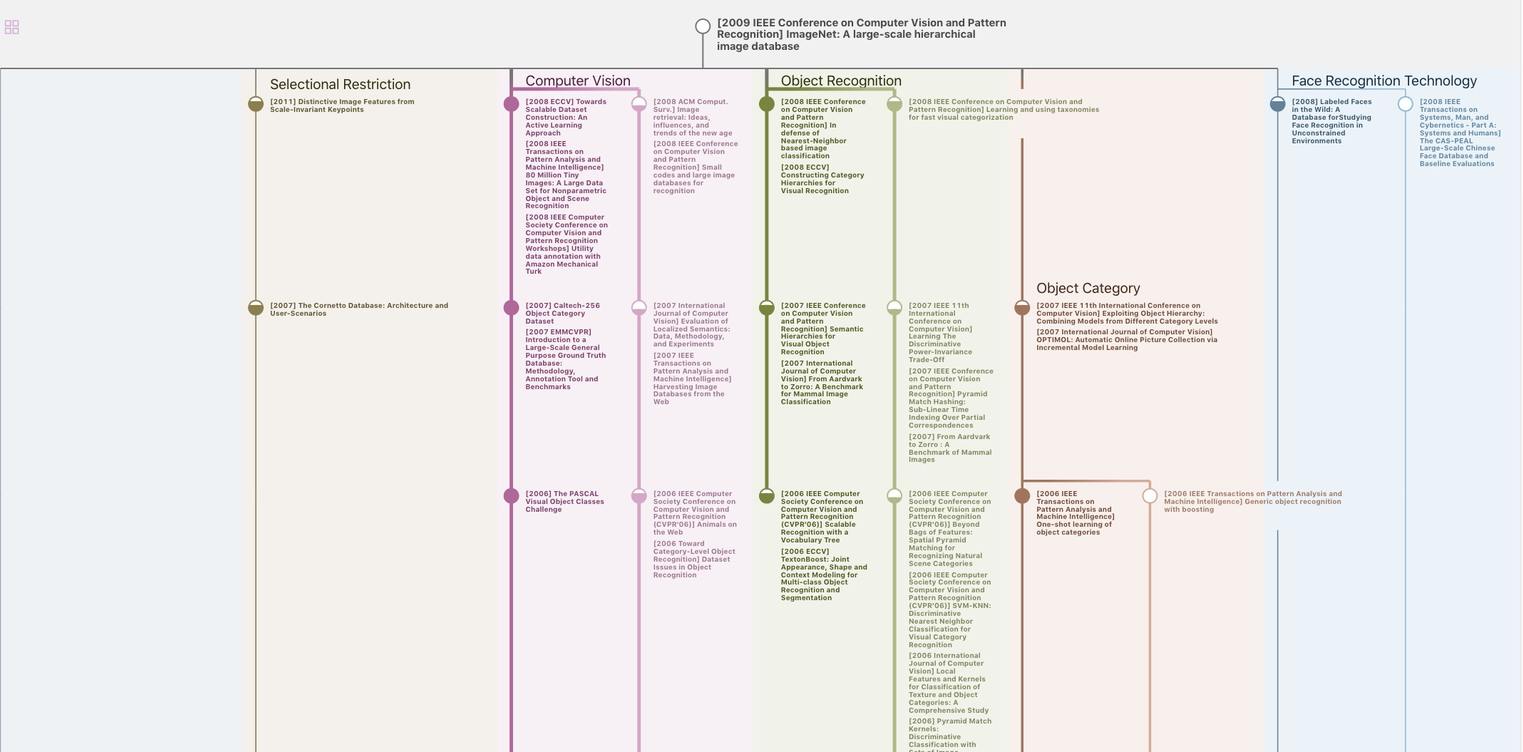
生成溯源树,研究论文发展脉络
Chat Paper
正在生成论文摘要