Evidence Reconciled Neural Network for Out-of-Distribution Detection in Medical Images
MEDICAL IMAGE COMPUTING AND COMPUTER ASSISTED INTERVENTION, MICCAI 2023, PT III(2023)
摘要
Near Out-of-Distribution (OOD) detection is a crucial issue in medical applications, as misdiagnosis caused by the presence of rare diseases inevitablely poses a significant risk. Recently, several deep learning-based methods for OOD detection with uncertainty estimation, such as the Evidential Deep Learning (EDL) and its variants, have shown remarkable performance in identifying outliers that significantly differ from training samples. Nevertheless, few studies focus on the great challenge of near OOD detection problem, which involves detecting outliers that are close to the training distribution, as commonly encountered in medical image application. To address this limitation and reduce the risk of misdiagnosis, we propose an Evidence Reconciled Neural Network (ERNN). Concretely, we reform the evidence representation obtained from the evidential head with the proposed Evidential Reconcile Block (ERB), which restricts the decision boundary of the model and further improves the performance in near OOD detection. Compared with the state-of-the-art uncertainty-based methods for OOD detection, our method reduces the evidential error and enhances the capability of near OOD detection in medical applications. The experiments on both the ISIC2019 dataset and an in-house pancreas tumor dataset validate the robustness and effectiveness of our approach. Code for ERNN has been released at https://github.com/KellaDoe/ERNN.
更多查看译文
关键词
Near out-of-distribution detection,Uncertainty estimation,Reconciled evidence representation,Evidential neural network
AI 理解论文
溯源树
样例
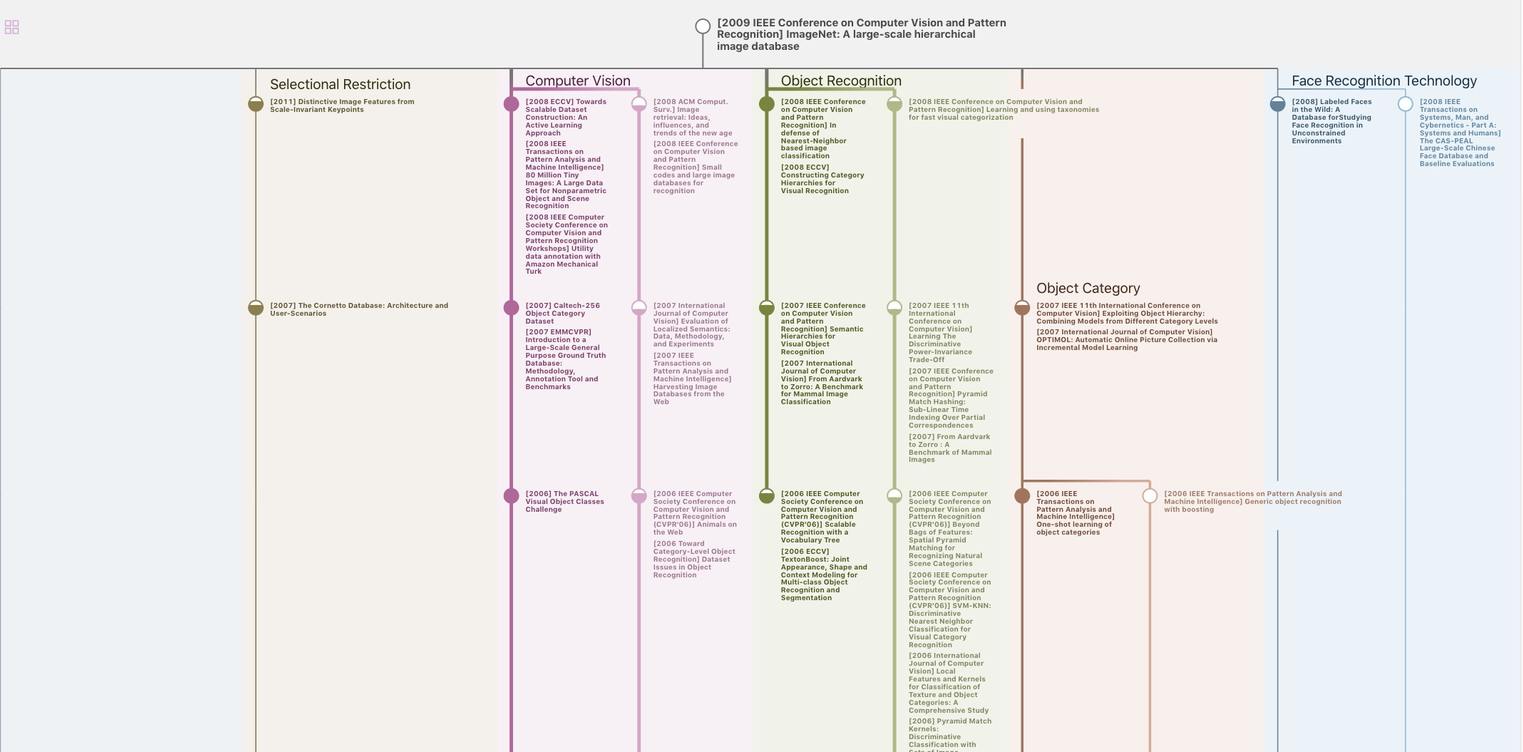
生成溯源树,研究论文发展脉络
Chat Paper
正在生成论文摘要